11111111
This commit is contained in:
commit
f322af6014
|
@ -0,0 +1,201 @@
|
|||
Apache License
|
||||
Version 2.0, January 2004
|
||||
http://www.apache.org/licenses/
|
||||
|
||||
TERMS AND CONDITIONS FOR USE, REPRODUCTION, AND DISTRIBUTION
|
||||
|
||||
1. Definitions.
|
||||
|
||||
"License" shall mean the terms and conditions for use, reproduction,
|
||||
and distribution as defined by Sections 1 through 9 of this document.
|
||||
|
||||
"Licensor" shall mean the copyright owner or entity authorized by
|
||||
the copyright owner that is granting the License.
|
||||
|
||||
"Legal Entity" shall mean the union of the acting entity and all
|
||||
other entities that control, are controlled by, or are under common
|
||||
control with that entity. For the purposes of this definition,
|
||||
"control" means (i) the power, direct or indirect, to cause the
|
||||
direction or management of such entity, whether by contract or
|
||||
otherwise, or (ii) ownership of fifty percent (50%) or more of the
|
||||
outstanding shares, or (iii) beneficial ownership of such entity.
|
||||
|
||||
"You" (or "Your") shall mean an individual or Legal Entity
|
||||
exercising permissions granted by this License.
|
||||
|
||||
"Source" form shall mean the preferred form for making modifications,
|
||||
including but not limited to software source code, documentation
|
||||
source, and configuration files.
|
||||
|
||||
"Object" form shall mean any form resulting from mechanical
|
||||
transformation or translation of a Source form, including but
|
||||
not limited to compiled object code, generated documentation,
|
||||
and conversions to other media types.
|
||||
|
||||
"Work" shall mean the work of authorship, whether in Source or
|
||||
Object form, made available under the License, as indicated by a
|
||||
copyright notice that is included in or attached to the work
|
||||
(an example is provided in the Appendix below).
|
||||
|
||||
"Derivative Works" shall mean any work, whether in Source or Object
|
||||
form, that is based on (or derived from) the Work and for which the
|
||||
editorial revisions, annotations, elaborations, or other modifications
|
||||
represent, as a whole, an original work of authorship. For the purposes
|
||||
of this License, Derivative Works shall not include works that remain
|
||||
separable from, or merely link (or bind by name) to the interfaces of,
|
||||
the Work and Derivative Works thereof.
|
||||
|
||||
"Contribution" shall mean any work of authorship, including
|
||||
the original version of the Work and any modifications or additions
|
||||
to that Work or Derivative Works thereof, that is intentionally
|
||||
submitted to Licensor for inclusion in the Work by the copyright owner
|
||||
or by an individual or Legal Entity authorized to submit on behalf of
|
||||
the copyright owner. For the purposes of this definition, "submitted"
|
||||
means any form of electronic, verbal, or written communication sent
|
||||
to the Licensor or its representatives, including but not limited to
|
||||
communication on electronic mailing lists, source code control systems,
|
||||
and issue tracking systems that are managed by, or on behalf of, the
|
||||
Licensor for the purpose of discussing and improving the Work, but
|
||||
excluding communication that is conspicuously marked or otherwise
|
||||
designated in writing by the copyright owner as "Not a Contribution."
|
||||
|
||||
"Contributor" shall mean Licensor and any individual or Legal Entity
|
||||
on behalf of whom a Contribution has been received by Licensor and
|
||||
subsequently incorporated within the Work.
|
||||
|
||||
2. Grant of Copyright License. Subject to the terms and conditions of
|
||||
this License, each Contributor hereby grants to You a perpetual,
|
||||
worldwide, non-exclusive, no-charge, royalty-free, irrevocable
|
||||
copyright license to reproduce, prepare Derivative Works of,
|
||||
publicly display, publicly perform, sublicense, and distribute the
|
||||
Work and such Derivative Works in Source or Object form.
|
||||
|
||||
3. Grant of Patent License. Subject to the terms and conditions of
|
||||
this License, each Contributor hereby grants to You a perpetual,
|
||||
worldwide, non-exclusive, no-charge, royalty-free, irrevocable
|
||||
(except as stated in this section) patent license to make, have made,
|
||||
use, offer to sell, sell, import, and otherwise transfer the Work,
|
||||
where such license applies only to those patent claims licensable
|
||||
by such Contributor that are necessarily infringed by their
|
||||
Contribution(s) alone or by combination of their Contribution(s)
|
||||
with the Work to which such Contribution(s) was submitted. If You
|
||||
institute patent litigation against any entity (including a
|
||||
cross-claim or counterclaim in a lawsuit) alleging that the Work
|
||||
or a Contribution incorporated within the Work constitutes direct
|
||||
or contributory patent infringement, then any patent licenses
|
||||
granted to You under this License for that Work shall terminate
|
||||
as of the date such litigation is filed.
|
||||
|
||||
4. Redistribution. You may reproduce and distribute copies of the
|
||||
Work or Derivative Works thereof in any medium, with or without
|
||||
modifications, and in Source or Object form, provided that You
|
||||
meet the following conditions:
|
||||
|
||||
(a) You must give any other recipients of the Work or
|
||||
Derivative Works a copy of this License; and
|
||||
|
||||
(b) You must cause any modified files to carry prominent notices
|
||||
stating that You changed the files; and
|
||||
|
||||
(c) You must retain, in the Source form of any Derivative Works
|
||||
that You distribute, all copyright, patent, trademark, and
|
||||
attribution notices from the Source form of the Work,
|
||||
excluding those notices that do not pertain to any part of
|
||||
the Derivative Works; and
|
||||
|
||||
(d) If the Work includes a "NOTICE" text file as part of its
|
||||
distribution, then any Derivative Works that You distribute must
|
||||
include a readable copy of the attribution notices contained
|
||||
within such NOTICE file, excluding those notices that do not
|
||||
pertain to any part of the Derivative Works, in at least one
|
||||
of the following places: within a NOTICE text file distributed
|
||||
as part of the Derivative Works; within the Source form or
|
||||
documentation, if provided along with the Derivative Works; or,
|
||||
within a display generated by the Derivative Works, if and
|
||||
wherever such third-party notices normally appear. The contents
|
||||
of the NOTICE file are for informational purposes only and
|
||||
do not modify the License. You may add Your own attribution
|
||||
notices within Derivative Works that You distribute, alongside
|
||||
or as an addendum to the NOTICE text from the Work, provided
|
||||
that such additional attribution notices cannot be construed
|
||||
as modifying the License.
|
||||
|
||||
You may add Your own copyright statement to Your modifications and
|
||||
may provide additional or different license terms and conditions
|
||||
for use, reproduction, or distribution of Your modifications, or
|
||||
for any such Derivative Works as a whole, provided Your use,
|
||||
reproduction, and distribution of the Work otherwise complies with
|
||||
the conditions stated in this License.
|
||||
|
||||
5. Submission of Contributions. Unless You explicitly state otherwise,
|
||||
any Contribution intentionally submitted for inclusion in the Work
|
||||
by You to the Licensor shall be under the terms and conditions of
|
||||
this License, without any additional terms or conditions.
|
||||
Notwithstanding the above, nothing herein shall supersede or modify
|
||||
the terms of any separate license agreement you may have executed
|
||||
with Licensor regarding such Contributions.
|
||||
|
||||
6. Trademarks. This License does not grant permission to use the trade
|
||||
names, trademarks, service marks, or product names of the Licensor,
|
||||
except as required for reasonable and customary use in describing the
|
||||
origin of the Work and reproducing the content of the NOTICE file.
|
||||
|
||||
7. Disclaimer of Warranty. Unless required by applicable law or
|
||||
agreed to in writing, Licensor provides the Work (and each
|
||||
Contributor provides its Contributions) on an "AS IS" BASIS,
|
||||
WITHOUT WARRANTIES OR CONDITIONS OF ANY KIND, either express or
|
||||
implied, including, without limitation, any warranties or conditions
|
||||
of TITLE, NON-INFRINGEMENT, MERCHANTABILITY, or FITNESS FOR A
|
||||
PARTICULAR PURPOSE. You are solely responsible for determining the
|
||||
appropriateness of using or redistributing the Work and assume any
|
||||
risks associated with Your exercise of permissions under this License.
|
||||
|
||||
8. Limitation of Liability. In no event and under no legal theory,
|
||||
whether in tort (including negligence), contract, or otherwise,
|
||||
unless required by applicable law (such as deliberate and grossly
|
||||
negligent acts) or agreed to in writing, shall any Contributor be
|
||||
liable to You for damages, including any direct, indirect, special,
|
||||
incidental, or consequential damages of any character arising as a
|
||||
result of this License or out of the use or inability to use the
|
||||
Work (including but not limited to damages for loss of goodwill,
|
||||
work stoppage, computer failure or malfunction, or any and all
|
||||
other commercial damages or losses), even if such Contributor
|
||||
has been advised of the possibility of such damages.
|
||||
|
||||
9. Accepting Warranty or Additional Liability. While redistributing
|
||||
the Work or Derivative Works thereof, You may choose to offer,
|
||||
and charge a fee for, acceptance of support, warranty, indemnity,
|
||||
or other liability obligations and/or rights consistent with this
|
||||
License. However, in accepting such obligations, You may act only
|
||||
on Your own behalf and on Your sole responsibility, not on behalf
|
||||
of any other Contributor, and only if You agree to indemnify,
|
||||
defend, and hold each Contributor harmless for any liability
|
||||
incurred by, or claims asserted against, such Contributor by reason
|
||||
of your accepting any such warranty or additional liability.
|
||||
|
||||
END OF TERMS AND CONDITIONS
|
||||
|
||||
APPENDIX: How to apply the Apache License to your work.
|
||||
|
||||
To apply the Apache License to your work, attach the following
|
||||
boilerplate notice, with the fields enclosed by brackets "[]"
|
||||
replaced with your own identifying information. (Don't include
|
||||
the brackets!) The text should be enclosed in the appropriate
|
||||
comment syntax for the file format. We also recommend that a
|
||||
file or class name and description of purpose be included on the
|
||||
same "printed page" as the copyright notice for easier
|
||||
identification within third-party archives.
|
||||
|
||||
Copyright [yyyy] [name of copyright owner]
|
||||
|
||||
Licensed under the Apache License, Version 2.0 (the "License");
|
||||
you may not use this file except in compliance with the License.
|
||||
You may obtain a copy of the License at
|
||||
|
||||
http://www.apache.org/licenses/LICENSE-2.0
|
||||
|
||||
Unless required by applicable law or agreed to in writing, software
|
||||
distributed under the License is distributed on an "AS IS" BASIS,
|
||||
WITHOUT WARRANTIES OR CONDITIONS OF ANY KIND, either express or implied.
|
||||
See the License for the specific language governing permissions and
|
||||
limitations under the License.
|
|
@ -0,0 +1,691 @@
|
|||
<div align="center">
|
||||
<img src='docs/img/flgo_icon.png' width="200"/>
|
||||
<h1>FLGo: A Lightning Framework for Federated Learning</h1>
|
||||
|
||||
<!-- [](https://pypi.org/project/flgo/)
|
||||
[](https://pypi.org/project/flgo/) -->
|
||||
[](https://pypi.org/project/flgo/)
|
||||
[](https://flgo-xmu.github.io/)
|
||||
[](https://github.com/WwZzz/easyFL/blob/FLGo/LICENSE)
|
||||
|
||||
|
||||
</div>
|
||||
|
||||
|
||||
<!-- ## Major Feature -->
|
||||
|
||||
[//]: # (## Table of Contents)
|
||||
|
||||
[//]: # (- [Introduction](#Introduction))
|
||||
|
||||
[//]: # (- [QuickStart](#Quick Start with 3 lines))
|
||||
|
||||
[//]: # (- [Architecture](#Architecture))
|
||||
|
||||
[//]: # (- [Citation](#Citation))
|
||||
|
||||
[//]: # (- [Contacts](#Contacts))
|
||||
|
||||
[//]: # (- [References](#References))
|
||||
|
||||
[//]: # (- )
|
||||
# Introduction
|
||||
FLGo is a library to conduct experiments about Federated Learning (FL). It is strong and reusable for research on FL, providing comprehensive easy-to-use modules to hold out for those who want to do various federated learning experiments.
|
||||
|
||||
## Installation
|
||||
* Install FLGo through pip. It's recommended to install pytorch by yourself before installing this library.
|
||||
```sh
|
||||
pip install flgo --upgrade
|
||||
```
|
||||
* Install FLGo through git
|
||||
```sh
|
||||
git clone https://github.com/WwZzz/easyFL.git
|
||||
```
|
||||
## Join Us :smiley:
|
||||
Welcome to our FLGo's WeChat group/QQ Group for more technical discussion.
|
||||
|
||||
<center>
|
||||
<!-- <img src="https://github.com/user-attachments/assets/230247bc-8fce-4821-901b-d0e22ca360fd" width=180/> -->
|
||||
|
||||
<img src="https://github.com/user-attachments/assets/39070ba7-4752-46ec-b8b4-3d5591992595" width=180/>
|
||||
</center>
|
||||
|
||||
Group Number: 838298386
|
||||
|
||||
Tutorials in Chinese can be found [here](https://www.zhihu.com/column/c_1618319253936984064)
|
||||
# News
|
||||
**[2024.9.20]** We present a comprehensive benchmark gallery [here](https://github.com/WwZzz/FLGo-Bench)
|
||||
|
||||
**[2024.8.01]** Improving efficiency by sharing datasets across multiple processes within each task in the shared memory
|
||||
|
||||
# Quick Start with 3 lines :zap:
|
||||
```python
|
||||
import flgo
|
||||
import flgo.benchmark.mnist_classification as mnist
|
||||
import flgo.benchmark.partition as fbp
|
||||
import flgo.algorithm.fedavg as fedavg
|
||||
|
||||
# Line 1: Create a typical federated learning task
|
||||
flgo.gen_task_by_(mnist, fbp.IIDPartitioner(num_clients=100), './my_task')
|
||||
|
||||
# Line 2: Running FedAvg on this task
|
||||
fedavg_runner = flgo.init('./my_task', fedavg, {'gpu': [0,], 'num_rounds':20, 'num_epochs': 1})
|
||||
|
||||
# Line 3: Start Training
|
||||
fedavg_runner.run()
|
||||
```
|
||||
We take a classical federated dataset, Federated MNIST, as the example. The MNIST dataset is splitted into 100 parts identically and independently.
|
||||
|
||||
Line 1 creates the federated dataset as `./my_task` and visualizes it in `./my_task/res.png`
|
||||

|
||||
|
||||
Lines 2 and 3 start the training procedure and outputs information to the console
|
||||
```
|
||||
2024-04-15 02:30:43,763 fflow.py init [line:642] INFO PROCESS ID: 552206
|
||||
2024-04-15 02:30:43,763 fflow.py init [line:643] INFO Initializing devices: cuda:0 will be used for this running.
|
||||
2024-04-15 02:30:43,763 fflow.py init [line:646] INFO BENCHMARK: flgo.benchmark.mnist_classification
|
||||
2024-04-15 02:30:43,763 fflow.py init [line:647] INFO TASK: ./my_task
|
||||
2024-04-15 02:30:43,763 fflow.py init [line:648] INFO MODEL: flgo.benchmark.mnist_classification.model.cnn
|
||||
2024-04-15 02:30:43,763 fflow.py init [line:649] INFO ALGORITHM: fedavg
|
||||
2024-04-15 02:30:43,774 fflow.py init [line:688] INFO SCENE: horizontal FL with 1 <class 'flgo.algorithm.fedbase.BasicServer'>, 100 <class 'flgo.algorithm.fedbase.BasicClient'>
|
||||
2024-04-15 02:30:47,851 fflow.py init [line:705] INFO SIMULATOR: <class 'flgo.simulator.default_simulator.Simulator'>
|
||||
2024-04-15 02:30:47,853 fflow.py init [line:718] INFO Ready to start.
|
||||
...
|
||||
2024-04-15 02:30:52,466 fedbase.py run [line:253] INFO --------------Round 1--------------
|
||||
2024-04-15 02:30:52,466 simple_logger.py log_once [line:14] INFO Current_time:1
|
||||
2024-04-15 02:30:54,402 simple_logger.py log_once [line:28] INFO test_accuracy 0.6534
|
||||
2024-04-15 02:30:54,402 simple_logger.py log_once [line:28] INFO test_loss 1.5835
|
||||
...
|
||||
```
|
||||
* **Show Training Result (optional)**
|
||||
```python
|
||||
import flgo.experiment.analyzer as fea
|
||||
# Create the analysis plan
|
||||
analysis_plan = {
|
||||
'Selector':{'task': './my_task', 'header':['fedavg',], },
|
||||
'Painter':{'Curve':[{'args':{'x':'communication_round', 'y':'val_loss'}}]},
|
||||
}
|
||||
|
||||
fea.show(analysis_plan)
|
||||
```
|
||||
Each training result will be saved as a record under `./my_task/record`. We can use the built-in analyzer to read and show it.
|
||||
|
||||

|
||||
|
||||
# Why Using FLGo? :hammer_and_wrench:
|
||||
## Simulate Real-World System Heterogeneity :iphone:
|
||||
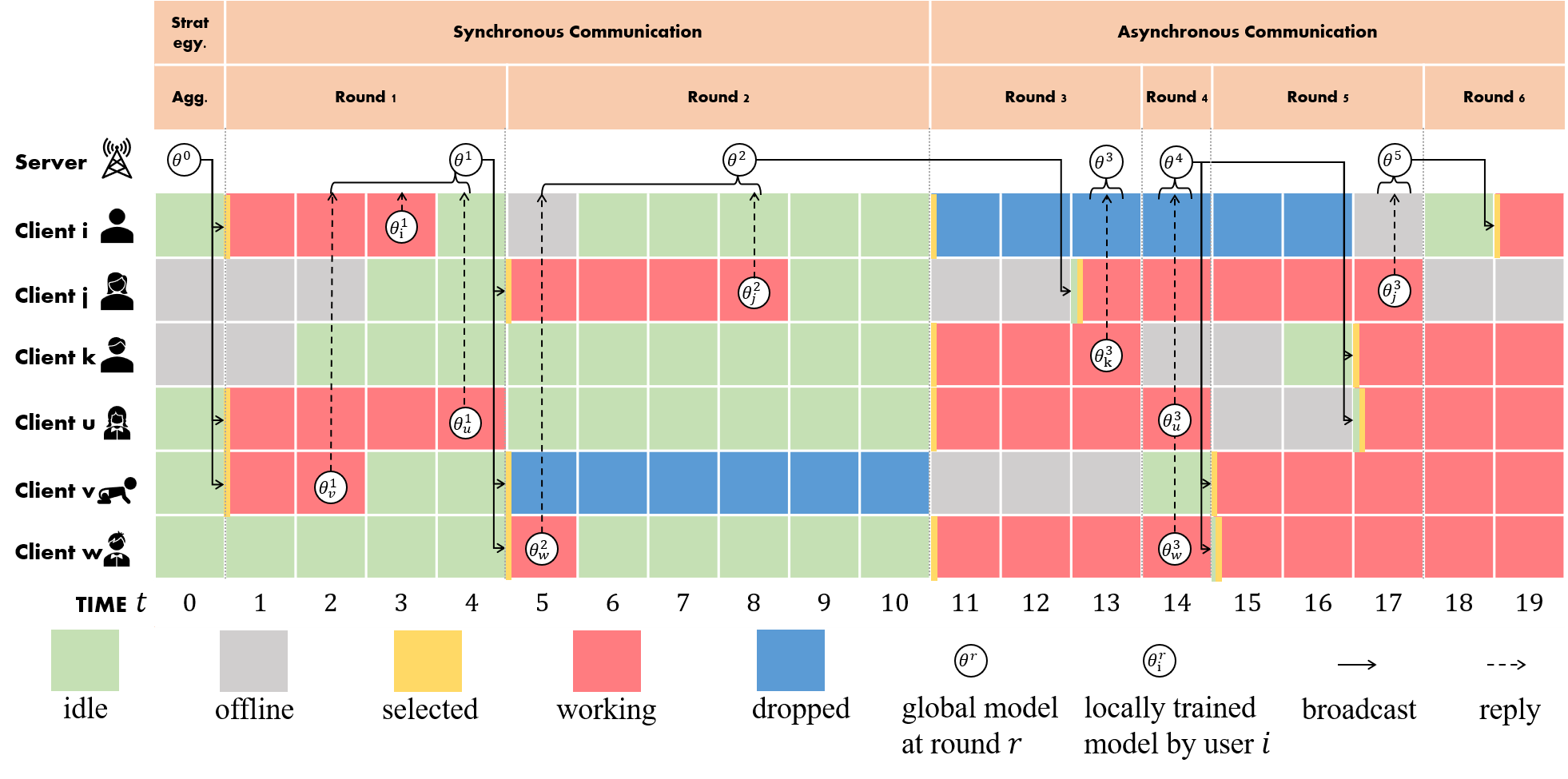
|
||||
Our FLGo supports running different algorithms in virtual environments like real-world. For example, clients in practice may
|
||||
* *be sometime inavailable*,
|
||||
* *response to the server very slow*,
|
||||
* *accidiently lose connection*,
|
||||
* *upload incomplete model updates*,
|
||||
* ...
|
||||
|
||||
All of these behavior can be easily realized by integrating a simple `Simulator` to the runner like
|
||||
```python
|
||||
import flgo
|
||||
from flgo.simulator import ExampleSimulator
|
||||
import flgo.algorithm.fedavg as fedavg
|
||||
|
||||
fedavg_runner = flgo.init('./my_task', fedavg, {'gpu': [0,]}, simulator=ExampleSimulator)
|
||||
fedavg_runner.run()
|
||||
```
|
||||
|
||||
`Simulator` is fully customizable and can fairly reflect the impact of system heterogeneity on different algorithms. Please refer to [Paper](https://arxiv.org/abs/2306.12079) or [Tutorial](https://flgo-xmu.github.io/Tutorials/4_Simulator_Customization/) for more details.
|
||||
## Comprehensive Benchmarks :family_woman_woman_boy_boy:
|
||||
FLGo provides more than 50 benchmarks across different data types, different communication topology,...
|
||||
<table>
|
||||
<tr>
|
||||
<td>
|
||||
<td>Task
|
||||
<td>Scenario
|
||||
<td>Datasets
|
||||
<td>
|
||||
</tr>
|
||||
<tr>
|
||||
<td rowspan=3>CV
|
||||
<td>Classification
|
||||
<td>Horizontal & Vertical
|
||||
<td>CIFAR10\100, MNIST, FashionMNIST,FEMNIST, EMNIST, SVHN
|
||||
<td>
|
||||
</tr>
|
||||
<tr>
|
||||
<td>Detection
|
||||
<td>Horizontal
|
||||
<td>Coco, VOC
|
||||
<td>
|
||||
</tr>
|
||||
<tr>
|
||||
<td>Segmentation
|
||||
<td>Horizontal
|
||||
<td>Coco, SBDataset
|
||||
<td>
|
||||
</tr>
|
||||
<tr>
|
||||
<td rowspan=3>NLP
|
||||
<td>Classification
|
||||
<td>Horizontal
|
||||
<td>Sentiment140, AG_NEWS, sst2
|
||||
<td>
|
||||
</tr>
|
||||
<tr>
|
||||
<td>Text Prediction
|
||||
<td>Horizontal
|
||||
<td>Shakespeare, Reddit
|
||||
<td>
|
||||
</tr>
|
||||
<tr>
|
||||
<td>Translation
|
||||
<td>Horizontal
|
||||
<td>Multi30k
|
||||
<td>
|
||||
</tr>
|
||||
<tr>
|
||||
<td rowspan=3>Graph
|
||||
<td>Node Classification
|
||||
<td>Horizontal
|
||||
<td>Cora, Citeseer, Pubmed
|
||||
<td>
|
||||
</tr>
|
||||
<tr>
|
||||
<td>Link Prediction
|
||||
<td>Horizontal
|
||||
<td>Cora, Citeseer, Pubmed
|
||||
<td>
|
||||
</tr>
|
||||
<tr>
|
||||
<td>Graph Classification
|
||||
<td>Horizontal
|
||||
<td>Enzymes, Mutag
|
||||
<td>
|
||||
</tr>
|
||||
<tr>
|
||||
<td>Recommendation
|
||||
<td>Rating Prediction
|
||||
<td>Horizontal & Vertical
|
||||
<td>Ciao, Movielens, Epinions, Filmtrust, Douban
|
||||
<td>
|
||||
</tr>
|
||||
<tr>
|
||||
<td>Series
|
||||
<td>Time series forecasting
|
||||
<td>Horizontal
|
||||
<td>Electricity, Exchange Rate
|
||||
<td>
|
||||
</tr>
|
||||
<tr>
|
||||
<td>Tabular
|
||||
<td>Classification
|
||||
<td>Horizontal
|
||||
<td>Adult, Bank Marketing
|
||||
<td>
|
||||
</tr>
|
||||
<tr>
|
||||
<td>Synthetic
|
||||
<td>Regression
|
||||
<td>Horizontal
|
||||
<td>Synthetic, DistributedQP, CUBE
|
||||
<td>
|
||||
</tr>
|
||||
</table>
|
||||
|
||||
### Usage
|
||||
Each benchmark can be used to generate federated tasks that denote distributed scenes with specific data distributions like
|
||||
|
||||
```python
|
||||
import flgo
|
||||
import flgo.benchmark.cifar10_classification as cifar10
|
||||
import flgo.benchmark.partition as fbp
|
||||
import flgo.algorithm.fedavg as fedavg
|
||||
|
||||
task = './my_first_cifar' # task name
|
||||
flgo.gen_task_by_(cifar10, fbp.IIDPartitioner(num_clients=10), task) # generate task from benchmark with partitioner
|
||||
flgo.init(task, fedavg, {'gpu':0}).run()
|
||||
```
|
||||
|
||||
|
||||
## Visualized Data Heterogeneity :eyes:
|
||||
We realize data heterogeneity by flexible partitioners. These partitioners can be easily combined with `benchmark` to generate federated tasks with different data distributions.
|
||||
```python
|
||||
import flgo.benchmark.cifar10_classification as cifar10
|
||||
import flgo.benchmark.partition as fbp
|
||||
```
|
||||
#### Dirichlet(α) of labels
|
||||
```python
|
||||
flgo.gen_task_by_(cifar10, fbp.DirichletPartitioner(num_clients=100, alpha=0.1), 'dir0.1_cifar')
|
||||
flgo.gen_task_by_(cifar10, fbp.DirichletPartitioner(num_clients=100, alpha=1.0), 'dir1.0_cifar')
|
||||
flgo.gen_task_by_(cifar10, fbp.DirichletPartitioner(num_clients=100, alpha=5.0), 'dir5.0_cifar')
|
||||
flgo.gen_task_by_(cifar10, fbp.DirichletPartitioner(num_clients=100, alpha=10.0), 'dir10.0_cifar')
|
||||
```
|
||||

|
||||
#### Controllable Data Imbalance
|
||||
```python
|
||||
# set imbalance=0.1, 0.3, 0.6 or 1.0
|
||||
flgo.gen_task_by_(cifar10, fbp.DirichletPartitioner(num_clients=100, alpha=1.0, imbalance=0.1), 'dir1.0_cifar_imb0.1')
|
||||
flgo.gen_task_by_(cifar10, fbp.DirichletPartitioner(num_clients=100, alpha=1.0, imbalance=0.3), 'dir1.0_cifar_imb0.3')
|
||||
flgo.gen_task_by_(cifar10, fbp.DirichletPartitioner(num_clients=100, alpha=1.0, imbalance=0.6), 'dir1.0_cifar_imb0.6')
|
||||
flgo.gen_task_by_(cifar10, fbp.DirichletPartitioner(num_clients=100, alpha=1.0, imbalance=1.0), 'dir1.0_cifar_imb1.0')
|
||||
```
|
||||

|
||||
#### Heterogeneous Label Diversity
|
||||
```python
|
||||
flgo.gen_task_by_(cifar10, fbp.DiversityPartitioner(num_clients=100, diversity=0.1), 'div0.1_cifar')
|
||||
flgo.gen_task_by_(cifar10, fbp.DiversityPartitioner(num_clients=100, diversity=0.3), 'div0.3_cifar')
|
||||
flgo.gen_task_by_(cifar10, fbp.DiversityPartitioner(num_clients=100, diversity=0.6), 'div0.6_cifar')
|
||||
flgo.gen_task_by_(cifar10, fbp.DiversityPartitioner(num_clients=100, diversity=1.0), 'div1.0_cifar')
|
||||
```
|
||||

|
||||
|
||||
`Partitioner` is also customizable in flgo. We have provided a detailed example in this [Tutorial](https://flgo-xmu.github.io/Tutorials/3_Benchmark_Customization/3.7_Data_Heterogeneity/).
|
||||
|
||||
## Reproduction of Algorithms from TOP-tiers and Journals :1st_place_medal:
|
||||
We have realized more than 50 algorithms from TOP-tiers and Journals. The algorithms are listed as below
|
||||
|
||||
#### Classical FL & Data Heterogeneity
|
||||
|
||||
| Method | Reference | Publication |
|
||||
|----------|-----------|-------------|
|
||||
| FedAvg | [link](http://arxiv.org/abs/1602.05629) | AISTAS2017 |
|
||||
| FedProx | [link](http://arxiv.org/abs/1812.06127) | MLSys 2020 |
|
||||
| Scaffold | [link](http://arxiv.org/abs/1910.06378) | ICML 2020 |
|
||||
| FedDyn | [link](http://arxiv.org/abs/2111.04263) | ICLR 2021 |
|
||||
| MOON | [link](http://arxiv.org/abs/2103.16257) | CVPR 2021 |
|
||||
| FedNova | [link](http://arxiv.org/abs/2007.07481) | NIPS 2021 |
|
||||
| FedAvgM | [link](https://arxiv.org/abs/1909.06335) | arxiv |
|
||||
| GradMA |[link](http://arxiv.org/abs/2302.14307) | CVPR 2023 |
|
||||
|
||||
|
||||
#### Personalized FL
|
||||
| Method | Reference | Publication |
|
||||
|-----------------|-----------------------------------------------------------------|--------------------|
|
||||
| Standalone | [link](http://arxiv.org/abs/1602.05629) | - |
|
||||
| FedAvg+FineTune | - | - |
|
||||
| Ditto | [link](http://arxiv.org/abs/2007.14390) | ICML 2021 |
|
||||
| FedALA | [link](http://arxiv.org/abs/2212.01197) | AAAI 2023 |
|
||||
| FedRep | [link](http://arxiv.org/abs/2102.07078) | ICML 2021 |
|
||||
| pFedMe | [link](http://arxiv.org/abs/2006.08848) | NIPS 2020 |
|
||||
| Per-FedAvg | [link](http://arxiv.org/abs/2002.07948) | NIPS 2020 |
|
||||
| FedAMP | [link](http://arxiv.org/abs/2007.03797) | AAAI 2021 |
|
||||
| FedFomo | [link](http://arxiv.org/abs/2012.08565) | ICLR 2021 |
|
||||
| LG-FedAvg | [link](http://arxiv.org/abs/2001.01523) | NIPS 2019 workshop |
|
||||
| pFedHN | [link](https://proceedings.mlr.press/v139/shamsian21a.html) | ICML 2021 |
|
||||
| Fed-ROD | [link](https://openreview.net/forum?id=I1hQbx10Kxn) | ICLR 2023 |
|
||||
| FedPAC | [link](http://arxiv.org/abs/2306.11867) | ICLR 2023 |
|
||||
| FedPer | [link](http://arxiv.org/abs/1912.00818) | AISTATS 2020 |
|
||||
| APPLE | [link](https://www.ijcai.org/proceedings/2022/301) | IJCAI 2022 |
|
||||
| FedBABU | [link](http://arxiv.org/abs/2106.06042) | ICLR 2022 |
|
||||
| FedBN | [link](https://openreview.net/pdf?id=6YEQUn0QICG) | ICLR 2021 |
|
||||
| FedPHP | [link](https://dl.acm.org/doi/abs/10.1007/978-3-030-86486-6_36) | ECML/PKDD 2021 |
|
||||
| APFL | [link](http://arxiv.org/abs/2003.13461) | arxiv |
|
||||
| FedProto | [link](https://ojs.aaai.org/index.php/AAAI/article/view/20819) | AAAI 2022 |
|
||||
| FedCP | [link](http://arxiv.org/abs/2307.01217) | KDD 2023 |
|
||||
| GPFL | [link](http://arxiv.org/abs/2308.10279) | ICCV 2023 |
|
||||
| pFedPara | [link](http://arxiv.org/abs/2108.06098) | ICLR 2022 |
|
||||
| FedFA | [link](https://arxiv.org/abs/2301.12995) | ICLR 2023 |
|
||||
|
||||
#### Fairness-Aware FL
|
||||
| Method |Reference| Publication |
|
||||
|----------|---|---------------------------|
|
||||
| AFL |[link](http://arxiv.org/abs/1902.00146) | ICML 2019 |
|
||||
| FedFv |[link](http://arxiv.org/abs/2104.14937) | IJCAI 2021 |
|
||||
| FedFa |[link](http://arxiv.org/abs/2012.10069) | Information Sciences 2022 |
|
||||
| FedMgda+ |[link](http://arxiv.org/abs/2006.11489) | IEEE TNSE 2022 |
|
||||
| QFedAvg |[link](http://arxiv.org/abs/1905.10497) | ICLR 2020 |
|
||||
|
||||
#### Asynchronous FL
|
||||
| Method |Reference| Publication |
|
||||
|----------|---|--------------|
|
||||
| FedAsync |[link](http://arxiv.org/abs/1903.03934) | arxiv |
|
||||
| FedBuff |[link](http://arxiv.org/abs/2106.06639) | AISTATS 2022 |
|
||||
| CA2FL |[link](https://openreview.net/forum?id=4aywmeb97I) | ICLR2024 |
|
||||
#### Client Sampling & Heterogeneous Availability
|
||||
| Method |Reference| Publication |
|
||||
|-------------------|---|--------------|
|
||||
| MIFA |[link](http://arxiv.org/abs/2106.04159) | NeurIPS 2021 |
|
||||
| PowerofChoice |[link](http://arxiv.org/abs/2010.13723) | arxiv |
|
||||
| FedGS |[link](https://arxiv.org/abs/2211.13975) | AAAI 2023 |
|
||||
| ClusteredSampling |[link](http://arxiv.org/abs/2105.05883) | ICML 2021 |
|
||||
|
||||
|
||||
#### Capacity Heterogeneity
|
||||
| Method | Reference | Publication |
|
||||
|------------------|------------------------------------------------------------------------------------------------------------------------------|--------------|
|
||||
| FederatedDropout | [link](http://arxiv.org/abs/1812.07210) | arxiv |
|
||||
| FedRolex | [link](https://openreview.net/forum?id=OtxyysUdBE) | NIPS 2022 |
|
||||
| Fjord | [link](https://proceedings.neurips.cc/paper/2021/hash/6aed000af86a084f9cb0264161e29dd3-Abstract.html) | NIPS 2021 |
|
||||
| FLANC | [link](https://proceedings.neurips.cc/paper_files/paper/2022/hash/1b61ad02f2da8450e08bb015638a9007-Abstract-Conference.html) | NIPS 2022 |
|
||||
| Hermes | [link](https://dl.acm.org/doi/10.1145/3447993.3483278) | MobiCom 2021 |
|
||||
| FedMask | [link](https://dl.acm.org/doi/10.1145/3485730.3485929) | SenSys 2021 |
|
||||
| LotteryFL | [link](http://arxiv.org/abs/2008.03371) | arxiv |
|
||||
| HeteroFL | [link](http://arxiv.org/abs/2010.01264) | ICLR 2021 |
|
||||
| TailorFL | [link](https://dl.acm.org/doi/10.1145/3560905.3568503) | SenSys 2022 |
|
||||
| pFedGate | [link](http://arxiv.org/abs/2305.02776) | ICML 2023 |
|
||||
|
||||
## Combine All The Things Together! :bricks:
|
||||
<img src="https://github.com/WwZzz/myfigs/blob/master/readme_flgo_com.png?raw=true" width=500/>
|
||||
|
||||
FLGo supports flexible combinations of benchmarks, partitioners, algorithms and simulators , which are independent to each other and thus can be used like plugins. We have provided these plugins [here](https://github.com/WwZzz/easyFL/tree/FLGo/resources) , where each can be immediately downloaded and used by API
|
||||
|
||||
```python
|
||||
import flgo
|
||||
import flgo.benchmark.partition as fbp
|
||||
|
||||
fedavg = flgo.download_resource(root='.', name='fedavg', type='algorithm')
|
||||
mnist = flgo.download_resource(root='.', name='mnist_classification', type='benchmark')
|
||||
task = 'test_down_mnist'
|
||||
flgo.gen_task_by_(mnist,fbp.IIDPartitioner(num_clients=10,), task_path=task)
|
||||
flgo.init(task, fedavg, {'gpu':0}).run()
|
||||
```
|
||||
|
||||
[//]: # (## Multiple Communication Topology Support)
|
||||
## Easy-to-use Experimental Tools :toolbox:
|
||||
### Load Results
|
||||
Each runned result will be automatically saved in `task_path/record/`. We provide an API to easily load and filter records.
|
||||
```python
|
||||
import flgo
|
||||
import flgo.experiment.analyzer as fea
|
||||
import matplotlib.pyplot as plt
|
||||
res = fea.Selector({'task': './my_task', 'header':['fedavg',], },)
|
||||
log_data = res.records['./my_task'][0].data
|
||||
val_loss = log_data['val_loss']
|
||||
plt.plot(list(range(len(val_loss))), val_loss)
|
||||
plt.show()
|
||||
```
|
||||
|
||||
### Use Checkpoint
|
||||
```python
|
||||
import flgo.algorithm.fedavg as fedavg
|
||||
import flgo.experiment.analyzer
|
||||
|
||||
task = './my_task'
|
||||
ckpt = '1'
|
||||
runner = flgo.init(task, fedavg, {'gpu':[0,],'log_file':True, 'num_epochs':1, 'save_checkpoint':ckpt, 'load_checkpoint':ckpt})
|
||||
runner.run()
|
||||
```
|
||||
We save each checkpoint at `task_path/checkpoint/checkpoint_name/`. By specifying the name of checkpoints, the training can be automatically recovered from them.
|
||||
```python
|
||||
import flgo.algorithm.fedavg as fedavg
|
||||
# the two methods need to be extended when using other algorithms
|
||||
class Server(fedavg.Server):
|
||||
def save_checkpoint(self):
|
||||
cpt = {
|
||||
'round': self.current_round, # current communication round
|
||||
'learning_rate': self.learning_rate, # learning rate
|
||||
'model_state_dict': self.model.state_dict(), # model
|
||||
'early_stop_option': { # early stop option
|
||||
'_es_best_score': self.gv.logger._es_best_score,
|
||||
'_es_best_round': self.gv.logger._es_best_round,
|
||||
'_es_patience': self.gv.logger._es_patience,
|
||||
},
|
||||
'output': self.gv.logger.output, # recorded information by Logger
|
||||
'time': self.gv.clock.current_time, # virtual time
|
||||
}
|
||||
return cpt
|
||||
|
||||
def load_checkpoint(self, cpt):
|
||||
md = cpt.get('model_state_dict', None)
|
||||
round = cpt.get('round', None)
|
||||
output = cpt.get('output', None)
|
||||
early_stop_option = cpt.get('early_stop_option', None)
|
||||
time = cpt.get('time', None)
|
||||
learning_rate = cpt.get('learning_rate', None)
|
||||
if md is not None: self.model.load_state_dict(md)
|
||||
if round is not None: self.current_round = round + 1
|
||||
if output is not None: self.gv.logger.output = output
|
||||
if time is not None: self.gv.clock.set_time(time)
|
||||
if learning_rate is not None: self.learning_rate = learning_rate
|
||||
if early_stop_option is not None:
|
||||
self.gv.logger._es_best_score = early_stop_option['_es_best_score']
|
||||
self.gv.logger._es_best_round = early_stop_option['_es_best_round']
|
||||
self.gv.logger._es_patience = early_stop_option['_es_patience']
|
||||
```
|
||||
**Note**: different FL algorithms need to save different types of checkpoints. Here we only provide checkpoint save&load mechanism of FedAvg. We remain two APIs for customization above:
|
||||
|
||||
### Use Logger
|
||||
We show how to use customized Logger [Here](https://flgo-xmu.github.io/Tutorials/1_Configuration/1.6_Logger_Configuration/)
|
||||
## Tutorials and Documents :page_with_curl:
|
||||
We have provided comprehensive [Tutorials](https://flgo-xmu.github.io/Tutorials/) and [Document](https://flgo-xmu.github.io/Docs/FLGo/) for FLGo.
|
||||
## Deployment To Real Machines :computer:
|
||||
Our FLGo is able to be extended to real-world application. We provide a simple [Example](https://github.com/WwZzz/easyFL/tree/FLGo/example/realworld_case) to show how to run FLGo on multiple machines.
|
||||
|
||||
|
||||
|
||||
|
||||
# Overview :notebook:
|
||||
|
||||
### Options
|
||||
|
||||
Basic options:
|
||||
|
||||
* `task` is to choose the task of splited dataset. Options: name of fedtask (e.g. `mnist_classification_client100_dist0_beta0_noise0`).
|
||||
|
||||
* `algorithm` is to choose the FL algorithm. Options: `fedfv`, `fedavg`, `fedprox`, …
|
||||
|
||||
* `model` should be the corresponding model of the dataset. Options: `mlp`, `cnn`, `resnet18.`
|
||||
|
||||
Server-side options:
|
||||
|
||||
* `sample` decides the way to sample clients in each round. Options: `uniform` means uniformly, `md` means choosing with probability.
|
||||
|
||||
* `aggregate` decides the way to aggregate clients' model. Options: `uniform`, `weighted_scale`, `weighted_com`
|
||||
|
||||
* `num_rounds` is the number of communication rounds.
|
||||
|
||||
* `proportion` is the proportion of clients to be selected in each round.
|
||||
|
||||
* `lr_scheduler` is the global learning rate scheduler.
|
||||
|
||||
* `learning_rate_decay` is the decay rate of the learning rate.
|
||||
|
||||
Client-side options:
|
||||
|
||||
* `num_epochs` is the number of local training epochs.
|
||||
|
||||
* `num_steps` is the number of local updating steps and the default value is -1. If this term is set larger than 0, `num_epochs` is not valid.
|
||||
|
||||
* `learning_rate ` is the step size when locally training.
|
||||
|
||||
* `batch_size ` is the size of one batch data during local training. `batch_size = full_batch` if `batch_size==-1` and `batch_size=|Di|*batch_size` if `1>batch_size>0`.
|
||||
|
||||
* `optimizer` is to choose the optimizer. Options: `SGD`, `Adam`.
|
||||
|
||||
* `weight_decay` is to set ratio for weight decay during the local training process.
|
||||
|
||||
* `momentum` is the ratio of the momentum item when the optimizer SGD taking each step.
|
||||
|
||||
Real Machine-Dependent options:
|
||||
|
||||
* `seed ` is the initial random seed.
|
||||
|
||||
* `gpu ` is the id of the GPU device. (e.g. CPU is used without specifying this term. `--gpu 0` will use device GPU 0, and `--gpu 0 1 2 3` will use the specified 4 GPUs when `num_threads`>0.
|
||||
|
||||
* `server_with_cpu ` is set False as default value,..
|
||||
|
||||
* `test_batch_size ` is the batch_size used when evaluating models on validation datasets, which is limited by the free space of the used device.
|
||||
|
||||
* `eval_interval ` controls the interval between every two evaluations.
|
||||
|
||||
* `num_threads` is the number of threads in the clients computing session that aims to accelerate the training process.
|
||||
|
||||
* `num_workers` is the number of workers of the torch.utils.data.Dataloader
|
||||
|
||||
Additional hyper-parameters for particular federated algorithms:
|
||||
|
||||
* `algo_para` is used to receive the algorithm-dependent hyper-parameters from command lines. Usage: 1) The hyper-parameter will be set as the default value defined in Server.__init__() if not specifying this term, 2) For algorithms with one or more parameters, use `--algo_para v1 v2 ...` to specify the values for the parameters. The input order depends on the dict `Server.algo_para` defined in `Server.__init__()`.
|
||||
|
||||
Logger's setting
|
||||
|
||||
* `logger` is used to selected the logger that has the same name with this term.
|
||||
|
||||
* `log_level` shares the same meaning with the LEVEL in the python's native module logging.
|
||||
|
||||
* `log_file` controls whether to store the running-time information into `.log` in `fedtask/taskname/log/`, default value is false.
|
||||
|
||||
* `no_log_console` controls whether to show the running time information on the console, and default value is false.
|
||||
|
||||
### More
|
||||
|
||||
To get more information and full-understanding of FLGo please refer to <a href='https://flgo-xmu.github.io/'>our website</a>.
|
||||
|
||||
In the website, we offer :
|
||||
|
||||
- API docs: Detailed introduction of packages, classes and methods.
|
||||
- Tutorial: Materials that help user to master FLGo.
|
||||
|
||||
## Architecture
|
||||
|
||||
We seperate the FL system into five parts:`algorithm`, `benchmark`, `experiment`, `simulator` and `utils`.
|
||||
```
|
||||
├─ algorithm
|
||||
│ ├─ fedavg.py //fedavg algorithm
|
||||
│ ├─ ...
|
||||
│ ├─ fedasync.py //the base class for asynchronous federated algorithms
|
||||
│ └─ fedbase.py //the base class for federated algorithms
|
||||
├─ benchmark
|
||||
│ ├─ mnist_classification //classification on mnist dataset
|
||||
│ │ ├─ model //the corresponding model
|
||||
│ | └─ core.py //the core supporting for the dataset, and each contains three necessary classes(e.g. TaskGen, TaskReader, TaskCalculator)
|
||||
│ ├─ ...
|
||||
│ ├─ RAW_DATA // storing the downloaded raw dataset
|
||||
│ └─ toolkits //the basic tools for generating federated dataset
|
||||
│ ├─ cv // common federal division on cv
|
||||
│ │ ├─ horizontal // horizontal fedtask
|
||||
│ │ │ └─ image_classification.py // the base class for image classification
|
||||
│ │ └─ ...
|
||||
│ ├─ ...
|
||||
│ ├─ base.py // the base class for all fedtask
|
||||
│ ├─ partition.py // the parttion class for federal division
|
||||
│ └─ visualization.py // visualization after the data set is divided
|
||||
├─ experiment
|
||||
│ ├─ logger //the class that records the experimental process
|
||||
│ │ ├─ basic_logger.py //the base logger class
|
||||
│ | └─ simple_logger.py //a simple logger class
|
||||
│ ├─ analyzer.py //the class for analyzing and printing experimental results
|
||||
│ ├─ res_config.yml //hyperparameter file of analyzer.py
|
||||
│ ├─ run_config.yml //hyperparameter file of runner.py
|
||||
| └─ runner.py //the class for generating experimental commands based on hyperparameter combinations and processor scheduling for all experimental
|
||||
├─ system_simulator //system heterogeneity simulation module
|
||||
│ ├─ base.py //the base class for simulate system heterogeneity
|
||||
│ ├─ default_simulator.py //the default class for simulate system heterogeneity
|
||||
| └─ ...
|
||||
├─ utils
|
||||
│ ├─ fflow.py //option to read, initialize,...
|
||||
│ └─ fmodule.py //model-level operators
|
||||
└─ requirements.txt
|
||||
```
|
||||
|
||||
### Benchmark
|
||||
|
||||
We have added many benchmarks covering several different areas such as CV, NLP, etc
|
||||
|
||||
### Algorithm
|
||||

|
||||
This module is the specific federated learning algorithm implementation. Each method contains two classes: the `Server` and the `Client`.
|
||||
|
||||
|
||||
#### Server
|
||||
|
||||
The whole FL system starts with the `main.py`, which runs `server.run()` after initialization. Then the server repeat the method `iterate()` for `num_rounds` times, which simulates the communication process in FL. In the `iterate()`, the `BaseServer` start with sampling clients by `select()`, and then exchanges model parameters with them by `communicate()`, and finally aggregate the different models into a new one with `aggregate()`. Therefore, anyone who wants to customize its own method that specifies some operations on the server-side should rewrite the method `iterate()` and particular methods mentioned above.
|
||||
|
||||
#### Client
|
||||
|
||||
The clients reponse to the server after the server `communicate_with()` them, who first `unpack()` the received package and then train the model with their local dataset by `train()`. After training the model, the clients `pack()` send package (e.g. parameters, loss, gradient,... ) to the server through `reply()`.
|
||||
|
||||
|
||||
### Experiment
|
||||
|
||||
The experiment module contains experiment command generation and scheduling operation, which can help FL researchers more conveniently conduct experiments in the field of federated learning.
|
||||
|
||||
### simulator
|
||||
|
||||
The system_simulator module is used to realize the simulation of heterogeneous systems, and we set multiple states such as network speed and availability to better simulate the system heterogeneity of federated learning parties.
|
||||
|
||||
### Utils
|
||||
|
||||
Utils is composed of commonly used operations:
|
||||
1) model-level operation (we convert model layers and parameters to dictionary type and apply it in the whole FL system).
|
||||
2) API for the FL workflow like gen_benchmark, gen_task, init, ...
|
||||
## Citation
|
||||
|
||||
Please cite our paper in your publications if this code helps your research.
|
||||
|
||||
```
|
||||
@misc{wang2021federated,
|
||||
title={Federated Learning with Fair Averaging},
|
||||
author={Zheng Wang and Xiaoliang Fan and Jianzhong Qi and Chenglu Wen and Cheng Wang and Rongshan Yu},
|
||||
year={2021},
|
||||
eprint={2104.14937},
|
||||
archivePrefix={arXiv},
|
||||
primaryClass={cs.LG}
|
||||
}
|
||||
|
||||
@misc{wang2023flgo,
|
||||
title={FLGo: A Fully Customizable Federated Learning Platform},
|
||||
author={Zheng Wang and Xiaoliang Fan and Zhaopeng Peng and Xueheng Li and Ziqi Yang and Mingkuan Feng and Zhicheng Yang and Xiao Liu and Cheng Wang},
|
||||
year={2023},
|
||||
eprint={2306.12079},
|
||||
archivePrefix={arXiv},
|
||||
primaryClass={cs.LG}
|
||||
}
|
||||
```
|
||||
|
||||
## Contacts
|
||||
Zheng Wang, zwang@stu.xmu.edu.cn
|
||||
|
||||
|
||||
# Buy Me a Coffee :coffee:
|
||||
Buy me a coffee if you'd like to support the development of this repo.
|
||||
<center>
|
||||
<img src="https://github.com/WwZzz/easyFL/assets/20792079/89050169-3927-4eb0-ac32-68d8bee12326" width=180/>
|
||||
</center>
|
||||
|
||||
## References
|
||||
<div id='refer-anchor-1'></div>
|
||||
|
||||
\[McMahan. et al., 2017\] [Brendan McMahan, Eider Moore, Daniel Ramage, Seth Hampson, and Blaise Aguera y Arcas. Communication-Efficient Learning of Deep Networks from Decentralized Data. In International Conference on Artificial Intelligence and Statistics (AISTATS), 2017.](https://arxiv.org/abs/1602.05629)
|
||||
|
||||
<div id='refer-anchor-2'></div>
|
||||
|
||||
\[Cong Xie. et al., 2019\] [Cong Xie, Sanmi Koyejo, Indranil Gupta. Asynchronous Federated Optimization. ](https://arxiv.org/abs/1903.03934)
|
||||
|
||||
<div id='refer-anchor-3'></div>
|
||||
|
||||
\[John Nguyen. et al., 2022\] [John Nguyen, Kshitiz Malik, Hongyuan Zhan, Ashkan Yousefpour, Michael Rabbat, Mani Malek, Dzmitry Huba. Federated Learning with Buffered Asynchronous Aggregation. In International Conference on Artificial Intelligence and Statistics (AISTATS), 2022.](https://arxiv.org/abs/2106.06639)
|
||||
|
||||
<div id='refer-anchor-4'></div>
|
||||
|
||||
\[Zheng Chai. et al., 2020\] [Zheng Chai, Ahsan Ali, Syed Zawad, Stacey Truex, Ali Anwar, Nathalie Baracaldo, Yi Zhou, Heiko Ludwig, Feng Yan, Yue Cheng. TiFL: A Tier-based Federated Learning System.In International Symposium on High-Performance Parallel and Distributed Computing(HPDC), 2020](https://arxiv.org/abs/2106.06639)
|
||||
|
||||
<div id='refer-anchor-5'></div>
|
||||
|
||||
\[Mehryar Mohri. et al., 2019\] [Mehryar Mohri, Gary Sivek, Ananda Theertha Suresh. Agnostic Federated Learning.In International Conference on Machine Learning(ICML), 2019](https://arxiv.org/abs/1902.00146)
|
||||
|
||||
<div id='refer-anchor-6'></div>
|
||||
|
||||
\[Zheng Wang. et al., 2021\] [Zheng Wang, Xiaoliang Fan, Jianzhong Qi, Chenglu Wen, Cheng Wang, Rongshan Yu. Federated Learning with Fair Averaging. In International Joint Conference on Artificial Intelligence, 2021](https://arxiv.org/abs/2104.14937#)
|
||||
|
||||
<div id='refer-anchor-7'></div>
|
||||
|
||||
\[Zeou Hu. et al., 2022\] [Zeou Hu, Kiarash Shaloudegi, Guojun Zhang, Yaoliang Yu. Federated Learning Meets Multi-objective Optimization. In IEEE Transactions on Network Science and Engineering, 2022](https://arxiv.org/abs/2006.11489)
|
||||
|
||||
<div id='refer-anchor-8'></div>
|
||||
|
||||
\[Tian Li. et al., 2020\] [Tian Li, Anit Kumar Sahu, Manzil Zaheer, Maziar Sanjabi, Ameet Talwalkar, Virginia Smith. Federated Optimization in Heterogeneous Networks. In Conference on Machine Learning and Systems, 2020](https://arxiv.org/abs/1812.06127)
|
||||
|
||||
<div id='refer-anchor-9'></div>
|
||||
|
||||
\[Xinran Gu. et al., 2021\] [Xinran Gu, Kaixuan Huang, Jingzhao Zhang, Longbo Huang. Fast Federated Learning in the Presence of Arbitrary Device Unavailability. In Neural Information Processing Systems(NeurIPS), 2021](https://arxiv.org/abs/2106.04159)
|
||||
|
||||
<div id='refer-anchor-10'></div>
|
||||
|
||||
\[Yae Jee Cho. et al., 2020\] [Yae Jee Cho, Jianyu Wang, Gauri Joshi. Client Selection in Federated Learning: Convergence Analysis and Power-of-Choice Selection Strategies. ](https://arxiv.org/abs/2010.01243)
|
||||
|
||||
<div id='refer-anchor-11'></div>
|
||||
|
||||
\[Tian Li. et al., 2020\] [Tian Li, Maziar Sanjabi, Ahmad Beirami, Virginia Smith. Fair Resource Allocation in Federated Learning. In International Conference on Learning Representations, 2020](https://arxiv.org/abs/1905.10497)
|
||||
|
||||
<div id='refer-anchor-12'></div>
|
||||
|
||||
\[Sai Praneeth Karimireddy. et al., 2020\] [Sai Praneeth Karimireddy, Satyen Kale, Mehryar Mohri, Sashank J. Reddi, Sebastian U. Stich, Ananda Theertha Suresh. SCAFFOLD: Stochastic Controlled Averaging for Federated Learning. In International Conference on Machine Learning, 2020](https://arxiv.org/abs/1910.06378)
|
||||
|
|
@ -0,0 +1,29 @@
|
|||
#choose a fitfal version of pytorch image according to your cuda and modify the arguement TORCH_VERSION
|
||||
#Reference website:https://hub.docker.com/r/pytorch/pytorch/tags
|
||||
ARG TORCH_VERSION=1.9.0-cuda10.2-cudnn7-runtime
|
||||
FROM pytorch/pytorch:${TORCH_VERSION}
|
||||
|
||||
# update & configure cuda and pip
|
||||
RUN pip install --upgrade pip \
|
||||
& conda update -n base -c https://mirrors.tuna.tsinghua.edu.cn/anaconda/cloud/conda-forge conda \
|
||||
& conda config --add channels https://mirrors.tuna.tsinghua.edu.cn/anaconda/pkgs/free/ \
|
||||
& conda config --add channels https://mirrors.tuna.tsinghua.edu.cn/anaconda/cloud/peterjc123/ \
|
||||
& conda config --add channels https://mirrors.tuna.tsinghua.edu.cn/anaconda/cloud/msys2/ \
|
||||
& conda config --add channels https://mirrors.tuna.tsinghua.edu.cn/anaconda/cloud/conda-forge \
|
||||
& conda config --add channels https://mirrors.tuna.tsinghua.edu.cn/anaconda/pkgs/free/ \
|
||||
& conda config --add channels https://mirrors.tuna.tsinghua.edu.cn/anaconda/pkgs/main/ \
|
||||
& conda config --set show_channel_urls yes
|
||||
|
||||
#download requirements
|
||||
RUN pip install cvxopt \
|
||||
& conda install scipy \
|
||||
& pip install matplotlib \
|
||||
& pip install prettytable \
|
||||
& pip install ujson \
|
||||
& pip install pyyaml \
|
||||
& pip install pynvml \
|
||||
& pip install pandas
|
||||
|
||||
|
||||
#install flgo
|
||||
RUN pip install flgo
|
|
@ -0,0 +1,21 @@
|
|||
|
||||
# How to use the Dcokerfile
|
||||
|
||||
|
||||
- Step 1
|
||||
|
||||
Modify the Dockerfile accorrding to the comment to build a basic pytorch environment
|
||||
|
||||
- Step 2
|
||||
|
||||
Build the images using the command:
|
||||
> $ docker build -t flgo .
|
||||
|
||||
- Note
|
||||
|
||||
- Step 3
|
||||
|
||||
Create a container using the command:
|
||||
|
||||
> $ docker run -itd --gpus all --network=host flgo /bin/bash
|
||||
|
|
@ -0,0 +1 @@
|
|||
::: flgo
|
|
@ -0,0 +1 @@
|
|||
:::flgo.algorithm.decentralized
|
|
@ -0,0 +1 @@
|
|||
:::flgo.algorithm.fedbase
|
|
@ -0,0 +1 @@
|
|||
:::flgo.algorithm.hierarchical
|
|
@ -0,0 +1,7 @@
|
|||
::: flgo.algorithm
|
||||
handler: python
|
||||
options:
|
||||
show_root_heading: true
|
||||
heading_level: 1
|
||||
show_source: false
|
||||
|
|
@ -0,0 +1 @@
|
|||
:::flgo.algorithm.vflbase
|
|
@ -0,0 +1 @@
|
|||
:::flgo.benchmark.base
|
|
@ -0,0 +1,6 @@
|
|||
::: flgo.benchmark
|
||||
handler: python
|
||||
options:
|
||||
show_root_heading: true
|
||||
heading_level: 1
|
||||
show_source: false
|
|
@ -0,0 +1 @@
|
|||
:::flgo.benchmark.partition
|
|
@ -0,0 +1 @@
|
|||
:::flgo.benchmark.toolkits.cv.classification
|
|
@ -0,0 +1 @@
|
|||
:::flgo.benchmark.toolkits.cv.detection
|
|
@ -0,0 +1 @@
|
|||
:::flgo.benchmark.toolkits.cv.segmentation
|
|
@ -0,0 +1 @@
|
|||
:::flgo.benchmark.toolkits.graph.graph_classification
|
|
@ -0,0 +1 @@
|
|||
:::flgo.benchmark.toolkits.graph.link_prediction
|
|
@ -0,0 +1 @@
|
|||
:::flgo.benchmark.toolkits.graph.node_classification
|
|
@ -0,0 +1 @@
|
|||
:::flgo.benchmark.toolkits.nlp.classification
|
|
@ -0,0 +1 @@
|
|||
:::flgo.benchmark.toolkits.nlp.language_modeling
|
|
@ -0,0 +1 @@
|
|||
:::flgo.benchmark.toolkits.nlp.translation
|
|
@ -0,0 +1 @@
|
|||
:::flgo.benchmark.toolkits.partition
|
|
@ -0,0 +1 @@
|
|||
:::flgo.benchmark.toolkits.visualization
|
|
@ -0,0 +1,35 @@
|
|||
::: flgo.algorithm
|
||||
handler: python
|
||||
options:
|
||||
show_root_heading: true
|
||||
heading_level: 1
|
||||
show_source: false
|
||||
|
||||
::: flgo.benchmark
|
||||
handler: python
|
||||
options:
|
||||
show_root_heading: true
|
||||
heading_level: 1
|
||||
show_source: false
|
||||
|
||||
::: flgo.experiment
|
||||
handler: python
|
||||
options:
|
||||
show_root_heading: true
|
||||
heading_level: 1
|
||||
show_source: false
|
||||
|
||||
::: flgo.simulator
|
||||
handler: python
|
||||
options:
|
||||
show_root_heading: true
|
||||
heading_level: 1
|
||||
show_source: false
|
||||
|
||||
::: flgo.utils
|
||||
handler: python
|
||||
options:
|
||||
show_root_heading: true
|
||||
heading_level: 1
|
||||
show_source: false
|
||||
|
|
@ -0,0 +1 @@
|
|||
To be implemented...
|
|
@ -0,0 +1 @@
|
|||
:::flgo.experiment.analyzer
|
|
@ -0,0 +1 @@
|
|||
:::flgo.experiment.device_scheduler
|
|
@ -0,0 +1,6 @@
|
|||
::: flgo.experiment
|
||||
handler: python
|
||||
options:
|
||||
show_root_heading: true
|
||||
heading_level: 1
|
||||
show_source: false
|
|
@ -0,0 +1 @@
|
|||
:::flgo.experiment.logger.BasicLogger
|
|
@ -0,0 +1 @@
|
|||
:::flgo.experiment.logger.BasicLogger
|
|
@ -0,0 +1 @@
|
|||
:::flgo.simulator.base
|
|
@ -0,0 +1 @@
|
|||
:::flgo.simulator.default_simulator
|
|
@ -0,0 +1,6 @@
|
|||
::: flgo.simulator
|
||||
handler: python
|
||||
options:
|
||||
show_root_heading: true
|
||||
heading_level: 1
|
||||
show_source: false
|
|
@ -0,0 +1 @@
|
|||
:::flgo.utils.fflow
|
|
@ -0,0 +1 @@
|
|||
:::flgo.utils.fmodule
|
|
@ -0,0 +1,19 @@
|
|||
# Algorithm
|
||||
In FLGo, each algorithm is described by an independent file consisting of the objects
|
||||
(i.e. server and clients in horizontal FL) with their actions.
|
||||
## Horizontal FL
|
||||
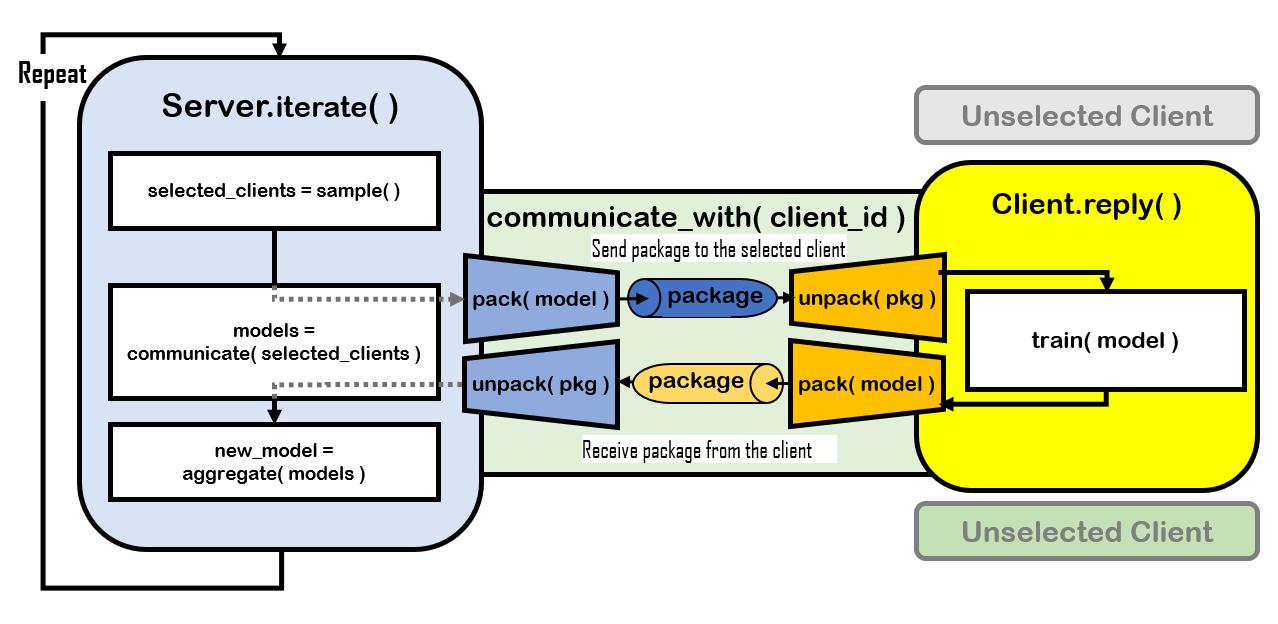
|
||||
A classical procedure of FL training process is as shown in the figure above, where the server iteratively
|
||||
broadcasts the global model to a subset of clients and aggregates the received locally
|
||||
trained models from them. Following this scheme, a great number of FL algorithms can be
|
||||
easily implemented by FLGo. For example, to implement methods that customize the local
|
||||
training process (e.g. FedProx, MOON), developers only need to modify the function
|
||||
`client.train(...)`. And a series of sampling strategies can be realized by only replacing
|
||||
the function `server.sample() `. We also provide comprehensive tutorial for using FLGo
|
||||
to implement the state of the art algorithms. In addition, asynchronous algorithms can
|
||||
share the same scheme with synchronous algorithms in FLGo, where developers only need to
|
||||
concern about the sampling strategy and how to deal with the currently received packages
|
||||
from clients at each moment.
|
||||
|
||||
## Vertical FL
|
||||
To be completed.
|
|
@ -0,0 +1,18 @@
|
|||
# Benchmark
|
||||
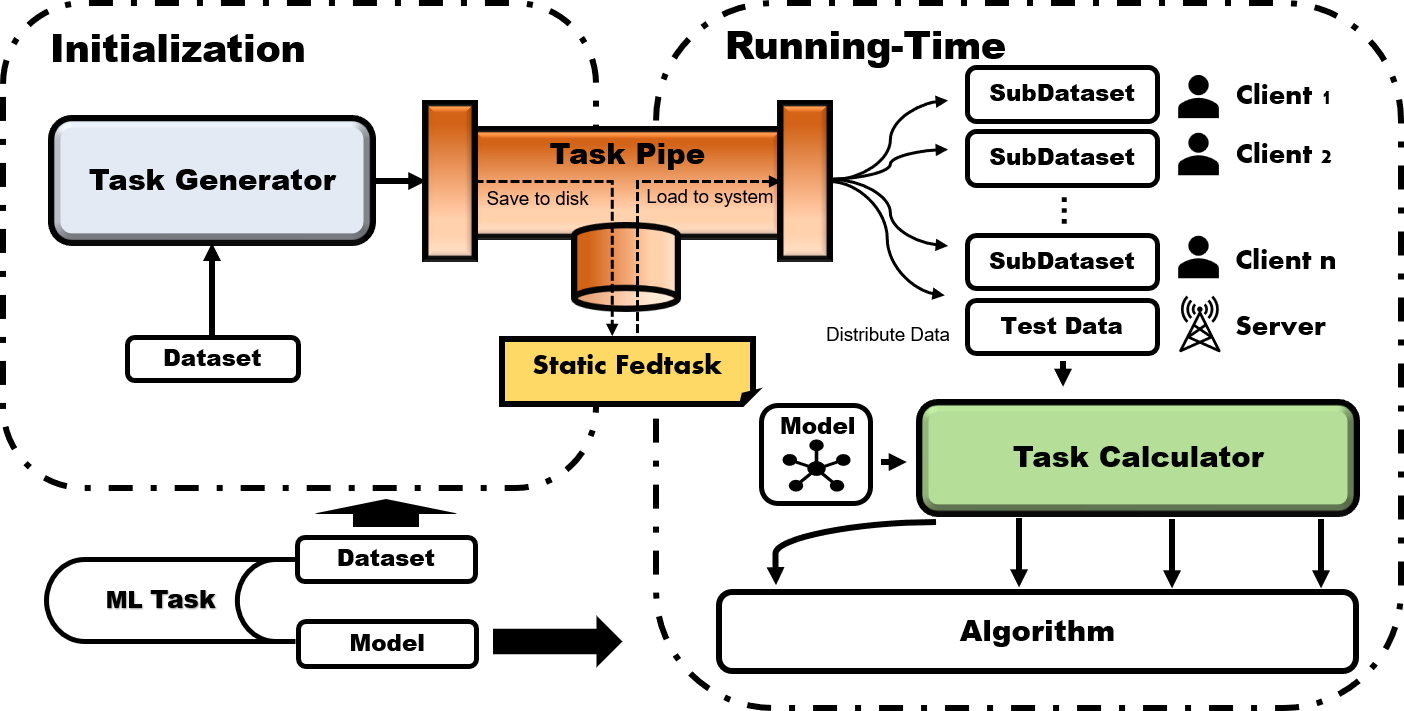
|
||||
|
||||
At the initialization phase, the original dataset is input to `TaskGenerator` that
|
||||
accordingly and flexibly partitions the dataset into local sub-datasets owned by
|
||||
clients and a testing dataset owned the server. And the local data is further divided
|
||||
to training part and validation part for hyper-parameter tuning purpose. Then, all of
|
||||
the division information on the original dataset will be stored by `TaskPipe` into
|
||||
the disk as a static `fedtask`, where different federated algorithms can fairly
|
||||
compare with each other on the same fedtask with a particular model.
|
||||
|
||||
During the running-time phase, `TaskPipe` first distributes the partitioned datasets
|
||||
to clients and the server after loading the saved partition information and the original
|
||||
dataset into memory. After the model training starts, Algorithm module can either use the
|
||||
presetting `TaskCalculator` APIs to complement the task-specific calculations (i.e. loss
|
||||
computation, transferring data across devices, evaluation, batching data) or optimize in
|
||||
customized way. In this manner, the task-relevant details will be blinded to the algorithm
|
||||
for most cases, which significantly eases the development of new algorithms.
|
|
@ -0,0 +1,14 @@
|
|||
# Logger and analyzer
|
||||
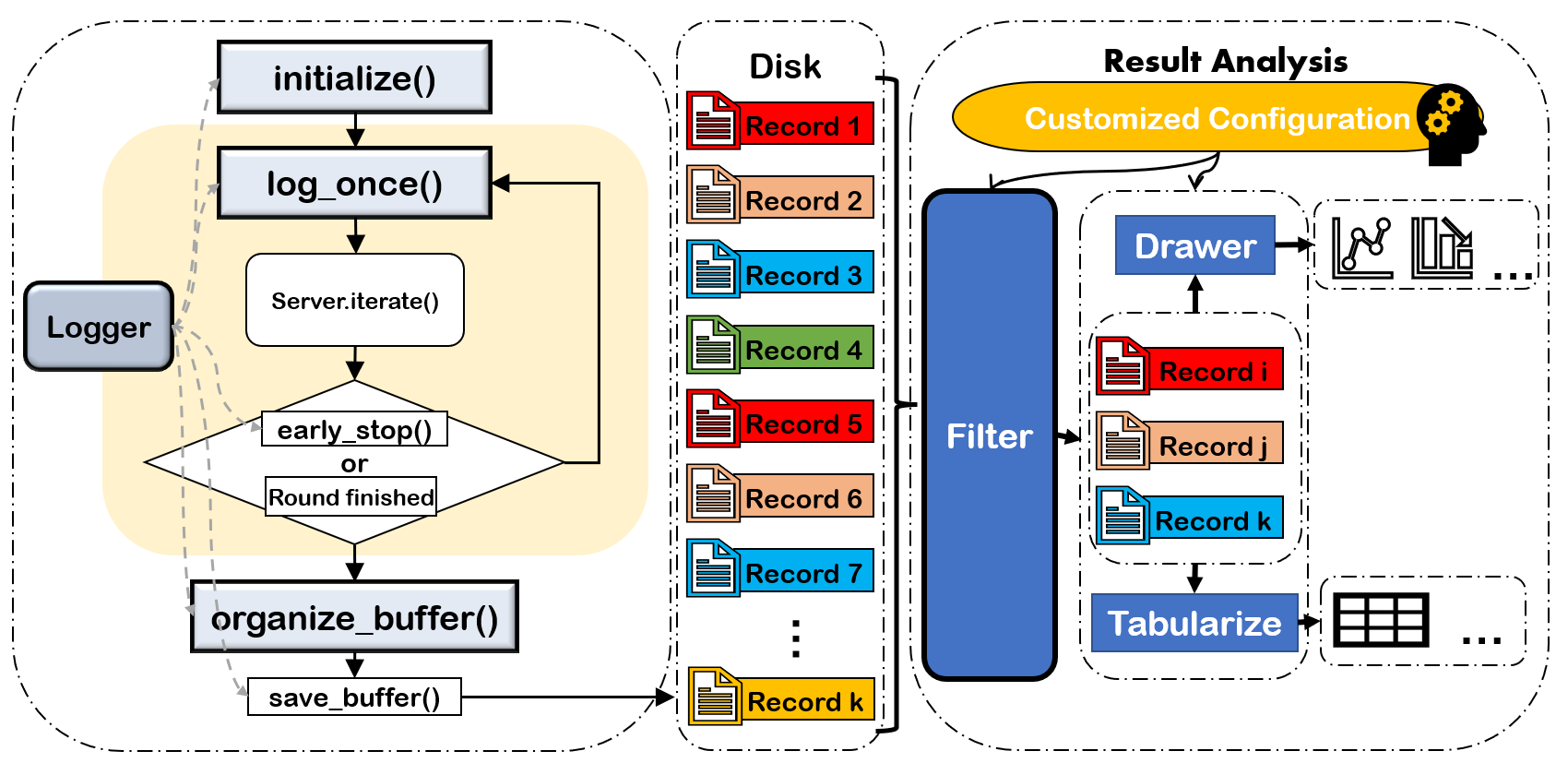
|
||||
Although there are already several comprehensive experiment managers (e.g. wandb,
|
||||
tensorboard), our `Experiment` module is compatible with them and enable
|
||||
customizing experiments in a non-intrusive way to the codes, where users can create a
|
||||
`logger` by modifying some APIs to track variables of interest and specify the customized
|
||||
`logger` in optional parameters.
|
||||
|
||||
After the `logger` stores the running-time information into records, the `analyzer` can read
|
||||
them from the disk. A filter is designed to enable only selecting records of interest, and
|
||||
several APIs are provided for quickly visualizing and analyzing the results by few codes.
|
||||
|
||||
# Device Scheduler
|
||||
To be complete.
|
|
@ -0,0 +1,60 @@
|
|||
# FLGo Framework
|
||||
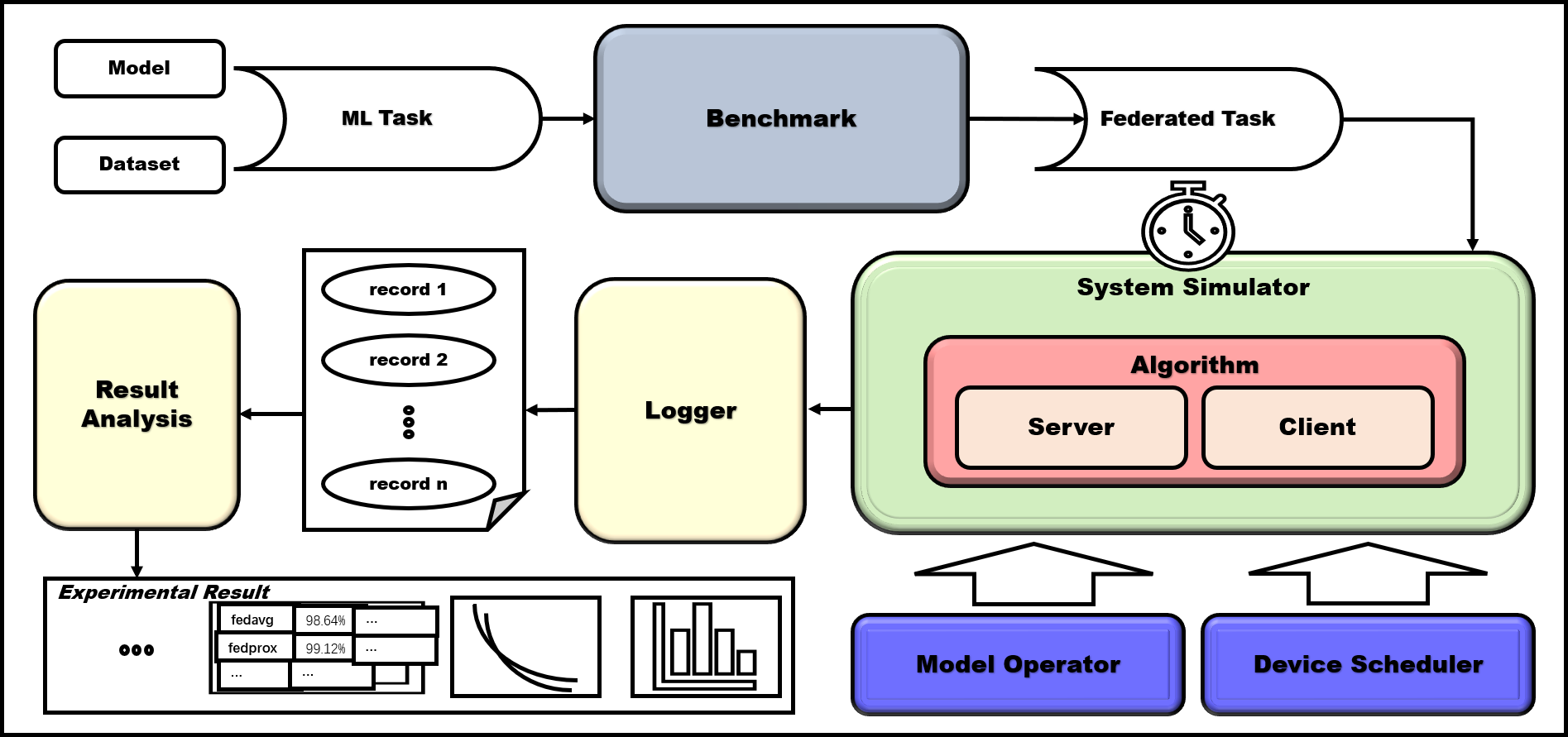
|
||||
|
||||
The whole workflow of FLGo is as shown in the above picture. FLGo framework mainly runs
|
||||
by three steps.
|
||||
|
||||
Firstly, given a ML task (i.e. dataset and model), FLGo converts it into a static federated
|
||||
task through partitioning the original ML dataset into subsets of data owned by different
|
||||
clients, and hide the task-specific details to the algorithms.
|
||||
|
||||
Secondly, different federated algorithms can run on the fed static federated task to train
|
||||
a particular model (e.g. CNN, MLP) . During training phase, the system simulator will create
|
||||
a simulated environment where a virtual global clock can fairly measure the time and arbitrary
|
||||
client behaviors can be modeled, which is also transparent to the implementation of algorithms.
|
||||
|
||||
Finally, the experimental tracker in FLGo is responsible for tracing the running-time information
|
||||
and organizing the results into tables or figures.
|
||||
|
||||
The organization of all the modules is as below
|
||||
|
||||
```
|
||||
├─ algorithm
|
||||
│ ├─ fedavg.py //fedavg algorithm
|
||||
│ ├─ ...
|
||||
│ ├─ fedasync.py //the base class for asynchronous federated algorithms
|
||||
│ └─ fedbase.py //the base class for federated algorithms
|
||||
|
|
||||
├─ benchmark
|
||||
│ ├─ mnist_classification //classification on mnist dataset
|
||||
│ │ ├─ model //the corresponding model
|
||||
│ | └─ core.py //the core supporting for the dataset, and each contains three necessary classes(e.g. TaskGen, TaskReader, TaskCalculator)
|
||||
│ ├─ base.py // the base class for all fedtask
|
||||
│ ├─ ...
|
||||
│ ├─ RAW_DATA // storing the downloaded raw dataset
|
||||
│ └─ toolkits //the basic tools for generating federated dataset
|
||||
│ ├─ cv // common federal division on cv
|
||||
│ │ ├─ horizontal // horizontal fedtask
|
||||
│ │ │ └─ image_classification.py // the base class for image classification
|
||||
│ │ └─ ...
|
||||
│ ├─ ...
|
||||
│ ├─ partition.py // the parttion class for federal division
|
||||
│ └─ visualization.py // visualization after the data set is divided
|
||||
|
|
||||
├─ experiment
|
||||
│ ├─ logger //the class that records the experimental process
|
||||
│ │ ├─ ...
|
||||
│ | └─ simple_logger.py //a simple logger class
|
||||
│ ├─ analyzer.py //the class for analyzing and printing experimental results
|
||||
| └─ device_scheduler.py // automatically schedule GPUs to run in parallel
|
||||
|
|
||||
├─ simulator //system heterogeneity simulation module
|
||||
│ ├─ base.py //the base class for simulate system heterogeneity
|
||||
│ ├─ default_simulator.py //the default class for simulate system heterogeneity
|
||||
| └─ ...
|
||||
|
|
||||
├─ utils
|
||||
│ ├─ fflow.py //option to read, initialize,...
|
||||
│ └─ fmodule.py //model-level operators
|
||||
└─ requirements.txt
|
||||
```
|
|
@ -0,0 +1,10 @@
|
|||
## Simulation with Client-State Machine
|
||||
We construct a client-state machine to simulate arbitrary system heterogeneiry. In
|
||||
this state machine, a client's state will change as time goes by or some particular
|
||||
actions were taken. For example, a client will be available with a probability at each
|
||||
moment, and clients will be in state 'working' after they were selected if not dropping out.
|
||||
The transfer rules across states are described in the figure below
|
||||
|
||||
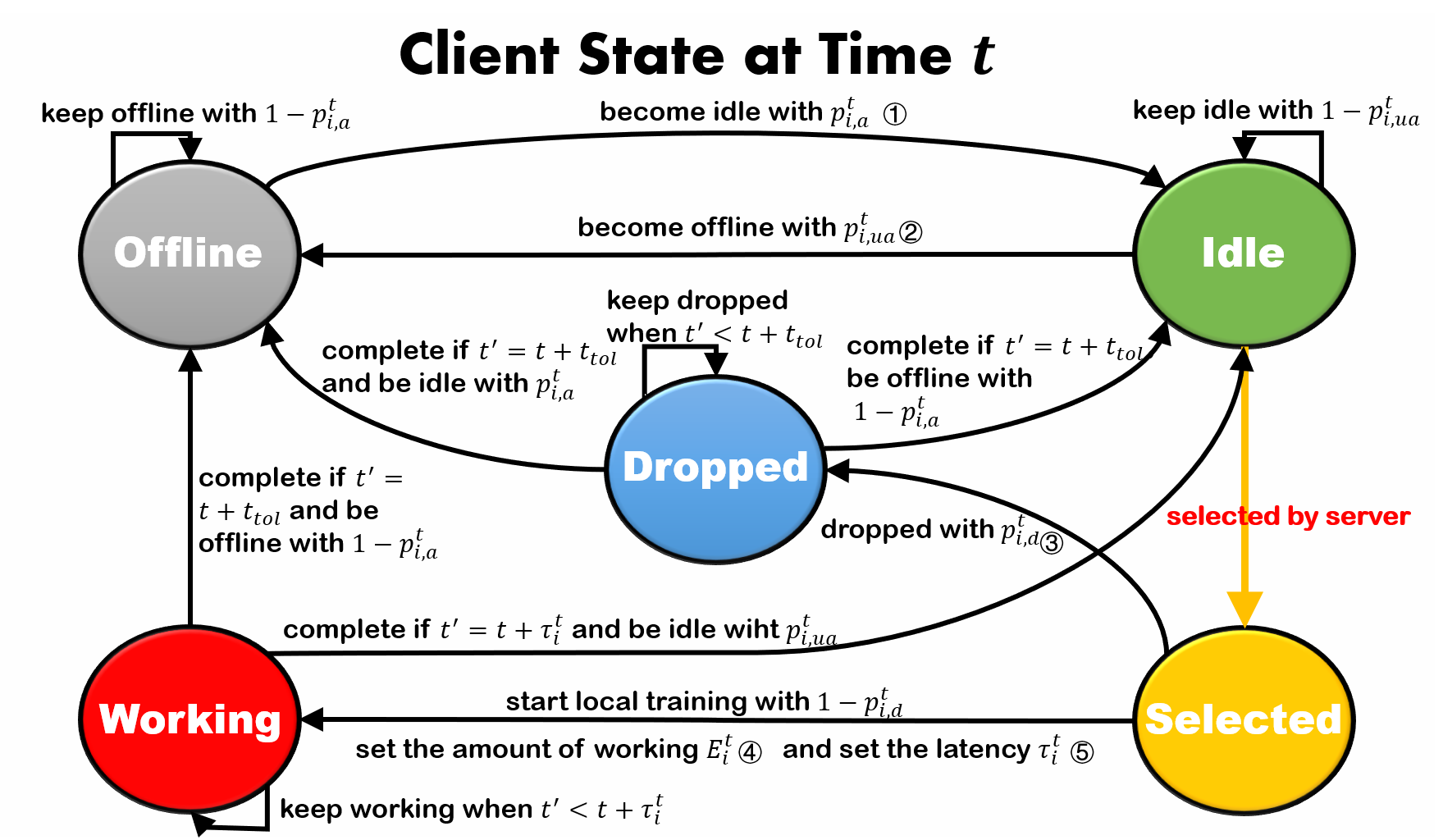
|
||||
We provide simple APIs for users to customize the system heterogeneity for simulation. Please see
|
||||
Tutorial 5.1 for details.
|
|
@ -0,0 +1,114 @@
|
|||
## Algorithm Integration
|
||||
We have already implemented 10+ SOTA algorithms in recent years' top tiers conferences and tiers.
|
||||
|
||||
| Method |Reference| Publication | Tag |
|
||||
|----------|---|----------------|------------------------------------------|
|
||||
| FedAvg |<a href='#refer-anchor-1'>[McMahan et al., 2017]</a>| AISTATS' 2017 ||
|
||||
| FedAsync |<a href='#refer-anchor-2'>[Cong Xie et al., 2019]</a>|| Asynchronous |
|
||||
| FedBuff |<a href='#refer-anchor-3'>[John Nguyen et al., 2022]</a>| AISTATS 2022 | Asynchronous |
|
||||
| TiFL |<a href='#refer-anchor-4'>[Zheng Chai et al., 2020]</a>| HPDC 2020 | Communication-efficiency, responsiveness |
|
||||
| AFL |<a href='#refer-anchor-5'>[Mehryar Mohri et al., 2019]</a>| ICML 2019 | Fairness |
|
||||
| FedFv |<a href='#refer-anchor-6'>[Zheng Wang et al., 2019]</a>| IJCAI 2021 | Fairness |
|
||||
| FedMgda+ |<a href='#refer-anchor-7'>[Zeou Hu et al., 2022]</a>| IEEE TNSE 2022 | Fairness, robustness |
|
||||
| FedProx |<a href='#refer-anchor-8'>[Tian Li et al., 2020]</a>| MLSys 2020 | Non-I.I.D., Incomplete Training |
|
||||
| Mifa |<a href='#refer-anchor-9'>[Xinran Gu et al., 2021]</a>| NeurIPS 2021 | Client Availability |
|
||||
| PowerofChoice |<a href='#refer-anchor-10'>[Yae Jee Cho et al., 2020]</a>| arxiv | Biased Sampling, Fast-Convergence |
|
||||
| QFedAvg |<a href='#refer-anchor-11'>[Tian Li et al., 2020]</a>| ICLR 2020 | Communication-efficient,fairness |
|
||||
| Scaffold |<a href='#refer-anchor-12'>[Sai Praneeth Karimireddy et al., 2020]</a>| ICML 2020 | Non-I.I.D., Communication Capacity |
|
||||
|
||||
|
||||
## Benchmark Gallary
|
||||
| Benchmark |Type| Scene | Task |
|
||||
|----------|---|----------------|------------------------------------------|
|
||||
| CIFAR100 | image| horizontal | classification ||
|
||||
| CIFAR10 | image| horizontal | classification ||
|
||||
| CiteSeer | graph | horizontal | classification ||
|
||||
| Cora | graph | horizontal | classification ||
|
||||
| PubMed | graph | horizontal | classification ||
|
||||
| MNIST | image| horizontal | classification ||
|
||||
| EMNIST | image| horizontal | classification ||
|
||||
| FEMINIST | image| horizontal | classification ||
|
||||
| FashionMINIST | image| horizontal | classification ||
|
||||
| ENZYMES | graph| horizontal | classification ||
|
||||
| Reddit | text | horizontal | classification ||
|
||||
| Sentiment140 | text| horizontal | classification ||
|
||||
| MUTAG | graph | horizontal | classification ||
|
||||
| Shakespeare | text | horizontal | classification ||
|
||||
| Synthetic | table| horizontal | classification ||
|
||||
|
||||
## Async/Sync Supported
|
||||
We set a virtual global clock and a client-state machine to simulate a real-world scenario for comparison on asynchronous
|
||||
and synchronous strategies. Here we provide a comprehensive example to help understand the difference
|
||||
between the two strategies in FLGo.
|
||||
|
||||
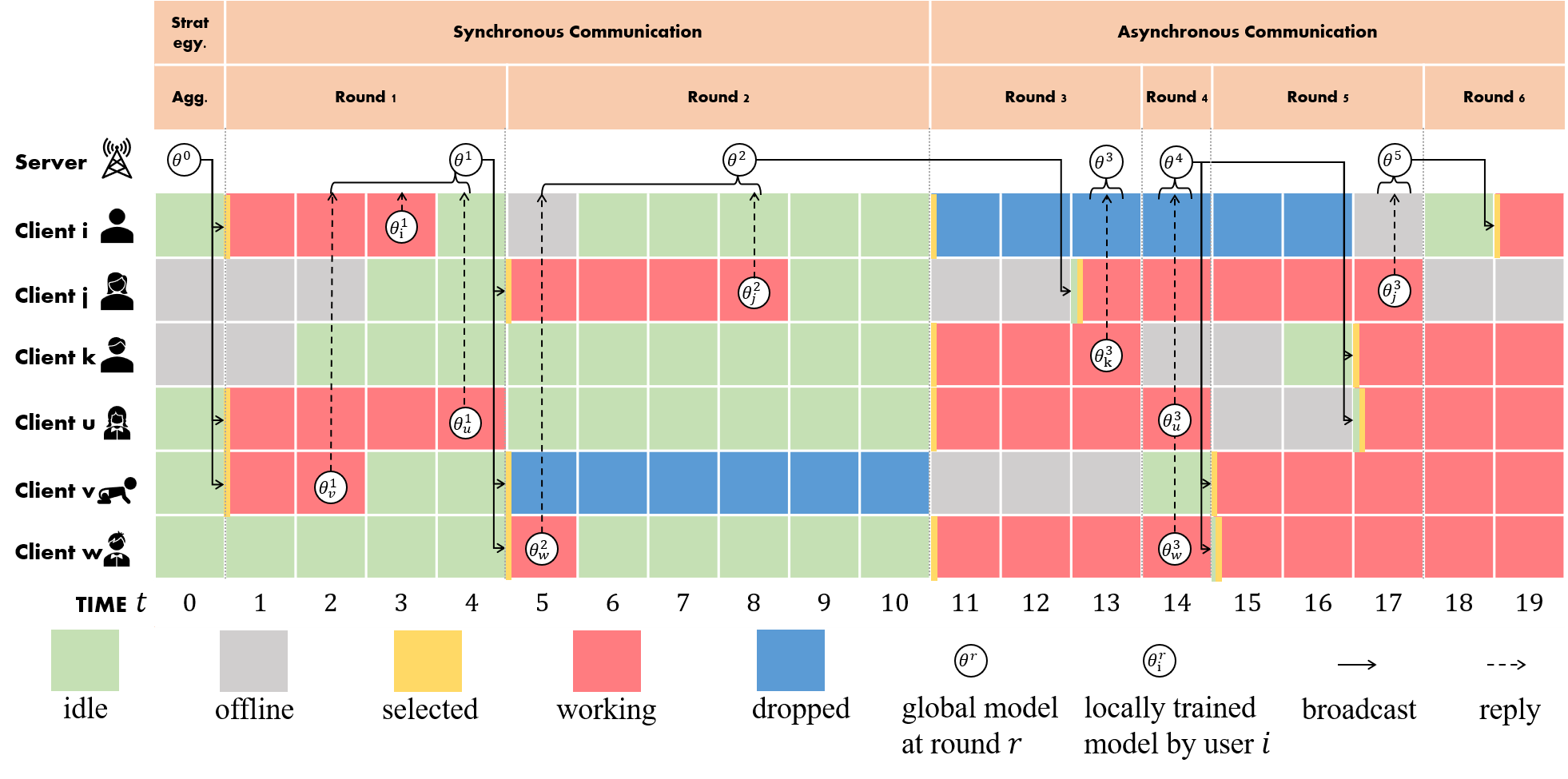
|
||||
For synchronous algorithms, the server would wait for the slowest clients.
|
||||
In round 1,the server select a subset of idle clients (i.e. client i,u,v)
|
||||
to join in training and the slowest client v dominates the duration of this
|
||||
round (i.e. four time units). If there is anyone suffering from
|
||||
training failure (i.e. being dropped out), the duration of the current round
|
||||
should be the longest time that the server will wait for it (e.g. round 2 takes
|
||||
the maximum waiting time of six units to wait for response from client v).
|
||||
|
||||
For asynchronous algorithms, the server usually periodically samples the idle
|
||||
clients to update models, where the length of the period is set as two time
|
||||
units in our example. After sampling the currently idle clients, the server will
|
||||
immediately checks whether there are packages currently returned from clients
|
||||
(e.g. the server selects client j and receives the package from client k at time 13).
|
||||
|
||||
## Experimental Tools
|
||||
For experimental purposes
|
||||
|
||||
## Automatical Tuning
|
||||
|
||||
## Multi-Scene (Horizontal and Vertical)
|
||||
|
||||
## Accelerating by Multi-Process
|
||||
|
||||
|
||||
## References
|
||||
<div id='refer-anchor-1'></div>
|
||||
|
||||
\[McMahan. et al., 2017\] [Brendan McMahan, Eider Moore, Daniel Ramage, Seth Hampson, and Blaise Aguera y Arcas. Communication-Efficient Learning of Deep Networks from Decentralized Data. In International Conference on Artificial Intelligence and Statistics (AISTATS), 2017.](https://arxiv.org/abs/1602.05629)
|
||||
|
||||
<div id='refer-anchor-2'></div>
|
||||
|
||||
\[Cong Xie. et al., 2019\] [Cong Xie, Sanmi Koyejo, Indranil Gupta. Asynchronous Federated Optimization. ](https://arxiv.org/abs/1903.03934)
|
||||
|
||||
<div id='refer-anchor-3'></div>
|
||||
|
||||
\[John Nguyen. et al., 2022\] [John Nguyen, Kshitiz Malik, Hongyuan Zhan, Ashkan Yousefpour, Michael Rabbat, Mani Malek, Dzmitry Huba. Federated Learning with Buffered Asynchronous Aggregation. In International Conference on Artificial Intelligence and Statistics (AISTATS), 2022.](https://arxiv.org/abs/2106.06639)
|
||||
|
||||
<div id='refer-anchor-4'></div>
|
||||
|
||||
\[Zheng Chai. et al., 2020\] [Zheng Chai, Ahsan Ali, Syed Zawad, Stacey Truex, Ali Anwar, Nathalie Baracaldo, Yi Zhou, Heiko Ludwig, Feng Yan, Yue Cheng. TiFL: A Tier-based Federated Learning System.In International Symposium on High-Performance Parallel and Distributed Computing(HPDC), 2020](https://arxiv.org/abs/2106.06639)
|
||||
|
||||
<div id='refer-anchor-5'></div>
|
||||
|
||||
\[Mehryar Mohri. et al., 2019\] [Mehryar Mohri, Gary Sivek, Ananda Theertha Suresh. Agnostic Federated Learning.In International Conference on Machine Learning(ICML), 2019](https://arxiv.org/abs/1902.00146)
|
||||
|
||||
<div id='refer-anchor-6'></div>
|
||||
|
||||
\[Zheng Wang. et al., 2021\] [Zheng Wang, Xiaoliang Fan, Jianzhong Qi, Chenglu Wen, Cheng Wang, Rongshan Yu. Federated Learning with Fair Averaging. In International Joint Conference on Artificial Intelligence, 2021](https://arxiv.org/abs/2104.14937#)
|
||||
|
||||
<div id='refer-anchor-7'></div>
|
||||
|
||||
\[Zeou Hu. et al., 2022\] [Zeou Hu, Kiarash Shaloudegi, Guojun Zhang, Yaoliang Yu. Federated Learning Meets Multi-objective Optimization. In IEEE Transactions on Network Science and Engineering, 2022](https://arxiv.org/abs/2006.11489)
|
||||
|
||||
<div id='refer-anchor-8'></div>
|
||||
|
||||
\[Tian Li. et al., 2020\] [Tian Li, Anit Kumar Sahu, Manzil Zaheer, Maziar Sanjabi, Ameet Talwalkar, Virginia Smith. Federated Optimization in Heterogeneous Networks. In Conference on Machine Learning and Systems, 2020](https://arxiv.org/abs/1812.06127)
|
||||
|
||||
<div id='refer-anchor-9'></div>
|
||||
|
||||
\[Xinran Gu. et al., 2021\] [Xinran Gu, Kaixuan Huang, Jingzhao Zhang, Longbo Huang. Fast Federated Learning in the Presence of Arbitrary Device Unavailability. In Neural Information Processing Systems(NeurIPS), 2021](https://arxiv.org/abs/2106.04159)
|
||||
|
||||
<div id='refer-anchor-10'></div>
|
||||
|
||||
\[Yae Jee Cho. et al., 2020\] [Yae Jee Cho, Jianyu Wang, Gauri Joshi. Client Selection in Federated Learning: Convergence Analysis and Power-of-Choice Selection Strategies. ](https://arxiv.org/abs/2010.01243)
|
||||
|
||||
<div id='refer-anchor-11'></div>
|
||||
|
||||
\[Tian Li. et al., 2020\] [Tian Li, Maziar Sanjabi, Ahmad Beirami, Virginia Smith. Fair Resource Allocation in Federated Learning. In International Conference on Learning Representations, 2020](https://arxiv.org/abs/1905.10497)
|
||||
|
||||
<div id='refer-anchor-12'></div>
|
|
@ -0,0 +1,21 @@
|
|||
# Classical FL
|
||||
| **Name** | **Download** | **Publish** | **Paper Link** | **Remark** |
|
||||
|--------------------|--------------------------------------------------------------------------------------------------------------|---------------------------|------------------------------------------------------------------|------------------------------------------|
|
||||
| FedAvg | [source code](https://raw.githubusercontent.com/WwZzz/easyFL/FLGo/resources/algorithm/fedavg.py) | AISTAS 2017 | [Click](http://arxiv.org/abs/1602.05629) | |
|
||||
| FedProx | [source code](https://raw.githubusercontent.com/WwZzz/easyFL/FLGo/resources/algorithm/fedprox.py) | MLSys 2020 | [Click](http://arxiv.org/abs/1812.06127) | Data Heterogeneity / Incomplete Updates |
|
||||
| FedNova | [source code](https://raw.githubusercontent.com/WwZzz/easyFL/FLGo/resources/algorithm/fednova.py) | NIPS 2020 | [Click](http://arxiv.org/abs/2007.07481) | Incomplete Updates |
|
||||
| Scaffold | [source code](https://raw.githubusercontent.com/WwZzz/easyFL/FLGo/resources/algorithm/scaffold.py) | ICML 2020 | [Click](http://arxiv.org/abs/1910.06378) | Data Heterogeneity/ Client Dropout |
|
||||
| FedDyn | [source code](https://raw.githubusercontent.com/WwZzz/easyFL/FLGo/resources/algorithm/feddyn.py) | ICLR 2021 | [Click](http://arxiv.org/abs/2111.04263) | Data Heterogeneity |
|
||||
| FedAvgM | [source code](https://raw.githubusercontent.com/WwZzz/easyFL/FLGo/resources/algorithm/fedavgm.py) | arxiv 2019 | [Click](http://arxiv.org/abs/1909.06335) | Data Heterogeneity |
|
||||
| AFL | [source code](https://raw.githubusercontent.com/WwZzz/easyFL/FLGo/resources/algorithm/afl.py) | ICML 2019 | [Click](http://arxiv.org/abs/1902.00146) | Fairness |
|
||||
| qFedAvg | [source code](https://raw.githubusercontent.com/WwZzz/easyFL/FLGo/resources/algorithm/qfedavg.py) | ICLR 2020 | [Click](http://arxiv.org/abs/1905.10497) | Fairness |
|
||||
| FedMGDA+ | [source code](https://raw.githubusercontent.com/WwZzz/easyFL/FLGo/resources/algorithm/fedmgda+.py) | IEEE TNSE 2022 | [Click](http://arxiv.org/abs/2006.11489) | Fairness / Robustness |
|
||||
| FedFV | [source code](https://raw.githubusercontent.com/WwZzz/easyFL/FLGo/resources/algorithm/fedfv.py) | IJCAI 2021 | [Click](http://arxiv.org/abs/2104.14937) | Fairness |
|
||||
| FedFa | [source code](https://raw.githubusercontent.com/WwZzz/easyFL/FLGo/resources/algorithm/fedfa.py) | Information Sciences 2022 | [Click](http://arxiv.org/abs/2012.10069) | Fairness |
|
||||
| MIFA | [source code](https://raw.githubusercontent.com/WwZzz/easyFL/FLGo/resources/algorithm/mifa.py) | NIPS 2021 | [Click](http://arxiv.org/abs/2106.04159) | Client Availability |
|
||||
| PowerOfChoice | [source code](https://raw.githubusercontent.com/WwZzz/easyFL/FLGo/resources/algorithm/powerofchoice.py) | arxiv 2021 | [Click](http://arxiv.org/abs/2010.13723) | Data Heterogeneity / Client Availability |
|
||||
| FedGS | [source code](https://raw.githubusercontent.com/WwZzz/easyFL/FLGo/resources/algorithm/fedgs.py) | AAAI 2023 | [Click](https://ojs.aaai.org/index.php/AAAI/article/view/26223 ) | Data Heterogeneity / Client Availability |
|
||||
| Clustered Sampling | [source code](https://raw.githubusercontent.com/WwZzz/easyFL/FLGo/resources/algorithm/clustered_sampling.py) | ICML 2021 | [Click](http://arxiv.org/abs/2105.05883) | Data Heterogeneity |
|
||||
| MOON | [source code](https://raw.githubusercontent.com/WwZzz/easyFL/FLGo/resources/algorithm/moon.py) | | [Click](http://arxiv.org/abs/2103.16257) | Data Heterogeneity |
|
||||
| | | | | |
|
||||
|
|
@ -0,0 +1,45 @@
|
|||
# Usage
|
||||
## Use Algorithm as a Module
|
||||
To use the algorithm, a general way is to create an algorithm file `algorithm_name.py` in the current working project directory, and then copy the source code into it.
|
||||
|
||||
For example, we take next three steps to use FedAvg as a plugin.
|
||||
|
||||
1. Create a new file named 'my_fedavg.py'
|
||||
|
||||
2. Copy the source code of FedAvg [Here](https://raw.githubusercontent.com/WwZzz/easyFL/FLGo/resources/algorithm/fedavg.py) into `my_fedavg.py`
|
||||
|
||||
3. put the file into the currently working project directory and use it by
|
||||
```python
|
||||
import my_fedavg
|
||||
import flgo
|
||||
|
||||
flgo.init(.., algorithm=my_fedavg, ..).run()
|
||||
```
|
||||
## Use Algorithm as a Class
|
||||
Another way to use the algorithm as a plugin is to Create a class instead of a file.
|
||||
|
||||
1. Copy the source code (i.e. the source code is as follows)
|
||||
```python
|
||||
# Example: source code of FedAvg
|
||||
from flgo.algorithm.fedbase import BasicServer as Server
|
||||
from flgo.algorithm..fedbase import BasicClient as Client
|
||||
```
|
||||
|
||||
2. Create a new class like
|
||||
|
||||
```python
|
||||
# Example: source code of FedAvg
|
||||
#---------------codes of FedAvg----------------
|
||||
import flgo
|
||||
from flgo.algorithm.fedbase import BasicServer as Server
|
||||
from flgo.algorithm..fedbase import BasicClient as Client
|
||||
#----------------------end--------------------
|
||||
|
||||
class my_fedavg:
|
||||
# copy the source code here
|
||||
Server = Server
|
||||
Client = Client
|
||||
|
||||
# Run the algorithm
|
||||
flgo.init(.., algorithm=my_fedavg, ..).run()
|
||||
```
|
|
@ -0,0 +1,38 @@
|
|||
# Personalized FL
|
||||
|
||||
To use these algorithms, The term `Logger` should be set as `flgo.experiment.logger.pfl_logger.PFLLogger`. For example,
|
||||
```python
|
||||
import flgo
|
||||
from flgo.experiment.logger.pfl_logger import PFLLogger
|
||||
task = './my_task'
|
||||
# Download codes of ditto and copy it into file 'ditto.py'
|
||||
import ditto
|
||||
runner = flgo.init(task, ditto, {'gpu':[0,],'log_file':True, 'num_steps':5}, Logger=PFLLogger)
|
||||
runner.run()
|
||||
```
|
||||
|
||||
| **Name** | **Download** | **Publish** | **Paper Link** | **Remark** |
|
||||
|-----------------|-----------------------------------------------------------------------------------------------------------|--------------------|------------------------------------------------------------------|--------------------------------|
|
||||
| Standalone | [source code](https://raw.githubusercontent.com/WwZzz/easyFL/FLGo/resources/algorithm/standalone.py) | - | - | Only local training without FL |
|
||||
| FedAvg+FineTune | [source code](https://raw.githubusercontent.com/WwZzz/easyFL/FLGo/resources/algorithm/fedavg_finetune.py) | - | - | |
|
||||
| Ditto | [source code](https://raw.githubusercontent.com/WwZzz/easyFL/FLGo/resources/algorithm/ditto.py) | ICML 2021 | [Click](http://arxiv.org/abs/2007.14390) | |
|
||||
| FedALA | [source code](https://raw.githubusercontent.com/WwZzz/easyFL/FLGo/resources/algorithm/fedala.py) | AAAI 2023 | [Click](http://arxiv.org/abs/2212.01197) | |
|
||||
| FedRep | [source code](https://raw.githubusercontent.com/WwZzz/easyFL/FLGo/resources/algorithm/fedrep.py) | ICML 2021 | [Click](http://arxiv.org/abs/2102.07078) | |
|
||||
| pFedMe | [source code](https://raw.githubusercontent.com/WwZzz/easyFL/FLGo/resources/algorithm/pfedme.py) | NIPS 2020 | [Click](http://arxiv.org/abs/2006.08848) | | |
|
||||
| Per-FedAvg | [source code](https://raw.githubusercontent.com/WwZzz/easyFL/FLGo/resources/algorithm/perfedavg.py) | NIPS 2020 | [Click](http://arxiv.org/abs/2002.07948) | |
|
||||
| FedAMP | [source code](https://raw.githubusercontent.com/WwZzz/easyFL/FLGo/resources/algorithm/fedamp.py) | AAAI 2021 | [Click](http://arxiv.org/abs/2007.03797) | |
|
||||
| FedFomo | [source code](https://raw.githubusercontent.com/WwZzz/easyFL/FLGo/resources/algorithm/fedfomo.py) | ICLR 2021 | [Click](http://arxiv.org/abs/2012.08565) | |
|
||||
| LG-FedAvg | [source code](https://raw.githubusercontent.com/WwZzz/easyFL/FLGo/resources/algorithm/lgfedavg.py) | NIPS 2019 workshop | [Click](http://arxiv.org/abs/2001.01523) | |
|
||||
| pFedHN | [source code](https://raw.githubusercontent.com/WwZzz/easyFL/FLGo/resources/algorithm/pfedhn.py) | ICML 2021 | [Click](https://proceedings.mlr.press/v139/shamsian21a.html) | |
|
||||
| Fed-ROD | [source code](https://raw.githubusercontent.com/WwZzz/easyFL/FLGo/resources/algorithm/fedrod.py) | ICLR 2023 | [Click](https://openreview.net/forum?id=I1hQbx10Kxn) | |
|
||||
| FedPAC | [source code](https://raw.githubusercontent.com/WwZzz/easyFL/FLGo/resources/algorithm/fedpac.py) | ICLR 2023 | [Click](http://arxiv.org/abs/2306.11867) | |
|
||||
| FedPer | [source code](https://raw.githubusercontent.com/WwZzz/easyFL/FLGo/resources/algorithm/fedper.py) | AISTATS 2020 | [Click](http://arxiv.org/abs/1912.00818) | |
|
||||
| APPLE | [source code](https://raw.githubusercontent.com/WwZzz/easyFL/FLGo/resources/algorithm/apple.py) | IJCAI 2022 | [Click](https://www.ijcai.org/proceedings/2022/301) | |
|
||||
| FedBABU | [source code](https://raw.githubusercontent.com/WwZzz/easyFL/FLGo/resources/algorithm/fedbabu.py) | ICLR 2022 | [Click](http://arxiv.org/abs/2106.06042) | |
|
||||
| FedBN | [source code](https://raw.githubusercontent.com/WwZzz/easyFL/FLGo/resources/algorithm/fedbn.py) | ICLR 2021 | [Click](https://openreview.net/pdf?id=6YEQUn0QICG) | |
|
||||
| FedPHP | [source code](https://raw.githubusercontent.com/WwZzz/easyFL/FLGo/resources/algorithm/fedphp.py) | ECML/PKDD 2021 | [Click](https://dl.acm.org/doi/abs/10.1007/978-3-030-86486-6_36) | |
|
||||
| APFL | [source code](https://raw.githubusercontent.com/WwZzz/easyFL/FLGo/resources/algorithm/apfl.py) | 2020 - | [Click](http://arxiv.org/abs/2003.13461) | |
|
||||
| FedProto | [source code](https://raw.githubusercontent.com/WwZzz/easyFL/FLGo/resources/algorithm/fedproto.py) | AAAI 2022 | [Click](https://ojs.aaai.org/index.php/AAAI/article/view/20819) | |
|
||||
| FedCP | [source code](https://raw.githubusercontent.com/WwZzz/easyFL/FLGo/resources/algorithm/fedcp.py) | KDD 2023 | [Click](http://arxiv.org/abs/2307.01217) | |
|
||||
| GPFL | [source code](https://raw.githubusercontent.com/WwZzz/easyFL/FLGo/resources/algorithm/gpfl.py) | ICCV 2023 | [Click](http://arxiv.org/abs/2308.10279) | |
|
||||
| | | | | |
|
|
@ -0,0 +1,37 @@
|
|||
# Overview
|
||||
| **Name** | **Dataset** | **Description** | **Scene** | **Download** | **Remark** |
|
||||
|-------------------------|-------------------------------------------------------------|--------------------------------------|---------------|--------------------------------------------------------------------------------------------------------|------------|
|
||||
| enzymes_graph_classification | [ENZYMES]() | [See here](#enzymes_graph_classification) | Horizontal FL | [Click Here](https://github.com/WwZzz/easyFL/raw/FLGo/resources/benchmark/enzymes_graph_classification.zip) | - |
|
||||
| mutag_graph_classification | [MUTAG]() | [See here](#mutag_graph_classification) | Horizontal FL | [Click Here](https://github.com/WwZzz/easyFL/raw/FLGo/resources/benchmark/mutag_graph_classification.zip) | |
|
||||
|
||||
# Details
|
||||
|
||||
## **enzymes_graph_classification**
|
||||
<div id="enzymes_graph_classification"></div>
|
||||
|
||||
### model
|
||||
| **Model Name** | **Non-Fed Performance** | **NumPara** | **Implementation** |
|
||||
|----------------|-------------------------|-------------|--------------------|
|
||||
| GIN | - | | - |
|
||||
|
||||
### supported partitioner
|
||||
| Name | IsDefault | Comments |
|
||||
|----------------------|-----------|--------------------------------------------------------|
|
||||
| IIDPartitioner | yes | |
|
||||
| DiversityPartitioner | | Partitioning according to label diversity |
|
||||
| DirichletPartitioner | | Partitioning according to dir. distribution of labels |
|
||||
|
||||
## **mutag_graph_classification**
|
||||
<div id="mutag_graph_classification"></div>
|
||||
|
||||
### model
|
||||
| **Model Name** | **Non-Fed Performance** | **NumPara** | **Implementation** |
|
||||
|----------------|-------------------------|-------------|--------------------|
|
||||
| GCN | - | | - |
|
||||
|
||||
### supported partitioner
|
||||
| Name | IsDefault | Comments |
|
||||
|----------------------|-----------|--------------------------------------------------------|
|
||||
| IIDPartitioner | yes | |
|
||||
| DiversityPartitioner | | Partitioning according to label diversity |
|
||||
| DirichletPartitioner | | Partitioning according to dir. distribution of labels |
|
|
@ -0,0 +1,57 @@
|
|||
# Overview
|
||||
| **Name** | **Dataset** | **Description** | **Scene** | **Download** | **Remark** |
|
||||
|-------------------------|---------------------------------------------------------|--------------------------------------|---------------|--------------------------------------------------------------------------------------------------------|------------|
|
||||
| citeseer_link_prediction | [Citeseer](https://www.cs.toronto.edu/~kriz/cifar.html) | [See here](#citeseer_link_prediction) | Horizontal FL | [Click Here](https://github.com/WwZzz/easyFL/raw/FLGo/resources/benchmark/citeseer_link_prediction.zip) | |
|
||||
| cora_link_prediction | [Cora](http://yann.lecun.com/exdb/mnist/) | [See here](#cora_link_prediction) | Horizontal FL | [Click Here](https://github.com/WwZzz/easyFL/raw/FLGo/resources/benchmark/cora_link_prediction.zip) | - |
|
||||
| pubmed_link_prediction | [PubMed](https://www.cs.toronto.edu/~kriz/cifar.html) | [See here](#pubmed_link_prediction) | Horizontal FL | [Click Here](https://github.com/WwZzz/easyFL/raw/FLGo/resources/benchmark/pubmed_link_prediction.zip) | |
|
||||
|
||||
# Details
|
||||
## **citeseer_link_prediction**
|
||||
<div id="citeseer_link_prediction"></div>
|
||||
|
||||
### model
|
||||
| **Model Name** | **Non-Fed Performance** | **NumPara** | **Implementation** |
|
||||
|----------------|-------------------------|-------------|--------------------|
|
||||
| cnn | - | | - |
|
||||
| mlp | - | | - |
|
||||
|
||||
### supported partitioner
|
||||
| Name | IsDefault | Comments |
|
||||
|----------------------|-----------|--------------------------------------------------------|
|
||||
| IIDPartitioner | yes | |
|
||||
| DiversityPartitioner | | Partitioning according to label diversity |
|
||||
| DirichletPartitioner | | Partitioning according to dir. distribution of labels |
|
||||
|
||||
## **cora_link_prediction**
|
||||
<div id="cora_link_prediction"></div>
|
||||
|
||||
### model
|
||||
| **Model Name** | **Non-Fed Performance** | **NumPara** | **Implementation** |
|
||||
|----------------|-------------------------|-------------|--------------------|
|
||||
| cnn | - | | - |
|
||||
| mlp | - | | - |
|
||||
|
||||
### supported partitioner
|
||||
| Name | IsDefault | Comments |
|
||||
|----------------------|-----------|--------------------------------------------------------|
|
||||
| IIDPartitioner | yes | |
|
||||
| DiversityPartitioner | | Partitioning according to label diversity |
|
||||
| DirichletPartitioner | | Partitioning according to dir. distribution of labels |
|
||||
|
||||
## **pubmed_link_prediction**
|
||||
<div id="pubmed_link_prediction"></div>
|
||||
|
||||
### model
|
||||
| **Model Name** | **Non-Fed Performance** | **NumPara** | **Implementation** |
|
||||
|----------------|-------------------------|-------------|--------------------|
|
||||
| cnn | - | | - |
|
||||
| mlp | - | | - |
|
||||
|
||||
### supported partitioner
|
||||
| Name | IsDefault | Comments |
|
||||
|----------------------|-----------|--------------------------------------------------------|
|
||||
| IIDPartitioner | yes | |
|
||||
| DiversityPartitioner | | Partitioning according to label diversity |
|
||||
| DirichletPartitioner | | Partitioning according to dir. distribution of labels |
|
||||
|
||||
|
|
@ -0,0 +1,60 @@
|
|||
# Overview
|
||||
| **Name** | **Dataset** | **Description** | **Scene** | **Download** | **Remark** |
|
||||
|-------------------------|---------------------------------------------------------|--------------------------------------|---------------|--------------------------------------------------------------------------------------------------------|------------|
|
||||
| citeseer_node_classification | [Citeseer](https://www.cs.toronto.edu/~kriz/cifar.html) | [See here](#citeseer_node_classification) | Horizontal FL | [Click Here](https://github.com/WwZzz/easyFL/raw/FLGo/resources/benchmark/citeseer_node_classification.zip) | |
|
||||
| cora_node_classification | [Cora](http://yann.lecun.com/exdb/mnist/) | [See here](#cora_node_classification) | Horizontal FL | [Click Here](https://github.com/WwZzz/easyFL/raw/FLGo/resources/benchmark/cora_node_classification.zip) | - |
|
||||
| pubmed_node_classification | [PubMed](https://www.cs.toronto.edu/~kriz/cifar.html) | [See here](#pubmed_node_classification) | Horizontal FL | [Click Here](https://github.com/WwZzz/easyFL/raw/FLGo/resources/benchmark/pubmed_node_classification.zip) | |
|
||||
|
||||
# Details
|
||||
## **citeseer_node_classification**
|
||||
<div id="citeseer_node_classification"></div>
|
||||
Federated CIFAR100 classification is a commonly used benchmark in FL. It assumes different virtual clients having non-overlapping samples from CIFAR100 dataset.
|
||||
|
||||
### model
|
||||
| **Model Name** | **Non-Fed Performance** | **NumPara** | **Implementation** |
|
||||
|----------------|-------------------------|-------------|--------------------|
|
||||
| cnn | - | | - |
|
||||
| mlp | - | | - |
|
||||
|
||||
### supported partitioner
|
||||
| Name | IsDefault | Comments |
|
||||
|----------------------|-----------|--------------------------------------------------------|
|
||||
| IIDPartitioner | yes | |
|
||||
| DiversityPartitioner | | Partitioning according to label diversity |
|
||||
| DirichletPartitioner | | Partitioning according to dir. distribution of labels |
|
||||
|
||||
## **cora_node_classification**
|
||||
<div id="cora_node_classification"></div>
|
||||
Federated MNIST classification is a commonly used benchmark in FL. It assumes different virtual clients having non-overlapping samples from MNIST dataset.
|
||||
|
||||
### model
|
||||
| **Model Name** | **Non-Fed Performance** | **NumPara** | **Implementation** |
|
||||
|----------------|-------------------------|-------------|--------------------|
|
||||
| cnn | - | | - |
|
||||
| mlp | - | | - |
|
||||
|
||||
### supported partitioner
|
||||
| Name | IsDefault | Comments |
|
||||
|----------------------|-----------|--------------------------------------------------------|
|
||||
| IIDPartitioner | yes | |
|
||||
| DiversityPartitioner | | Partitioning according to label diversity |
|
||||
| DirichletPartitioner | | Partitioning according to dir. distribution of labels |
|
||||
|
||||
## **pubmed_node_classification**
|
||||
<div id="pubmed_node_classification"></div>
|
||||
Federated CIFAR10 classification is a commonly used benchmark in FL. It assumes different virtual clients having non-overlapping samples from CIFAR10 dataset.
|
||||
|
||||
### model
|
||||
| **Model Name** | **Non-Fed Performance** | **NumPara** | **Implementation** |
|
||||
|----------------|-------------------------|-------------|--------------------|
|
||||
| cnn | - | | - |
|
||||
| mlp | - | | - |
|
||||
|
||||
### supported partitioner
|
||||
| Name | IsDefault | Comments |
|
||||
|----------------------|-----------|--------------------------------------------------------|
|
||||
| IIDPartitioner | yes | |
|
||||
| DiversityPartitioner | | Partitioning according to label diversity |
|
||||
| DirichletPartitioner | | Partitioning according to dir. distribution of labels |
|
||||
|
||||
|
|
@ -0,0 +1,163 @@
|
|||
# Overview
|
||||
| **Name** | **Dataset** | **Description** | **Scene** | **Download** | **Remark** |
|
||||
|---------------------------------|--------------------------------------------------------------------------------|----------------------------------------------|----------------|----------------------------------------------------------------------------------------------------------------|--------------|
|
||||
| mnist_classification | [MNIST](http://yann.lecun.com/exdb/mnist/) | [See here](#mnist_classification) | Horizontal FL | [Click Here](https://github.com/WwZzz/easyFL/raw/FLGo/resources/benchmark/mnist_classification.zip) | - |
|
||||
| cifar10_classification | [CIFAR10](https://www.cs.toronto.edu/~kriz/cifar.html) | [See here](#cifar10_classification) | Horizontal FL | [Click Here](https://github.com/WwZzz/easyFL/raw/FLGo/resources/benchmark/cifar10_classification.zip) | |
|
||||
| cifar100_classification | [CIFAR100](https://www.cs.toronto.edu/~kriz/cifar.html) | [See here](#cifar100_classification) | Horizontal FL | [Click Here](https://github.com/WwZzz/easyFL/raw/FLGo/resources/benchmark/cifar100_classification.zip) | |
|
||||
| svhn_classification | [SVHN](http://ufldl.stanford.edu/housenumbers/) | [See here](#svhn_classification) | Horizontal FL | [Click Here](https://github.com/WwZzz/easyFL/raw/FLGo/resources/benchmark/svhn_classification.zip) | - |
|
||||
| fashion_classification | [FASHION](https://github.com/zalandoresearch/fashion-mnist) | [See here](#fashion_classification) | Horizontal FL | [Click Here](https://github.com/WwZzz/easyFL/raw/FLGo/resources/benchmark/fashion_classification.zip) | - |
|
||||
| domainnet_classification | [DomainNet](https://ai.bu.edu/M3SDA) | [See here](#domainnet_classification) | Horizontal FL | [Click Here](https://github.com/WwZzz/easyFL/raw/FLGo/resources/benchmark/domainnet_classification.zip) | Feature Skew |
|
||||
| office-caltech10_classification | [OfficeCaltech10](https://github.com/ChristophRaab/Office_Caltech_DA_Dataset/) | [See here](#office-caltech10_classification) | Horizontal FL | [Click Here](https://github.com/WwZzz/easyFL/raw/FLGo/resources/benchmark/office-caltech10_classification.zip) | Feature Skew |
|
||||
| pacs_classification | [PACS](https://github.com/MachineLearning2020/Homework3-PACS/tree/master) | [See here](#pacs_classification) | Horizontal FL | [Click Here](https://github.com/WwZzz/easyFL/raw/FLGo/resources/benchmark/pacs_classification.zip) | Feature Skew |
|
||||
|
||||
# Details
|
||||
|
||||
## **mnist_classification**
|
||||
<div id="mnist_classification"></div>
|
||||
|
||||

|
||||
|
||||
Federated MNIST classification is a commonly used benchmark in FL. It assumes different virtual clients having non-overlapping samples from MNIST dataset.
|
||||
|
||||
### model
|
||||
| **Model Name** | **Non-Fed Performance** | **NumPara** | **Implementation** |
|
||||
|----------------|-------------------------|-------------|--------------------|
|
||||
| cnn | - | | - |
|
||||
| mlp | - | | - |
|
||||
|
||||
### supported partitioner
|
||||
| Name | IsDefault | Comments |
|
||||
|----------------------|-----------|--------------------------------------------------------|
|
||||
| IIDPartitioner | yes | |
|
||||
| DiversityPartitioner | | Partitioning according to label diversity |
|
||||
| DirichletPartitioner | | Partitioning according to dir. distribution of labels |
|
||||
|
||||
## **cifar10_classification**
|
||||
<div id="cifar10_classification"></div>
|
||||
|
||||

|
||||
|
||||
Federated CIFAR10 classification is a commonly used benchmark in FL. It assumes different virtual clients having non-overlapping samples from CIFAR10 dataset.
|
||||
|
||||
### model
|
||||
| **Model Name** | **Non-Fed Performance** | **NumPara** | **Implementation** |
|
||||
|----------------|-------------------------|-------------|--------------------|
|
||||
| cnn | - | | - |
|
||||
| mlp | - | | - |
|
||||
|
||||
### supported partitioner
|
||||
| Name | IsDefault | Comments |
|
||||
|----------------------|-----------|--------------------------------------------------------|
|
||||
| IIDPartitioner | yes | |
|
||||
| DiversityPartitioner | | Partitioning according to label diversity |
|
||||
| DirichletPartitioner | | Partitioning according to dir. distribution of labels |
|
||||
|
||||
## **cifar100_classification**
|
||||
<div id="cifar100_classification"></div>
|
||||
Federated CIFAR100 classification is a commonly used benchmark in FL. It assumes different virtual clients having non-overlapping samples from CIFAR100 dataset.
|
||||
|
||||
### model
|
||||
| **Model Name** | **Non-Fed Performance** | **NumPara** | **Implementation** |
|
||||
|----------------|-------------------------|-------------|--------------------|
|
||||
| cnn | - | | - |
|
||||
| mlp | - | | - |
|
||||
|
||||
### supported partitioner
|
||||
| Name | IsDefault | Comments |
|
||||
|----------------------|-----------|--------------------------------------------------------|
|
||||
| IIDPartitioner | yes | |
|
||||
| DiversityPartitioner | | Partitioning according to label diversity |
|
||||
| DirichletPartitioner | | Partitioning according to dir. distribution of labels |
|
||||
|
||||
## **svhn_classification**
|
||||
<div id="svhn_classification"></div>
|
||||
Federated SVHN classification is a commonly used benchmark in FL. It assumes different virtual clients having non-overlapping samples from SVHN dataset.
|
||||
|
||||
### model
|
||||
| **Model Name** | **Non-Fed Performance** | **NumPara** | **Implementation** |
|
||||
|----------------|-------------------------|-------------|--------------------|
|
||||
| cnn | - | | - |
|
||||
| mlp | - | | - |
|
||||
|
||||
### supported partitioner
|
||||
| Name | IsDefault | Comments |
|
||||
|----------------------|-----------|--------------------------------------------------------|
|
||||
| IIDPartitioner | yes | |
|
||||
| DiversityPartitioner | | Partitioning according to label diversity |
|
||||
| DirichletPartitioner | | Partitioning according to dir. distribution of labels |
|
||||
|
||||
## **fashion_classification**
|
||||
<div id="fashion_classification"></div>
|
||||
Federated Fashion classification is a commonly used benchmark in FL. It assumes different virtual clients having non-overlapping samples from FashionMNIST dataset.
|
||||
|
||||
### model
|
||||
| **Model Name** | **Non-Fed Performance** | **NumPara** | **Implementation** |
|
||||
|----------------|-------------------------|-------------|--------------------|
|
||||
| lr | - | | - |
|
||||
|
||||
### supported partitioner
|
||||
| Name | IsDefault | Comments |
|
||||
|----------------------|-----------|--------------------------------------------------------|
|
||||
| IIDPartitioner | yes | |
|
||||
| DiversityPartitioner | | Partitioning according to label diversity |
|
||||
| DirichletPartitioner | | Partitioning according to dir. distribution of labels |
|
||||
|
||||
## **domainnet_classification**
|
||||
<div id="domainnet_classification"></div>
|
||||
|
||||
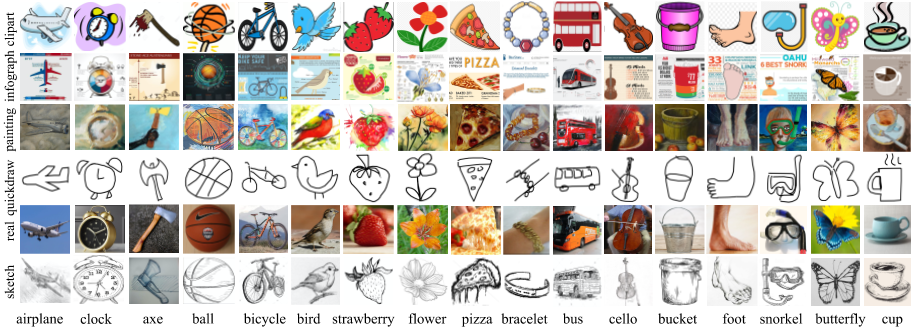
|
||||
|
||||
DomainNet contains images of the same labels but different styles (i.e. 6 styles), which can be used to investigate the influence of feature skew in FL.
|
||||
The paper is available at [link](https://arxiv.org/pdf/1812.01754.pdf)
|
||||
|
||||
### model
|
||||
| **Model Name** | **Non-Fed Performance** | **NumPara** | **Implementation** |
|
||||
|----------------|-------------------------|-------------|--------------------|
|
||||
| AlexNet | - | | - |
|
||||
| resnet18 | | | |
|
||||
|
||||
### supported partitioner
|
||||
| Name | IsDefault | Comments |
|
||||
|----------------------|-----------|--------------------------------------------------------|
|
||||
| IIDPartitioner | yes | |
|
||||
| DiversityPartitioner | | Partitioning according to label diversity |
|
||||
| DirichletPartitioner | | Partitioning according to dir. distribution of labels |
|
||||
|
||||
## **office-caltech10_classification**
|
||||
<div id="office-caltech10_classification"></div>
|
||||
|
||||

|
||||
|
||||
Office-Caltech-10 a standard benchmark for domain adaptation, which consists of Office 10 and Caltech 10 datasets. It contains the 10 overlapping categories between the Office dataset and Caltech256 dataset. SURF BoW historgram features, vector quantized to 800 dimensions are also available for this dataset.
|
||||
### model
|
||||
| **Model Name** | **Non-Fed Performance** | **NumPara** | **Implementation** |
|
||||
|----------------|-------------------------|-------------|--------------------|
|
||||
| AlexNet | - | | - |
|
||||
| resnet18 | | | |
|
||||
|
||||
### supported partitioner
|
||||
| Name | IsDefault | Comments |
|
||||
|----------------------|-----------|--------------------------------------------------------|
|
||||
| IIDPartitioner | yes | |
|
||||
| DiversityPartitioner | | |
|
||||
| DirichletPartitioner | | |
|
||||
|
||||
## **pacs_classification**
|
||||
<div id="pacs_classification"></div>
|
||||
|
||||
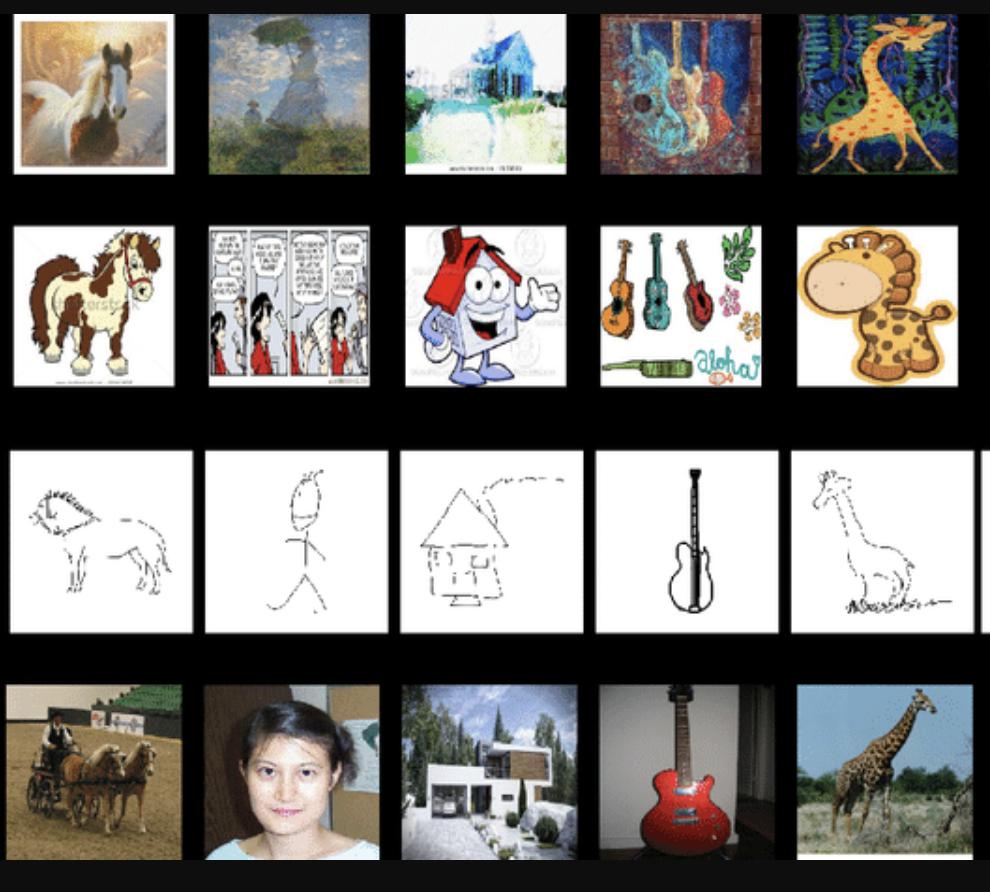
|
||||
PACS is an image dataset for domain generalization. It consists of four domains, namely Photo (1,670 images), Art Painting (2,048 images), Cartoon (2,344 images) and Sketch (3,929 images). Each domain contains seven categories.
|
||||
### model
|
||||
| **Model Name** | **Non-Fed Performance** | **NumPara** | **Implementation** |
|
||||
|----------------|-------------------------|-------------|--------------------|
|
||||
| AlexNet | - | | - |
|
||||
| resnet18 | | | |
|
||||
|
||||
### supported partitioner
|
||||
| Name | IsDefault | Comments |
|
||||
|----------------------|-----------|--------------------------------------------------------|
|
||||
| IIDPartitioner | yes | |
|
||||
| DiversityPartitioner | | |
|
||||
| DirichletPartitioner | | |
|
||||
|
||||
|
|
@ -0,0 +1,39 @@
|
|||
# Overview
|
||||
| **Name** | **Dataset** | **Description** | **Scene** | **Download** | **Remark** |
|
||||
|-------------------------|-------------------------------------------------------------|--------------------------------------|---------------|-------------------------------------------------------------------------------------------------------|-----------------|
|
||||
| coco_detection | [COCO](https://cocodataset.org/#detection-2016) | [See here](#coco_detection) | Horizontal FL | [Click Here](https://github.com/WwZzz/easyFL/raw/FLGo/resources/benchmark/coco_detection.zip) | (under testing) |
|
||||
| voc_detection | [VOC](http://host.robots.ox.ac.uk/pascal/VOC/) | [See here](#voc_detection) | Horizontal FL | [Click Here](https://github.com/WwZzz/easyFL/raw/FLGo/resources/benchmark/voc_detection.zip) | |
|
||||
|
||||
# Details
|
||||
|
||||
## **coco_detection**
|
||||
<div id="coco_detection"></div>
|
||||
coco
|
||||
|
||||
### model
|
||||
| **Model Name** | **Non-Fed Performance** | **NumPara** | **Implementation** |
|
||||
|----------------|-------------------------|-------------|--------------------|
|
||||
| FasterRCNN | - | | - |
|
||||
|
||||
### supported partitioner
|
||||
| Name | IsDefault | Comments |
|
||||
|----------------------|-----------|--------------------------------------------------------|
|
||||
| IIDPartitioner | yes | |
|
||||
| DiversityPartitioner | | Partitioning according to label diversity |
|
||||
| DirichletPartitioner | | Partitioning according to dir. distribution of labels |
|
||||
|
||||
## **voc_detection**
|
||||
<div id="voc_detection"></div>
|
||||
coco
|
||||
|
||||
### model
|
||||
| **Model Name** | **Non-Fed Performance** | **NumPara** | **Implementation** |
|
||||
|----------------|-------------------------|-------------|--------------------|
|
||||
| FasterRCNN | - | | - |
|
||||
|
||||
### supported partitioner
|
||||
| Name | IsDefault | Comments |
|
||||
|----------------------|-----------|--------------------------------------------------------|
|
||||
| IIDPartitioner | yes | |
|
||||
| DiversityPartitioner | | Partitioning according to label diversity |
|
||||
| DirichletPartitioner | | Partitioning according to dir. distribution of labels |
|
|
@ -0,0 +1,5 @@
|
|||
# Classification
|
||||
|
||||
# Detection
|
||||
|
||||
# Segmentation
|
|
@ -0,0 +1,148 @@
|
|||
# Overview
|
||||
| **Name** | **Dataset** | **Description** | **Scene** | **Download** | **Remark** |
|
||||
|----------------------------|---------------------------------------------------------------------|-----------------------------------------|---------------|-----------------------------------------------------------------------------------------------------------|-----------------|
|
||||
| coco_segmentation | [COCO](https://cocodataset.org/#detection-2016) | [See here](#coco_segmentation) | Horizontal FL | [Click Here](https://github.com/WwZzz/easyFL/raw/FLGo/resources/benchmark/coco_segmentation.zip) | (under testing) |
|
||||
| oxfordiiitpet_segmentation | [OxfordIIITPet](https://www.robots.ox.ac.uk/~vgg/data/pets/) | [See here](#oxfordiiitpet_segmentation) | Horizontal FL | [Click Here](https://github.com/WwZzz/easyFL/raw/FLGo/resources/benchmark/oxfordiiitpet_segmentation.zip) | |
|
||||
| sbdataset_segmentation | [SBDataset](http://home.bharathh.info/pubs/codes/SBD/download.html) | [See here](#sbdataset_segmentation) | Horizontal FL | [Click Here](https://github.com/WwZzz/easyFL/raw/FLGo/resources/benchmark/sbdataset_segmentation.zip) | |
|
||||
| cityspaces_segmentation | [Cityspaces](https://www.cityscapes-dataset.com/) | [See here](#cityspaces_segmentation) | Horizontal FL | [Click Here](https://github.com/WwZzz/easyFL/raw/FLGo/resources/benchmark/cityspaces_segmentation.zip) | |
|
||||
| camvid_segmentation | [CamVID](https://www.kaggle.com/datasets/carlolepelaars/camvid) | [See here](#camvid_segmentation) | Horizontal FL | [Click Here](https://github.com/WwZzz/easyFL/raw/FLGo/resources/benchmark/camvid_segmentation.zip) | |
|
||||
| ade20k_segmentation | [ADE20k](http://sceneparsing.csail.mit.edu/ ) | [See here](#ade20k_segmentation) | Horizontal FL | [Click Here](https://github.com/WwZzz/easyFL/raw/FLGo/resources/benchmark/ade20k_segmentation.zip) | |
|
||||
| voc_segmentation | [PASCAL_VOC](http://host.robots.ox.ac.uk/pascal/VOC/ ) | [See here](#voc_segmentation) | Horizontal FL | [Click Here](https://github.com/WwZzz/easyFL/raw/FLGo/resources/benchmark/voc_segmentation.zip) | |
|
||||
|
||||
|
||||
# Details
|
||||
|
||||
## **coco_segmentation**
|
||||
<div id="coco_segmentation"></div>
|
||||
coco
|
||||
|
||||
### model
|
||||
| **Model Name** | **Non-Fed Performance** | **NumPara** | **Implementation** |
|
||||
|----------------|-------------------------|-------------|--------------------|
|
||||
| FCN_ResNet50 | - | | - |
|
||||
| UNet | - | | - |
|
||||
|
||||
### supported partitioner
|
||||
| Name | IsDefault | Comments |
|
||||
|----------------------|-----------|--------------------------------------------------------|
|
||||
| IIDPartitioner | yes | |
|
||||
| DiversityPartitioner | | Partitioning according to label diversity |
|
||||
| DirichletPartitioner | | Partitioning according to dir. distribution of labels |
|
||||
|
||||
## **oxfordiiitpet_segmentation**
|
||||
<div id="oxfordiiitpet_segmentation"></div>
|
||||
coco
|
||||
|
||||
### model
|
||||
| **Model Name** | **Non-Fed Performance** | **NumPara** | **Implementation** |
|
||||
|----------------|-------------------------|-------------|--------------------|
|
||||
| FCN_ResNet50 | - | | - |
|
||||
|
||||
### supported partitioner
|
||||
| Name | IsDefault | Comments |
|
||||
|----------------------|-----------|--------------------------------------------------------|
|
||||
| IIDPartitioner | yes | |
|
||||
| DiversityPartitioner | | Partitioning according to label diversity |
|
||||
| DirichletPartitioner | | Partitioning according to dir. distribution of labels |
|
||||
|
||||
## **sbdataset_segmentation**
|
||||
<div id="sbdataset_segmentation"></div>
|
||||
coco
|
||||
|
||||
### model
|
||||
| **Model Name** | **Non-Fed Performance** | **NumPara** | **Implementation** |
|
||||
|----------------|-------------------------|-------------|--------------------|
|
||||
| FCN_ResNet50 | - | | - |
|
||||
| UNet | - | | - |
|
||||
|
||||
### supported partitioner
|
||||
| Name | IsDefault | Comments |
|
||||
|----------------------|-----------|--------------------------------------------------------|
|
||||
| IIDPartitioner | yes | |
|
||||
| DiversityPartitioner | | Partitioning according to label diversity |
|
||||
| DirichletPartitioner | | Partitioning according to dir. distribution of labels |
|
||||
|
||||
## **cityspaces_segmentation**
|
||||
<div id="cityspaces_segmentation"></div>
|
||||
|
||||
### Usage
|
||||
To use this benchmark, you need to manually download the raw data into the dictionary 'cityspaces_segmentation/cityspaces/'. The necessary file contains
|
||||
`leftImg8bit_trainvaltest.zip` (11GB) and `gtFine_trainvaltest.zip` (241MB).
|
||||
|
||||
### model
|
||||
| **Model Name** | **Non-Fed Performance** | **NumPara** | **Implementation** |
|
||||
|----------------|-------------------------|-------------|--------------------|
|
||||
| FCN_ResNet50 | - | | - |
|
||||
|
||||
### supported partitioner
|
||||
| Name | IsDefault | Comments |
|
||||
|----------------------|-----------|--------------------------------------------------------|
|
||||
| IIDPartitioner | yes | |
|
||||
|
||||
## **camvid_segmentation**
|
||||
<div id="camvid_segmentation"></div>
|
||||
|
||||
### Usage
|
||||
To use this benchmark, you need to manually download the raw data into the dictionary 'camvid_segmentation/CamVid/' from [Kaggle](https://www.kaggle.com/datasets/carlolepelaars/camvid).
|
||||
The downloaded .zip file should also be manually into 'camvid_segmentation/CamVid/'. The architecture of the benchmark should be like:
|
||||
```
|
||||
├─ camvid_segmentation
|
||||
│ ├─ CamVid //classification on mnist dataset
|
||||
│ │ ├─ train
|
||||
│ | │ ├─ xxx.png // horizontal fedtask
|
||||
│ | │ ...
|
||||
│ │ ├─ train_labels
|
||||
│ | │ ├─ xxx.png
|
||||
│ | │ ...
|
||||
│ │ ├─ val
|
||||
│ │ ├─ val_labels
|
||||
│ │ ├─ test
|
||||
│ │ ├─ test_labels
|
||||
│ │ └─ class_dict.csv
|
||||
│ |
|
||||
│ ├─ model
|
||||
│ ├─ config.py
|
||||
│ ├─ core.py
|
||||
│ └─ __init__.py
|
||||
```
|
||||
|
||||
### model
|
||||
| **Model Name** | **Non-Fed Performance** | **NumPara** | **Implementation** |
|
||||
|----------------|-------------------------|-------------|--------------------|
|
||||
| FCN_ResNet50 | - | | - |
|
||||
|
||||
### supported partitioner
|
||||
| Name | IsDefault | Comments |
|
||||
|----------------------|-----------|--------------------------------------------------------|
|
||||
| IIDPartitioner | yes | |
|
||||
|
||||
|
||||
## **ade20k_segmentation**
|
||||
<div id="ade20k_segmentation"></div>
|
||||
ADE20K
|
||||
|
||||
### model
|
||||
| **Model Name** | **Non-Fed Performance** | **NumPara** | **Implementation** |
|
||||
|----------------|-------------------------|-------------|--------------------|
|
||||
| FCN_ResNet50 | - | | - |
|
||||
|
||||
### supported partitioner
|
||||
| Name | IsDefault | Comments |
|
||||
|----------------------|-----------|--------------------------------------------------------|
|
||||
| IIDPartitioner | yes | |
|
||||
| DiversityPartitioner | | Partitioning according to label diversity |
|
||||
| DirichletPartitioner | | Partitioning according to dir. distribution of labels |
|
||||
|
||||
## **voc_segmentation**
|
||||
<div id="voc_segmentation"></div>
|
||||
VOC
|
||||
|
||||
### model
|
||||
| **Model Name** | **Non-Fed Performance** | **NumPara** | **Implementation** |
|
||||
|----------------|-------------------------|-------------|--------------------|
|
||||
| FCN_ResNet50 | - | | - |
|
||||
|
||||
### supported partitioner
|
||||
| Name | IsDefault | Comments |
|
||||
|----------------------|-----------|--------------------------------------------------------|
|
||||
| IIDPartitioner | yes | |
|
|
@ -0,0 +1,87 @@
|
|||
# Overview
|
||||
| **Name** | **Dataset** | **Description** | **Scene** | **Download** | **Remark** |
|
||||
|-------------------------|-----------------------------------------------------|--------------------------------------|---------------|--------------------------------------------------------------------------------------------------------|------------|
|
||||
| agnews_classification | [AGNEWS](http://yann.lecun.com/exdb/mnist/) | [See here](#agnews_classification) | Horizontal FL | [Click Here](https://github.com/WwZzz/easyFL/raw/FLGo/resources/benchmark/agnews_classification.zip) | - |
|
||||
| imdb_classification | [IMDB](https://www.cs.toronto.edu/~kriz/cifar.html) | [See here](#imdb_classification) | Horizontal FL | [Click Here](https://github.com/WwZzz/easyFL/raw/FLGo/resources/benchmark/imdb_classification.zip) | |
|
||||
| sst2_classification | [SST2](https://www.cs.toronto.edu/~kriz/cifar.html) | [See here](#sst2_classification) | Horizontal FL | [Click Here](https://github.com/WwZzz/easyFL/raw/FLGo/resources/benchmark/sst2_classification.zip) | |
|
||||
|
||||
# Details
|
||||
|
||||
## **agnews_classification**
|
||||
<div id="agnews_classification"></div>
|
||||
|
||||
### model
|
||||
| **Model Name** | **Non-Fed Performance** | **NumPara** | **Implementation** |
|
||||
|----------------|-------------------------|-------------|--------------------|
|
||||
| TextClassificationModel | - | | - |
|
||||
|
||||
### supported partitioner
|
||||
| Name | IsDefault | Comments |
|
||||
|----------------------|-----------|--------------------------------------------------------|
|
||||
| IIDPartitioner | yes | |
|
||||
| DiversityPartitioner | | Partitioning according to label diversity |
|
||||
| DirichletPartitioner | | Partitioning according to dir. distribution of labels |
|
||||
|
||||
## **imdb_classification**
|
||||
<div id="imdb_classification"></div>
|
||||
-
|
||||
### model
|
||||
| **Model Name** | **Non-Fed Performance** | **NumPara** | **Implementation** |
|
||||
|----------------|-------------------------|-------------|--------------------|
|
||||
| TextClassificationModel | - | | - |
|
||||
|
||||
|
||||
|
||||
### supported partitioner
|
||||
| Name | IsDefault | Comments |
|
||||
|----------------------|-----------|--------------------------------------------------------|
|
||||
| IIDPartitioner | yes | |
|
||||
| DiversityPartitioner | | Partitioning according to label diversity |
|
||||
| DirichletPartitioner | | Partitioning according to dir. distribution of labels |
|
||||
|
||||
## **sst2_classification**
|
||||
<div id="sst2_classification"></div>
|
||||
-
|
||||
### model
|
||||
| **Model Name** | **Non-Fed Performance** | **NumPara** | **Implementation** |
|
||||
|----------------|-------------------------|-------------|--------------------|
|
||||
| TextClassificationModel | - | | - |
|
||||
|
||||
|
||||
### supported partitioner
|
||||
| Name | IsDefault | Comments |
|
||||
|----------------------|-----------|--------------------------------------------------------|
|
||||
| IIDPartitioner | yes | |
|
||||
| DiversityPartitioner | | Partitioning according to label diversity |
|
||||
| DirichletPartitioner | | Partitioning according to dir. distribution of labels |
|
||||
|
||||
|
||||
[//]: # (## **bmk_name**)
|
||||
|
||||
[//]: # (<div id="bmk_name"></div>)
|
||||
|
||||
[//]: # ()
|
||||
[//]: # (description here.)
|
||||
|
||||
[//]: # ()
|
||||
[//]: # (### model)
|
||||
|
||||
[//]: # (| **Model Name** | **Non-Fed Performance** | **NumPara** | **Implementation** |)
|
||||
|
||||
[//]: # (|----------------|-------------------------|-------------|--------------------|)
|
||||
|
||||
[//]: # (| - | - | | - |)
|
||||
|
||||
[//]: # ()
|
||||
[//]: # (### supported partitioner)
|
||||
|
||||
[//]: # (| Name | IsDefault | Comments |)
|
||||
|
||||
[//]: # (|----------------------|-----------|--------------------------------------------------------|)
|
||||
|
||||
[//]: # (| IIDPartitioner | yes | |)
|
||||
|
||||
[//]: # (| DiversityPartitioner | | Partitioning according to label diversity |)
|
||||
|
||||
[//]: # (| DirichletPartitioner | | Partitioning according to dir. distribution of labels |)
|
||||
|
|
@ -0,0 +1,40 @@
|
|||
# Overview
|
||||
| **Name** | **Dataset** | **Description** | **Scene** | **Download** | **Remark** |
|
||||
|-------------------------|----------------------------------------------------------|--------------------------------------|---------------|--------------------------------------------------------------------------------------------------------|------------|
|
||||
| penntreebank_modeling | [PennTreebank]() | [See here](#penntreebank_modeling) | Horizontal FL | [Click Here](https://github.com/WwZzz/easyFL/raw/FLGo/resources/benchmark/penntreebank_modeling.zip) | - |
|
||||
| wikitext2_modeling | [WikiText2]() | [See here](#wikitext2_modeling) | Horizontal FL | [Click Here](https://github.com/WwZzz/easyFL/raw/FLGo/resources/benchmark/wikitext2_modeling.zip) | |
|
||||
|
||||
# Details
|
||||
|
||||
## **penntreebank_modeling**
|
||||
<div id="penntreebank_modeling"></div>
|
||||
|
||||
### model
|
||||
| **Model Name** | **Non-Fed Performance** | **NumPara** | **Implementation** |
|
||||
|----------------|-------------------------|-------------|--------------------|
|
||||
| Transformer | - | | - |
|
||||
|
||||
### supported partitioner
|
||||
| Name | IsDefault | Comments |
|
||||
|----------------------|-----------|--------------------------------------------------------|
|
||||
| IIDPartitioner | yes | |
|
||||
| DiversityPartitioner | | Partitioning according to label diversity |
|
||||
| DirichletPartitioner | | Partitioning according to dir. distribution of labels |
|
||||
|
||||
|
||||
## **wikitext2_modeling**
|
||||
<div id="wikitext2_modeling"></div>
|
||||
-
|
||||
### model
|
||||
| **Model Name** | **Non-Fed Performance** | **NumPara** | **Implementation** |
|
||||
|----------------|-------------------------|-------------|--------------------|
|
||||
| Transformer | - | | - |
|
||||
|
||||
|
||||
### supported partitioner
|
||||
| Name | IsDefault | Comments |
|
||||
|----------------------|-----------|--------------------------------------------------------|
|
||||
| IIDPartitioner | yes | |
|
||||
| DiversityPartitioner | | Partitioning according to label diversity |
|
||||
| DirichletPartitioner | | Partitioning according to dir. distribution of labels |
|
||||
|
|
@ -0,0 +1,21 @@
|
|||
# Overview
|
||||
| **Name** | **Dataset** | **Description** | **Scene** | **Download** | **Remark** |
|
||||
|-------------------------|-----------------------------------------------------|--------------------------------------|---------------|--------------------------------------------------------------------------------------------------------|------------|
|
||||
| multi30k_translation | [Multi30k]() | [See here](#multi30k_translation) | Horizontal FL | [Click Here](https://github.com/WwZzz/easyFL/raw/FLGo/resources/benchmark/multi30k_translation.zip) | - |
|
||||
|
||||
# Details
|
||||
|
||||
## **multi30k_translation**
|
||||
<div id="multi30k_translation"></div>
|
||||
|
||||
### model
|
||||
| **Model Name** | **Non-Fed Performance** | **NumPara** | **Implementation** |
|
||||
|----------------|-------------------------|-------------|--------------------|
|
||||
| Transformer | - | | - |
|
||||
|
||||
### supported partitioner
|
||||
| Name | IsDefault | Comments |
|
||||
|----------------------|-----------|--------------------------------------------------------|
|
||||
| IIDPartitioner | yes | |
|
||||
| DiversityPartitioner | | Partitioning according to label diversity |
|
||||
| DirichletPartitioner | | Partitioning according to dir. distribution of labels |
|
|
@ -0,0 +1,26 @@
|
|||
# Usage
|
||||
Each benchmark is compressed as a .zip file. To use benchmarks here, please follow the three steps below
|
||||
|
||||
1. Download the benchmark .zip file
|
||||
|
||||
2. Decompress the .zip file into the currently working project directory
|
||||
|
||||
3. Use the decompressed directory as a python module, and generate federated task from it
|
||||
|
||||
## Example on MNIST
|
||||
1. download mnist_classification.zip from [here](https://github.com/WwZzz/easyFL/raw/FLGo/resources/benchmark/image/classification/mnist_classification.zip) and decompress it into the currently working project directory.
|
||||
|
||||
2. Write codes as follows to use it
|
||||
|
||||
```python
|
||||
import flgo
|
||||
import mnist_classification
|
||||
|
||||
task = './test_mnist_download'
|
||||
flgo.gen_task({'benchmark': mnist_classification}, task)
|
||||
|
||||
import flgo.algorithm.fedavg as fedavg
|
||||
|
||||
flgo.init(task, fedavg, {'gpu':0}).run()
|
||||
|
||||
```
|
|
@ -0,0 +1,49 @@
|
|||
This is a library for sharing the resources. You can contribute to this library by
|
||||
uploading your personal algorithms, simulators, and benchmarks. All the resources here will
|
||||
be opened to the public to promote interaction among developers/researchers.
|
||||
|
||||
# Usages
|
||||
The usages of the three kinds of resources are respectively introduced in [Usage Algorithm](./algorithm/index.md), [Usage Benchmark](./benchmark/index.md), [Usage Simulator](./simulator/index.md)
|
||||
|
||||
# Contribute to Resources
|
||||
We welcome researchers to contribute to this open-source library to share their own studies by introducing new benchmarks, novel algorithms, and more practical simulators, as we hope this can promoto the development of FL community.
|
||||
To simplify integrating different kinds of resources, we have also provided easy APIs and comprehensive [tutorials](../Tutorials/index.md).
|
||||
We will remark the contributors for the submitted resources in our website.
|
||||
|
||||
## Submit Contributions
|
||||
There are two ways to submit your contributions to this platform.
|
||||
|
||||
### (1) Push commits to the Github repo
|
||||
- **Firstly**, clone our github repo
|
||||
```shell
|
||||
git clone https://github.com/WwZzz/easyFL.git
|
||||
```
|
||||
|
||||
- **Secondly**, git add your resources in proper positions (i.e. benchmark, algorithm, or simulator) in easyFL/resources
|
||||
For example,
|
||||
```
|
||||
└─ resources
|
||||
├─ algorithm # algorithm files of .py should be placed here
|
||||
│ ├─ fedavg.py
|
||||
│ └─ ...
|
||||
├─ benchmark # benchmark files of .zip should be placed here
|
||||
│ ├─ mnist_classification.zip
|
||||
│ └─ ...
|
||||
└─ simulator # simulator files of .py should be placed here
|
||||
├─ ...
|
||||
└─ ...
|
||||
|
||||
```
|
||||
|
||||
- **Thirdly**, git commit your changes with necessary information and push it to our repo, which includes
|
||||
|
||||
> **algorithm**: publishment, year, scene (e.g. horizontal, vertical or others)
|
||||
>
|
||||
> **benchmark**: the name of dataset, the type of data, the type of the ML task
|
||||
>
|
||||
> **simulator**: the name of simulator, synthetic-based or real-world-based
|
||||
|
||||
### (2) Contact us through email
|
||||
Send resources to me through [E-mail](../about.md). The necessacy information should also be contained.
|
||||
|
||||
|
|
@ -0,0 +1,12 @@
|
|||
# Overview
|
||||
|
||||
| **Name** | **Download** | **Description** | **Types** | **Remark** |
|
||||
|-------------------|-------------------------------------------------------------------------------------------------------------|-----------------|------------------|------------|
|
||||
| default_simulator | [source code](https://raw.githubusercontent.com/WwZzz/easyFL/FLGo/resources/simulator/default_simulator.py) | - | AVL\COM\RES\CON | |
|
||||
| phone_simulator | [source code](https://raw.githubusercontent.com/WwZzz/easyFL/FLGo/resources/simulator/phone_simulator.py) | - | AVL\COM\RES\CON | |
|
||||
| | | | | |
|
||||
|
||||
# Details
|
||||
## default_simulator
|
||||
|
||||
## phone_simulator
|
|
@ -0,0 +1,24 @@
|
|||
|
||||
# Usage
|
||||
Each simulator is a new defined class inheriting from ```flgo.simulator.base.BasicSimulator```. To use simulators here, please follow the two steps below
|
||||
|
||||
1. Copy the source code of simulator
|
||||
|
||||
2. Write codes as follows to use it
|
||||
|
||||
```python
|
||||
import flgo
|
||||
import flgo.simulator.base
|
||||
import mnist_classification
|
||||
|
||||
#### 1. Paste Souce code here ##########################
|
||||
class MySimulator(flgo.simulator.base.BasicSimulator):
|
||||
...
|
||||
########################################################
|
||||
task = './test_mnist_download'
|
||||
flgo.gen_task({'benchmark': mnist_classification}, task)
|
||||
import flgo.algorithm.fedavg as fedavg
|
||||
|
||||
##### 2. Specify simulator here by para Simulator in flgo.init######
|
||||
flgo.init(task, fedavg, {'gpu':0}, Simulator=MySimulator).run()
|
||||
```
|
|
@ -0,0 +1,62 @@
|
|||
## Install FLGo
|
||||
|
||||
Install FLGo through pip.
|
||||
|
||||
```
|
||||
pip install flgo
|
||||
```
|
||||
|
||||
If the package is not found, please use the command below to update pip
|
||||
|
||||
```
|
||||
pip install --upgrade pip
|
||||
```
|
||||
|
||||
## Create Your First Federated Task
|
||||
|
||||
Here we take the classical federated benchmark, Federated MNIST [1], as the example, where the MNIST dataset is splitted into 100 parts identically and independently.
|
||||
|
||||
```python
|
||||
import flgo
|
||||
import os
|
||||
|
||||
# the target path of the task
|
||||
task_path = './my_first_task'
|
||||
|
||||
# create task configuration
|
||||
task_config = {'benchmark':{'name': 'flgo.benchmark.mnist_classification'}, 'partitioner':{'name':'IIDPartitioner', 'para':{'num_clients':100}}}
|
||||
|
||||
# generate the task if the task doesn't exist
|
||||
if not os.path.exists(task_path):
|
||||
flgo.gen_task(task_config, task_path)
|
||||
```
|
||||
|
||||
After running the codes above, a federated dataset is successfully created in the `task_path`. The visualization of the task is stored in
|
||||
`task_path/res.png` as below
|
||||

|
||||
|
||||
|
||||
|
||||
## Run FedAvg to Train Your Model
|
||||
Now we are going to run the classical federated optimization algorithm, FedAvg [1], on the task created by us to train a model.
|
||||
```python
|
||||
import flgo.algorithm.fedavg as fedavg
|
||||
# create fedavg runner on the task
|
||||
runner = flgo.init(task, fedavg, {'gpu':[0,],'log_file':True, 'num_steps':5})
|
||||
runner.run()
|
||||
```
|
||||
|
||||
## Show Training Result
|
||||
The training result is saved as a record under the dictionary of the task `task_path/record`. We use the built-in analyzer to read and show it.
|
||||
```python
|
||||
import flgo.experiment.analyzer
|
||||
# create the analysis plan
|
||||
analysis_plan = {
|
||||
'Selector':{'task': task_path, 'header':['fedavg',], },
|
||||
'Painter':{'Curve':[{'args':{'x':'communication_round', 'y':'val_loss'}}]},
|
||||
'Table':{'min_value':[{'x':'val_loss'}]},
|
||||
}
|
||||
|
||||
flgo.experiment.analyzer.show(analysis_plan)
|
||||
```
|
||||

|
|
@ -0,0 +1,35 @@
|
|||
# 1.1 Descriptor of FL
|
||||
|
||||
We first introduce how we describe FL in our framework. We use the API ```flgo.init``` to create a federated runner to finish a run of FL, which is described as below:
|
||||
|
||||
```
|
||||
def init(task: str, algorithm, option = {}, model=None, Logger: flgo.experiment.logger.BasicLogger = flgo.experiment.logger.simple_logger.SimpleLogger, Simulator: BasicSimulator=flgo.simulator.DefaultSimulator, scene='horizontal'):
|
||||
r"""
|
||||
Initialize a runner in FLGo, which is to optimize a model on a specific task (i.e. IID-mnist-of-100-clients) by the selected federated algorithm.
|
||||
:param
|
||||
task (str): the dictionary of the federated task
|
||||
algorithm (module || class): the algorithm will be used to optimize the model in federated manner, which must contain pre-defined attributions (e.g. algorithm.Server and algorithm.Client for horizontal federated learning)
|
||||
option (dict || str): the configurations of training, environment, algorithm, logger and simulator
|
||||
model (module || class): the model module that contains two methods: model.init_local_module(object) and model.init_global_module(object)
|
||||
Logger (class): the class of the logger inherited from flgo.experiment.logger.BasicLogger
|
||||
Simulator (class): the class of the simulator inherited from flgo.simulator.BasicSimulator
|
||||
scene (str): 'horizontal' or 'vertical' in current version of FLGo
|
||||
:return
|
||||
runner: the object instance that has the method runner.run()
|
||||
"""
|
||||
...
|
||||
```
|
||||
|
||||
Each run of a federated training process aims to optimize a given **model** on a specific **task** by using an **algorithm** with some hyper-parameter (i.e. **option**) under a particular environment (e.g. **scene**, **hardware condition**).
|
||||
|
||||
The term **model** usually shares the same meaning with centralized ML. The term **task** describes how the datasets are distributed among clients and some task-specific configuration (e.g. the dataset information, the target). **Algorithm** is the used optimization strategy and **option** contains several running-time option like learning rate and the number of training rounds. The **hardware condition** is simulated by the **Simulator**. For example, different clients may have different computing power, network latency, communication bandwidth. Finally, the **scene** refers to the four main paradigm in FL: Horizontal FL, Vertical FL, Decentralized FL and Hierarchical FL, as shown in Figure 1.
|
||||
|
||||
- **(a) Horizontal FL**: a server coordinates different clients to collaboratively train the model. Particularly, each clients owns different samples, and each sample is with full features and labels.
|
||||
- **(b) Vertical FL**: an active party (i.e. label owner) coordinates other passive parties to improve the model performance for its local objective. Particularly, different parties own different dimensions of the feature of each sample, and different data owners will shares a set of the same sample IDs.
|
||||
- **(c) Hierarchical FL**: edge servers are responsible for coordinating their themselves clients, and a global server coordinates different edge servers to train the model.
|
||||
- **(d) Decentralized FL**: clients directly communicates with other clients to collaboratively maintain a global model or improve their own local models under specific communication protocols (e.g. line, ring, full).
|
||||
|
||||
|
||||

|
||||
|
||||
Finally, from the view of doing experiments, we add the term **Logger** to customizely log the variables of interest (e.g. model checkpoints, training-time performance). Some options of experiments (e.g. device, the number of processes) are also contained in the term **option**.
|
|
@ -0,0 +1,44 @@
|
|||
|
||||
# 1.2 Option Configuration
|
||||
|
||||
The full options are shown as below
|
||||
|
||||
| Category | Name | Type | Description | Default Value | Comment |
|
||||
|----------------------|---------------------|-----------|------------------------------------------------------------------------------------------------|---------------|-------------------------------------------------------------------------|
|
||||
| **Training Option** | num_rounds | int | number of communication rounds | 20 | |
|
||||
| | proportion | float | proportion of clients sampled per round | 0.2 | |
|
||||
| | learning_rate_decay | float | learning rate decay for the training process | 0.998 | effective if lr_scheduler>-1 |
|
||||
| | lr_scheduler | int | type of the global learning rate scheduler | -1 | effective if larger than -1 |
|
||||
| | early_stop | int | stop training if there is no improvement for no smaller than the maximum rounds | -1 | effective if larger than -1 |
|
||||
| | num_epochs | int | number of epochs of local training | 5 | |
|
||||
| | num_steps | int | number of steps of local training, conflict with num_epochs | -1 | dominates num_epochs if larger than 0 |
|
||||
| | learning_rate | float | learning rate of local training | 0.1 | |
|
||||
| | batch_size | int\float | batch size of local training | 64 | -1 means full batch, float value means the ratio of the full datasets |
|
||||
| | optimizer | str | to select the optimizer of local training | 'sgd' | 'sgd','adam','rmsprop','adagrad' |
|
||||
| | clip_grad | float | clipping gradients if the max norm of gradients \ | \ |g\|\| > clip_norm > 0 | 0.0 | effective if larger than 0.0 |
|
||||
| | momentum | float | momentum of local training | 0.0 | |
|
||||
| | weight_decay | float | weight decay of local training | 0.0 | |
|
||||
| | num_edge_rounds | int | number of edge rounds in hierFL | 5 | effective if scene is 'hierarchical' |
|
||||
| | algo_para | int\list | algorithm-specific hyper-parameters | [] | the order should be consistent with the claim |
|
||||
| | sample | str | to select sampling form | 'uniform' | 'uniform', 'md', 'full', x+'_with_availability' |
|
||||
| | aggregate | str | to select aggregation form | 'other' | 'uniform', 'weighted_com', 'weighted_scale', 'other' |
|
||||
| **External Option** | train_holdout | float | the rate of holding out the validation dataset from all the local training datasets | 0.1 | |
|
||||
| | test_holdout | float | the rate of holding out the validation dataset from the testing datasets owned by the server | 0.0 | effective if the server has no validation data |
|
||||
| | local_test | bool | the local validation data will be equally split into validation and testing parts if True | False | |
|
||||
| | seed | int | seed for all the random modules | 0 | |
|
||||
| | dataseed | int | seed for all the random modules for local data train/val/test partition | 0 | |
|
||||
| | gpu | int\list | GPU IDs and empty input means using CPU | [] | |
|
||||
| | server_with_cpu | bool | the model parameters will be stored in the memory if True | False | |
|
||||
| | num_parallels | int | the number of parallels during communications | 1 | |
|
||||
| | num_workers | int | the number of workers of DataLoader | 0 | |
|
||||
| | pin_memory | bool | pin_memory of DataLoader | False | |
|
||||
| | test_batch_size | int | the batch_size used in testing phase | 512 | |
|
||||
| **Simulator Option** | availability | str | to select client availability mode | 'IDL' | 'IDL','YMF','MDF','LDF','YFF', 'HOMO','LN','SLN','YC' |
|
||||
| | connectivity | str | to select client connectivity mode | 'IDL' | 'IDL','HOMO' |
|
||||
| | completeness | str | to select client completeness mode | 'IDL' | 'IDL','PDU','FSU','ADU','ASU' |
|
||||
| | responsiveness | str | to select client responsiveness mode | 'IDL' | 'IDL','LN','UNI' |
|
||||
| **Logger Option** | log_level | str | the level of logger | 'INFO' | 'INFO','DEBUG' |
|
||||
| | log_file | bool | whether log to file and default value is False | False | |
|
||||
| | no_log_console | bool | whether log to screen and default value is True | True | |
|
||||
| | no_overwrite | bool | whether to overwrite the old result | False | |
|
||||
| | eval_interval | int | evaluate every __ rounds; | 1 | |
|
|
@ -0,0 +1,118 @@
|
|||
|
||||
# 1.3 Task Configuration
|
||||
|
||||
This section introduces the definition of a federated task and how to run algorihms on different tasks in our frameworks. A federated task is defined as **optimizing an objective on a given data distribution**.
|
||||
|
||||
Concretely, **the objective** is defined by the dataset, the objective function, and the evaluation metrics, and each benchmark module is consist of these three terms. For example, the benchmark `flgo.benchmark.mnist_classification` requires a model to perform correct classification on hand written digits images, which is evaluated by the accuracy.
|
||||
|
||||
On the other hand, the **data distribution** suggests how the data is distributed among participants. For example, each participent may owns data that is identically and independently sampled from a global dataset, which is called the i.i.d. case. In our framework, `Paritioner` is responsible for creating such data distributions.
|
||||
|
||||
In our framework, we use the configuration of `benchmark` and `partitioner` to generate federated tasks. We now take a example to show how to write configurations to generate different data distributions on the same given benchmark.
|
||||
|
||||
## 1.3.1 Example: MNIST classification under different data distributions
|
||||
|
||||
Firstly, each config is of the type `dict` in python. The key 'benchmark' and 'partitioner' repsectively specify the information about the aforementioned benchmark and Partitioner.
|
||||
|
||||
|
||||
```python
|
||||
import flgo
|
||||
flgo.set_data_root('cwd') # change the directory storing raw data to the current working directory
|
||||
import flgo.benchmark.mnist_classification as mnist
|
||||
import flgo.algorithm.fedavg as fedavg
|
||||
import flgo.benchmark.partition
|
||||
import os
|
||||
# DiversityPartitioner will allocate the data to clients w.r.t. data diversity (e.g. here is label diversity)
|
||||
# DirichletPartitioner will allocate the data to clients w.r.t. dirichlet distribution on specific attr. (e.g. here is also label)
|
||||
config_iid = {'benchmark':mnist,'partitioner':{'name': flgo.benchmark.partition.IIDPartitioner,'para':{'num_clients':100}}}
|
||||
config_div01 = {'benchmark':mnist,'partitioner':{'name': flgo.benchmark.partition.DiversityPartitioner,'para':{'num_clients':100, 'diversity':0.1}}}
|
||||
config_div05 = {'benchmark':mnist,'partitioner':{'name': flgo.benchmark.partition.DiversityPartitioner,'para':{'num_clients':100, 'diversity':0.5}}}
|
||||
config_div09 = {'benchmark':mnist,'partitioner':{'name': flgo.benchmark.partition.DiversityPartitioner,'para':{'num_clients':100, 'diversity':0.9}}}
|
||||
config_dir01 = {'benchmark':mnist,'partitioner':{'name': flgo.benchmark.partition.DirichletPartitioner,'para':{'num_clients':100, 'alpha':0.1}}}
|
||||
config_dir10 = {'benchmark':mnist,'partitioner':{'name': flgo.benchmark.partition.DirichletPartitioner,'para':{'num_clients':100, 'alpha':1.0}}}
|
||||
config_dir50 = {'benchmark':mnist,'partitioner':{'name': flgo.benchmark.partition.DirichletPartitioner,'para':{'num_clients':100, 'alpha':5.0}}}
|
||||
task_dict = {
|
||||
'./mnist_iid': config_iid,
|
||||
'./mnist_div01': config_div01,
|
||||
'./mnist_div05': config_div05,
|
||||
'./mnist_div09': config_div09,
|
||||
'./mnist_dir01': config_dir01,
|
||||
'./mnist_dir10': config_dir10,
|
||||
'./mnist_dir50': config_dir50,
|
||||
}
|
||||
|
||||
for task in task_dict:
|
||||
if not os.path.exists(task):
|
||||
flgo.gen_task(task_dict[task], task)
|
||||
```
|
||||
|
||||
Data root directory has successfully been changed to /home/wz/xw_d2l/Jupyter
|
||||
|
||||
|
||||
Secondly, use FedAvg to optimize these tasks with the same hyper-parameters. Note that each benchmark will be attached with a default model (e.g. a predefined CNN for mnist_classification), and initialization without specifying model will automatically load the default model.
|
||||
|
||||
|
||||
```python
|
||||
import flgo.algorithm.fedavg as fedavg
|
||||
option = {'gpu':0, 'num_rounds':20, 'num_epochs':1, 'learning_rate':0.1, 'batch_size':64, 'eval_interval':2}
|
||||
runners = [flgo.init(task, fedavg, option) for task in task_dict]
|
||||
for runner in runners:
|
||||
runner.run()
|
||||
```
|
||||
|
||||
|
||||
|
||||
Thirdly, use `flgo.experiment.analyzer` to read the records and visuazlie the results.
|
||||
|
||||
|
||||
```python
|
||||
import flgo.experiment.analyzer as al
|
||||
import matplotlib.pyplot as plt
|
||||
div_recs = al.Selector({'task':[t for t in task_dict if 'iid' in t or 'div' in t], 'header':['fedavg']})
|
||||
|
||||
plt.subplot(221)
|
||||
for task in div_recs.tasks:
|
||||
rec_list = div_recs.records[task]
|
||||
for rec in rec_list:
|
||||
plt.plot(rec.data['communication_round'], rec.data['test_accuracy'], label=task.split('/')[-1])
|
||||
plt.title('testing accuracy - diversity')
|
||||
plt.ylabel('test_accuracy')
|
||||
plt.xlabel('communication round')
|
||||
plt.legend()
|
||||
|
||||
plt.subplot(222)
|
||||
for task in div_recs.tasks:
|
||||
rec_list = div_recs.records[task]
|
||||
for rec in rec_list:
|
||||
plt.plot(rec.data['communication_round'], rec.data['test_loss'], label=task.split('/')[-1])
|
||||
plt.title('testing loss - diversity')
|
||||
plt.ylabel('test_loss')
|
||||
plt.xlabel('communication round')
|
||||
plt.legend()
|
||||
|
||||
plt.subplot(223)
|
||||
dir_recs = al.Selector({'task':[task for task in task_dict if 'iid' in task or 'dir' in task], 'header':['fedavg']})
|
||||
for task in dir_recs.tasks:
|
||||
rec_list = dir_recs.records[task]
|
||||
for rec in rec_list:
|
||||
plt.plot(rec.data['communication_round'], rec.data['test_accuracy'], label=task.split('/')[-1])
|
||||
plt.title('testing accuracy - Dirichlet')
|
||||
plt.ylabel('test_accuracy')
|
||||
plt.xlabel('communication round')
|
||||
plt.legend()
|
||||
|
||||
plt.subplot(224)
|
||||
dir_recs = al.Selector({'task':[task for task in task_dict if 'iid' in task or 'dir' in task], 'header':['fedavg']})
|
||||
for task in dir_recs.tasks:
|
||||
rec_list = dir_recs.records[task]
|
||||
for rec in rec_list:
|
||||
plt.plot(rec.data['communication_round'], rec.data['test_loss'], label=task.split('/')[-1])
|
||||
plt.title('testing loss - Dirichlet')
|
||||
plt.ylabel('test_loss')
|
||||
plt.xlabel('communication round')
|
||||
plt.legend()
|
||||
plt.show()
|
||||
```
|
||||
|
||||
|
||||
|
||||

|
|
@ -0,0 +1,74 @@
|
|||
|
||||
# 1.4 Algorithm Configuration
|
||||
|
||||
This section introduces how to change algorithms on the same federated task. In our framework, the algorithm object describes the behaviors of participants in the FL system. For example, the server of horizontal FL usually samples clients, broadcasts the global model to them, and aggregates the collected models from them, where there exist a plenty of different strategies to improve each step. Now we compare a heterogeneous-aware FL algorithm, FedProx, against FedAvg under the same task to show the usage of algorihm.
|
||||
|
||||
## 1.4.1 Example: Comparison on FedAvg and FedProx
|
||||
|
||||
### Dataset: Synthetic(0.5, 0.5)
|
||||
|
||||
We conduct the experiments that shares the same setting in []. Firstly, we generate synthetic datasets with 30 clients. This dataset is generated by
|
||||
|
||||
$$y_{k,i}=\text{argmax}\{softmax({W_k} x_{k,i}+ b_k)\}$$
|
||||
|
||||
where $(x_{k,i}, y_{k,i})$ is the $i$th example in the local data $D_k$ of client $c_k$. For each client $c_k$, its local optimal model parameter $(W_k, b_k)$ is generated by $\mu_k\sim \mathcal{N}(0,\alpha)\in \mathbb{R},W_{k}[i,j]\sim\mathcal{N}(\mu_k,1), W_k\in \mathbb{R}^{10\times 60}, b_{k}[i]\sim\mathcal{N}(\mu_k,1), b_k\in\mathbb{R}^{10}$, and its local data distribution is generated by $B_k\sim\mathcal{N}(0,\beta), v_k[i]\sim\mathcal{N}(B_k,1), v_k\in \mathbb{R}^{60}, x_{k,i}\sim\mathcal{N}(v_k, \Sigma)\in \mathbb{R}^{60},\Sigma=\text{diag}(\{i^{-1.2}\}_{i=1}^{60})$. Here we conduct experiments on Synthetic(0.5,0.5) where $\alpha=\beta=0.5$.
|
||||
|
||||
|
||||
```python
|
||||
import os
|
||||
import flgo
|
||||
task = './test_synthetic'
|
||||
config = {'benchmark':{'name':'flgo.benchmark.synthetic_regression', 'para':{'alpha':0.5, 'beta':0.5, 'num_clients':30}}}
|
||||
if not os.path.exists(task): flgo.gen_task(config, task_path = task)
|
||||
```
|
||||
|
||||
Task ./test_synthetic has been successfully generated.
|
||||
|
||||
|
||||
### Running two algorithms
|
||||
|
||||
|
||||
```python
|
||||
import flgo.algorithm.fedprox as fedprox
|
||||
import flgo.algorithm.fedavg as fedavg
|
||||
|
||||
option = {'num_rounds':200, 'num_epochs':5, 'batch_size':10, 'learning_rate':0.1, 'gpu':0}
|
||||
fedavg_runner = flgo.init(task, fedavg, option=option)
|
||||
fedprox_runner = flgo.init(task, fedprox, option=option)
|
||||
fedavg_runner.run()
|
||||
fedprox_runner.run()
|
||||
```
|
||||
|
||||
### Plot the results
|
||||
|
||||
|
||||
```python
|
||||
import flgo.experiment.analyzer
|
||||
analysis_plan = {
|
||||
'Selector':{
|
||||
'task': task,
|
||||
'header':['fedavg', 'fedprox']
|
||||
},
|
||||
'Painter':{
|
||||
'Curve':[
|
||||
{'args':{'x': 'communication_round', 'y':'val_loss'}, 'fig_option':{'title':'valid loss on Synthetic'}},
|
||||
{'args':{'x': 'communication_round', 'y':'val_accuracy'}, 'fig_option':{'title':'valid accuracy on Synthetic'}},
|
||||
]
|
||||
}
|
||||
}
|
||||
flgo.experiment.analyzer.show(analysis_plan)
|
||||
```
|
||||
|
||||
|
||||
|
||||

|
||||
|
||||
|
||||
|
||||
|
||||
|
||||

|
||||
|
||||
|
||||
|
||||
The result tells that FedProx has superior performance than FedAvg under this setting of data heterogeneity.
|
|
@ -0,0 +1,376 @@
|
|||
|
||||
# 1.5 Model Configuration
|
||||
|
||||
This section introduces how to change the model. Far now, we only train the default model for each benchmark (e.g. CNN for mnist_classification). For most of the benchmarks, we have provided several popular models that can be easily used by replacing the parameter **model** in `flgo.init`. We show the usage by the following example.
|
||||
|
||||
## 1.5.1 Example: Select model for MNIST
|
||||
|
||||
|
||||
```python
|
||||
import flgo.benchmark.mnist_classification.model.cnn as cnn
|
||||
import flgo.benchmark.mnist_classification.model.mlp as mlp
|
||||
import flgo.algorithm.fedavg as fedavg
|
||||
task = './mnist_iid' # this task has been generated in Example 2.1
|
||||
cnn_runner = flgo.init(task, fedavg, option={'num_rounds':5, 'num_epochs':1, 'gpu':0}, model=cnn)
|
||||
mlp_runner = flgo.init(task, fedavg, option={'num_rounds':5, 'num_epochs':1, 'gpu':0}, model=mlp)
|
||||
cnn_runner.run()
|
||||
mlp_runner.run()
|
||||
|
||||
# result analysis
|
||||
import flgo.experiment.analyzer
|
||||
analysis_plan = {
|
||||
'Selector':{
|
||||
'task': task,
|
||||
'header':['fedavg'],
|
||||
'filter':{'M':['cnn', 'mlp'], 'R':5}, # filter the result by the communication round R=5
|
||||
'legend_with':['M']
|
||||
},
|
||||
'Painter':{
|
||||
'Curve':[
|
||||
{'args':{'x': 'communication_round', 'y':'val_loss'}, 'fig_option':{'title':'valid loss on MNIST'}},
|
||||
{'args':{'x': 'communication_round', 'y':'val_accuracy'}, 'fig_option':{'title':'valid accuracy on MNIST'}},
|
||||
]
|
||||
}
|
||||
}
|
||||
flgo.experiment.analyzer.show(analysis_plan)
|
||||
```
|
||||
|
||||
|
||||
|
||||

|
||||
|
||||
|
||||
|
||||
|
||||
|
||||

|
||||
|
||||
|
||||
|
||||
## 1.5.2 Customization on models
|
||||
|
||||
We now discuss the impletementation of models in our framework. Different from the centralized ML setting where there is only a model that transform the input to the output, the **model** in our framework should describe what and how the models are kept by different participants. This is because different parties in FL may have models with different architectures and paramters (e.g. personzalized FL, vertical FL, model-agnostic FL). In addition, the model sometimes could also be a significant part of particular methods. Therefore, we define the model as a class as follows:
|
||||
|
||||
|
||||
```python
|
||||
class GeneralModel:
|
||||
@classmethod
|
||||
def init_local_module(cls, object):
|
||||
"""init local models (e.g. personal models that cannot be shared) for the object according to its information"""
|
||||
pass
|
||||
|
||||
@classmethod
|
||||
def init_global_module(cls, object):
|
||||
"""init global models (e.g. sharable models) for the object according to its information"""
|
||||
pass
|
||||
```
|
||||
|
||||
Now we construct a model and test it as the example.
|
||||
|
||||
|
||||
```python
|
||||
from torch import nn
|
||||
import torch.nn.functional as F
|
||||
from flgo.utils.fmodule import FModule
|
||||
|
||||
class CNNModel(FModule): # inherit from flgo.utils.fmodule.FModule instead of torch.nn.Module
|
||||
def __init__(self):
|
||||
super().__init__()
|
||||
self.conv1 = nn.Conv2d(in_channels=1, out_channels=32, kernel_size=5, padding=2)
|
||||
self.conv2 = nn.Conv2d(in_channels=32, out_channels=64, kernel_size=5, padding=2)
|
||||
self.fc1 = nn.Linear(3136, 512)
|
||||
self.fc2 = nn.Linear(512, 10)
|
||||
|
||||
def forward(self, x):
|
||||
x = x.view((x.shape[0],28,28))
|
||||
x = x.unsqueeze(1)
|
||||
x = F.max_pool2d(F.relu(self.conv1(x)), 2)
|
||||
x = F.max_pool2d(F.relu(self.conv2(x)), 2)
|
||||
x = x.view(-1, x.shape[1]*x.shape[2]*x.shape[3])
|
||||
x = F.relu(self.fc1(x))
|
||||
x = self.fc2(x)
|
||||
return x
|
||||
|
||||
def init_local_module(object):
|
||||
pass
|
||||
|
||||
def init_global_module(object):
|
||||
# In classical horizontal FL, only the server needs to trace the latest global and store it
|
||||
if 'Server' in object.get_classname():
|
||||
object.model = CNNModel().to(object.device)
|
||||
|
||||
class MyCNN:
|
||||
init_local_module = init_local_module
|
||||
init_global_module = init_global_module
|
||||
|
||||
mycnn_runner = flgo.init(task, fedavg, option={'num_rounds':5, 'num_epochs':1, 'gpu':0}, model=MyCNN)
|
||||
mycnn_runner.run()
|
||||
|
||||
analysis_plan = {
|
||||
'Selector':{
|
||||
'task': task,
|
||||
'header':['fedavg'],
|
||||
'filter':{'M':['MyCNN', 'cnn'], 'R':5},
|
||||
'legend_with':['M']
|
||||
},
|
||||
'Painter':{
|
||||
'Curve':[
|
||||
{'args':{'x': 'communication_round', 'y':'val_loss'}, 'fig_option':{'title':'valid loss on MNIST'}},
|
||||
{'args':{'x': 'communication_round', 'y':'val_accuracy'}, 'fig_option':{'title':'valid accuracy on MNIST'}},
|
||||
]
|
||||
}
|
||||
}
|
||||
flgo.experiment.analyzer.show(analysis_plan)
|
||||
```
|
||||
|
||||
|
||||
|
||||

|
||||
|
||||
|
||||
|
||||
|
||||
|
||||

|
||||
|
||||
|
||||
|
||||
The two CNNs of the same architecture has a similar performance in this example.
|
||||
|
||||
## 1.5.3 What is FModule?
|
||||
|
||||
`FModule` is a class that decorates the class `torch.nn.Module` to enable direct operations on models like add, sub. `FModule` directly inherits from `torch.nn.Module` and won't have any impact on its original characteristics. The only difference lies in that `FModule` allows the model-level operations by using operators +,-,* to obtain a new model. We show the usage of `FModule` by the following example.
|
||||
|
||||
### 1.5.3.1 Example: Model-level operators
|
||||
|
||||
|
||||
```python
|
||||
from torch import nn
|
||||
import torch.nn.functional as F
|
||||
from flgo.utils.fmodule import FModule
|
||||
|
||||
class Model(FModule):
|
||||
def __init__(self):
|
||||
super().__init__()
|
||||
self.fc = nn.Linear(3, 3, bias=False)
|
||||
|
||||
def forward(self, x):
|
||||
return x
|
||||
|
||||
A = Model()
|
||||
B = Model()
|
||||
```
|
||||
|
||||
```python
|
||||
print("model A: ", A.head.weight)
|
||||
print("model B: ", B.head.weight)
|
||||
|
||||
# add
|
||||
C = A + B # C is a new instance of class Model and changes on C won't have any impact on A or B
|
||||
print("C=A+B: {}", C.head.weight)
|
||||
print("Type of C:", C)
|
||||
```
|
||||
|
||||
model A: Parameter containing:
|
||||
tensor([[ 0.2429, -0.4990, 0.1843],
|
||||
[-0.2553, 0.1664, 0.3536],
|
||||
[ 0.5772, 0.0578, -0.0694]], requires_grad=True)
|
||||
model B: Parameter containing:
|
||||
tensor([[-0.4220, -0.3707, -0.2508],
|
||||
[-0.4888, -0.1267, 0.1310],
|
||||
[ 0.5714, -0.2370, 0.3410]], requires_grad=True)
|
||||
C=A+B: {} Parameter containing:
|
||||
tensor([[-0.1790, -0.8697, -0.0665],
|
||||
[-0.7441, 0.0397, 0.4845],
|
||||
[ 1.1486, -0.1792, 0.2716]], requires_grad=True)
|
||||
Type of C: Model(
|
||||
(fc): Linear(in_features=3, out_features=3, bias=False)
|
||||
)
|
||||
|
||||
```python
|
||||
# sub
|
||||
print('A-B: \n', (A - B).head.weight)
|
||||
print('+++++++++++++++++++++++++++++++++++')
|
||||
# scale
|
||||
print('2*A: \n', (2 * A).fc.weight)
|
||||
print('+++++++++++++++++++++++++++++++++++')
|
||||
# div
|
||||
print('A/2: \n', (A / 2).fc.weight)
|
||||
print('+++++++++++++++++++++++++++++++++++')
|
||||
# norm
|
||||
print('||A||_2: \n', (A ** 2))
|
||||
print('+++++++++++++++++++++++++++++++++++')
|
||||
# neg
|
||||
print('-A: \n', (-A).head.weight)
|
||||
print('+++++++++++++++++++++++++++++++++++')
|
||||
# zeros-copy
|
||||
print('A.zeros_like(): \n', A.zeros_like().head.weight)
|
||||
print('+++++++++++++++++++++++++++++++++++')
|
||||
# dot
|
||||
print("dot(A,B):\n", A.dot(B))
|
||||
print('+++++++++++++++++++++++++++++++++++')
|
||||
# cos-similarity
|
||||
print("cos_sim(A,B):\n", A.cos_sim(B))
|
||||
print('+++++++++++++++++++++++++++++++++++')
|
||||
# size
|
||||
print("size(A):\n", A.count_parameters())
|
||||
print('+++++++++++++++++++++++++++++++++++')
|
||||
```
|
||||
|
||||
A-B:
|
||||
Parameter containing:
|
||||
tensor([[ 0.6649, -0.1282, 0.4352],
|
||||
[ 0.2336, 0.2932, 0.2226],
|
||||
[ 0.0057, 0.2948, -0.4103]], requires_grad=True)
|
||||
+++++++++++++++++++++++++++++++++++
|
||||
2*A:
|
||||
Parameter containing:
|
||||
tensor([[ 0.4859, -0.9979, 0.3687],
|
||||
[-0.5105, 0.3329, 0.7072],
|
||||
[ 1.1543, 0.1156, -0.1388]], requires_grad=True)
|
||||
+++++++++++++++++++++++++++++++++++
|
||||
A/2:
|
||||
Parameter containing:
|
||||
tensor([[ 0.1215, -0.2495, 0.0922],
|
||||
[-0.1276, 0.0832, 0.1768],
|
||||
[ 0.2886, 0.0289, -0.0347]], requires_grad=True)
|
||||
+++++++++++++++++++++++++++++++++++
|
||||
||A||_2:
|
||||
tensor(0.9493)
|
||||
+++++++++++++++++++++++++++++++++++
|
||||
-A:
|
||||
Parameter containing:
|
||||
tensor([[-0.2429, 0.4990, -0.1843],
|
||||
[ 0.2553, -0.1664, -0.3536],
|
||||
[-0.5772, -0.0578, 0.0694]], requires_grad=True)
|
||||
+++++++++++++++++++++++++++++++++++
|
||||
A.zeros_like():
|
||||
Parameter containing:
|
||||
tensor([[0., -0., 0.],
|
||||
[-0., 0., 0.],
|
||||
[0., 0., -0.]], requires_grad=True)
|
||||
+++++++++++++++++++++++++++++++++++
|
||||
dot(A,B):
|
||||
tensor(0.4787)
|
||||
+++++++++++++++++++++++++++++++++++
|
||||
cos_sim(A,B):
|
||||
tensor(0.4703)
|
||||
+++++++++++++++++++++++++++++++++++
|
||||
size(A):
|
||||
9
|
||||
+++++++++++++++++++++++++++++++++++
|
||||
|
||||
|
||||
Besides the model-level operators, we also implement some common functions on model-level.
|
||||
|
||||
```python
|
||||
import flgo.utils.fmodule as ff
|
||||
|
||||
# exp(A)
|
||||
print('exp(A):\n', ff.exp(A).head.weight)
|
||||
print('+++++++++++++++++++++++++++++++++++')
|
||||
# log(A)
|
||||
print('log(A):\n', ff.log(A).head.weight)
|
||||
print('+++++++++++++++++++++++++++++++++++')
|
||||
# model to 1-D vector
|
||||
a = ff._model_to_tensor(A)
|
||||
print('a = Vec(A):\n', a)
|
||||
# 1-D tensor to model
|
||||
print('A from a: \n', ff._model_from_tensor(a, A.__class__).head.weight)
|
||||
print('+++++++++++++++++++++++++++++++++++')
|
||||
# model averaging
|
||||
print('AVERAGE([A,B]):\n', ff._model_average([A, B]).head.weight)
|
||||
# model sum
|
||||
print('SUM([A,B]):\n', ff._model_sum([A, B]).head.weight)
|
||||
```
|
||||
|
||||
exp(A):
|
||||
Parameter containing:
|
||||
tensor([[1.2750, 0.6072, 1.2024],
|
||||
[0.7747, 1.1811, 1.4242],
|
||||
[1.7810, 1.0595, 0.9330]], requires_grad=True)
|
||||
+++++++++++++++++++++++++++++++++++
|
||||
log(A):
|
||||
Parameter containing:
|
||||
tensor([[-1.4149, nan, -1.6910],
|
||||
[ nan, -1.7931, -1.0396],
|
||||
[-0.5496, -2.8510, nan]], requires_grad=True)
|
||||
+++++++++++++++++++++++++++++++++++
|
||||
a = Vec(A):
|
||||
tensor([ 0.2429, -0.4990, 0.1843, -0.2553, 0.1664, 0.3536, 0.5772, 0.0578,
|
||||
-0.0694])
|
||||
A from a:
|
||||
Parameter containing:
|
||||
tensor([[ 0.2429, -0.4990, 0.1843],
|
||||
[-0.2553, 0.1664, 0.3536],
|
||||
[ 0.5772, 0.0578, -0.0694]], requires_grad=True)
|
||||
+++++++++++++++++++++++++++++++++++
|
||||
AVERAGE([A,B]):
|
||||
Parameter containing:
|
||||
tensor([[-0.0895, -0.4348, -0.0333],
|
||||
[-0.3721, 0.0199, 0.2423],
|
||||
[ 0.5743, -0.0896, 0.1358]], requires_grad=True)
|
||||
SUM([A,B]):
|
||||
Parameter containing:
|
||||
tensor([[-0.1790, -0.8697, -0.0665],
|
||||
[-0.7441, 0.0397, 0.4845],
|
||||
[ 1.1486, -0.1792, 0.2716]], requires_grad=True)
|
||||
|
||||
|
||||
## 1.5.4 Fast Customization
|
||||
|
||||
We further provide fast API to convert a model into federated one by writing only one line code
|
||||
|
||||
|
||||
```python
|
||||
from torch import nn
|
||||
import torch.nn.functional as F
|
||||
|
||||
class NewModel(nn.Module):
|
||||
def __init__(self):
|
||||
super().__init__()
|
||||
self.conv1 = nn.Conv2d(in_channels=1, out_channels=32, kernel_size=5, padding=2)
|
||||
self.conv2 = nn.Conv2d(in_channels=32, out_channels=64, kernel_size=5, padding=2)
|
||||
self.fc1 = nn.Linear(3136, 512)
|
||||
self.fc2 = nn.Linear(512, 10)
|
||||
|
||||
def forward(self, x):
|
||||
x = x.view((x.shape[0],28,28))
|
||||
x = x.unsqueeze(1)
|
||||
x = F.max_pool2d(F.relu(self.conv1(x)), 2)
|
||||
x = F.max_pool2d(F.relu(self.conv2(x)), 2)
|
||||
x = x.view(-1, x.shape[1]*x.shape[2]*x.shape[3])
|
||||
x = F.relu(self.fc1(x))
|
||||
x = self.fc2(x)
|
||||
return x
|
||||
|
||||
model = flgo.convert_model(NewModel) # the default value of model_name is 'anonymous'
|
||||
mycnn_runner2 = flgo.init(task, fedavg, option={'num_rounds':5, 'num_epochs':1, 'gpu':0}, model=model)
|
||||
mycnn_runner2.run()
|
||||
|
||||
analysis_plan = {
|
||||
'Selector':{
|
||||
'task': task,
|
||||
'header':['fedavg'],
|
||||
'filter':{'M':['MyCNN', 'anonymous'], 'R':5},
|
||||
'legend_with':['M']
|
||||
},
|
||||
'Painter':{
|
||||
'Curve':[
|
||||
{'args':{'x': 'communication_round', 'y':'val_loss'}, 'fig_option':{'title':'valid loss on MNIST'}},
|
||||
{'args':{'x': 'communication_round', 'y':'val_accuracy'}, 'fig_option':{'title':'valid accuracy on MNIST'}},
|
||||
]
|
||||
}
|
||||
}
|
||||
flgo.experiment.analyzer.show(analysis_plan)
|
||||
```
|
||||
|
||||
|
||||
|
||||

|
||||
|
||||
|
||||
|
||||
|
||||
|
||||

|
|
@ -0,0 +1,92 @@
|
|||
|
||||
# 1.6 Logger Customization
|
||||
|
||||
This section introduces the usage of `Logger`. `Logger` is responsble for recording running-time variables of interest that can be very dependent on personal usage. We offer three key APIs to support different experiment purposes.
|
||||
|
||||
|
||||
```python
|
||||
import flgo.experiment.logger as fel
|
||||
class Logger(fel.BasicLogger):
|
||||
def initialize(self):
|
||||
# initialize necessary variables BEFORE training starts
|
||||
pass
|
||||
|
||||
def log_once(self):
|
||||
# will be carried out every K communication rounds DURING training process
|
||||
pass
|
||||
|
||||
def organize_output(self):
|
||||
# organize output AFTER training ends
|
||||
pass
|
||||
```
|
||||
|
||||
The three APIs are respectively responsible for customized operations before\during\after training. All the variables of interest should be recorded into `self.output` that will be finally saved as .json file. `self.output` is of type collections.defaultdict, and the default value of each key is an empty list. Now we take the following example to show how to customize `Logger`.
|
||||
|
||||
## 1.6.1 Example: Customization on Logger
|
||||
|
||||
|
||||
```python
|
||||
import collections
|
||||
import numpy as np
|
||||
import copy
|
||||
|
||||
class MyLogger(fel.BasicLogger):
|
||||
def initialize(self, *args, **kwargs):
|
||||
self.optimal_model = copy.deepcopy(self.coordinator.model)
|
||||
self.optimal_test_loss = 9999
|
||||
|
||||
def log_once(self):
|
||||
# evaluation on testing data
|
||||
test_metric = self.coordinator.test()
|
||||
for met_name, met_val in test_metric.items():
|
||||
self.output['test_' + met_name].append(met_val)
|
||||
# check whether the current model is the optimal
|
||||
if test_metric['loss']<self.optimal_test_loss:
|
||||
self.optimal_test_loss = test_metric['loss']
|
||||
self.optimal_model.load_state_dict(self.coordinator.model.state_dict())
|
||||
self.show_current_output()
|
||||
|
||||
def organize_output(self):
|
||||
super().organize_output()
|
||||
# evaluation on clients' validation datasets
|
||||
all_metrics = collections.defaultdict(list)
|
||||
for c in self.participants:
|
||||
client_metrics = c.test(self.optimal_model, 'val')
|
||||
for met_name, met_val in client_metrics.items():
|
||||
all_metrics[met_name].append(met_val)
|
||||
for met_name, metval in all_metrics.items():
|
||||
self.output[met_name] = metval
|
||||
# compute the optimal\worst 30% metrics on validation datasets
|
||||
met_name = 'loss'
|
||||
all_valid_losses = sorted(all_metrics[met_name])
|
||||
k1 = int(0.3*len(self.participants))
|
||||
k2 = int(0.7*len(self.participants))
|
||||
self.output['worst_30_valid_loss'] = 1.0*sum(all_valid_losses[k2:])/k1
|
||||
self.output['best_30_valid_loss'] = 1.0*sum(all_valid_losses[:k1])/k1
|
||||
|
||||
import flgo.algorithm.fedavg as fedavg
|
||||
import flgo.algorithm.qfedavg as qfedavg
|
||||
import os
|
||||
task = './test_synthetic' # this task has been generated in Sec.1.3.1
|
||||
|
||||
# running optimization
|
||||
op = {'num_rounds':30, 'num_epochs':1, 'batch_size':8, 'learning_rate':0.1, 'proportion':1.0 ,'gpu':0, 'algo_para':0.1}
|
||||
fedavg_runner = flgo.init(task, fedavg, option = op, Logger=MyLogger)
|
||||
qffl_runner = flgo.init(task, qfedavg, option=op, Logger=MyLogger)
|
||||
fedavg_runner.run()
|
||||
qffl_runner.run()
|
||||
|
||||
# Result analysis
|
||||
import flgo.experiment.analyzer as al
|
||||
records = al.Selector({'task':task, 'header':['fedavg', 'qfedavg_q0.1',], 'filter':{'R':30, 'E':1, 'B':8, 'LR':0.1,'P':1.0}}).records[task]
|
||||
for rec in records:
|
||||
wl = rec.data['worst_30_valid_loss']
|
||||
bl = rec.data['best_30_valid_loss']
|
||||
print('{}:(Worst is {}, Best is {})'.format(rec.data['option']['algorithm'], wl, bl))
|
||||
```
|
||||
|
||||
fedavg:(Worst is 1.5370861026975844, Best is 0.15324175854523978)
|
||||
qfedavg:(Worst is 1.5319330559836493, Best is 0.4078656468126509)
|
||||
|
||||
|
||||
The results tells that qfedavg has a superior performance for the worst 30% clients but sacrifies model performance for the optimal 30% clients.
|
|
@ -0,0 +1 @@
|
|||
This section introduces basic configurations in our framework (i.e. parameters of `flgo.init`).
|
|
@ -0,0 +1,252 @@
|
|||
In this section, we first introduce the general paradigm of horizontal FL and then discuss the corresponding implementation in FLGo.
|
||||
|
||||
# 2.1.1 Classical Paradigm
|
||||
|
||||
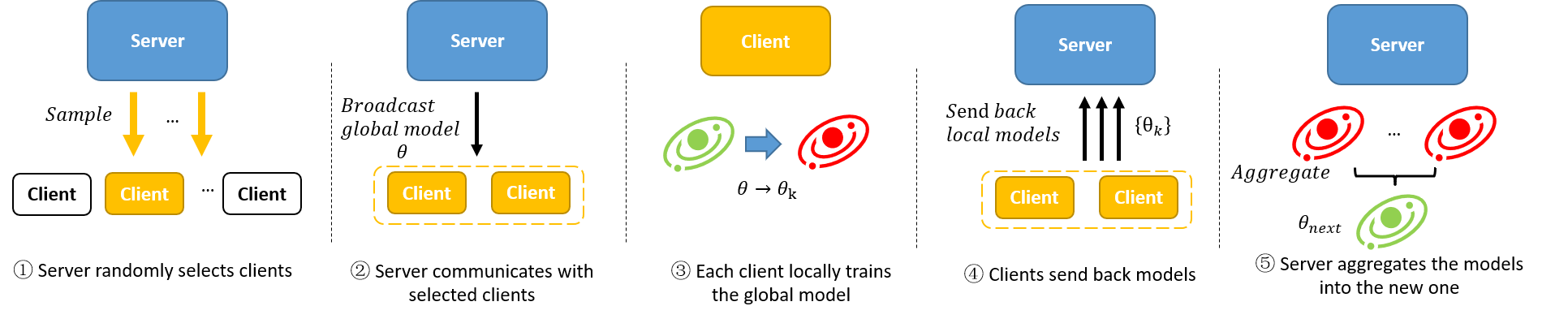
|
||||
|
||||
In a classical horizontal FL scene, there is a center server that coordinates clients to collaboratively train a global model iteratively. In each iteration, the server first samples a subset from all the clients. Then, the server broadcasts the global model the selected clients. After receiving the global model, the clients locally train it with local data. Finally, the clients send back the updated models to the server and the server aggregates the models into the new global model. The whole process is as shown in the figure above. Existing methods usually improve one or more of the five steps to realize various purposes like fairness and robustness.
|
||||
|
||||
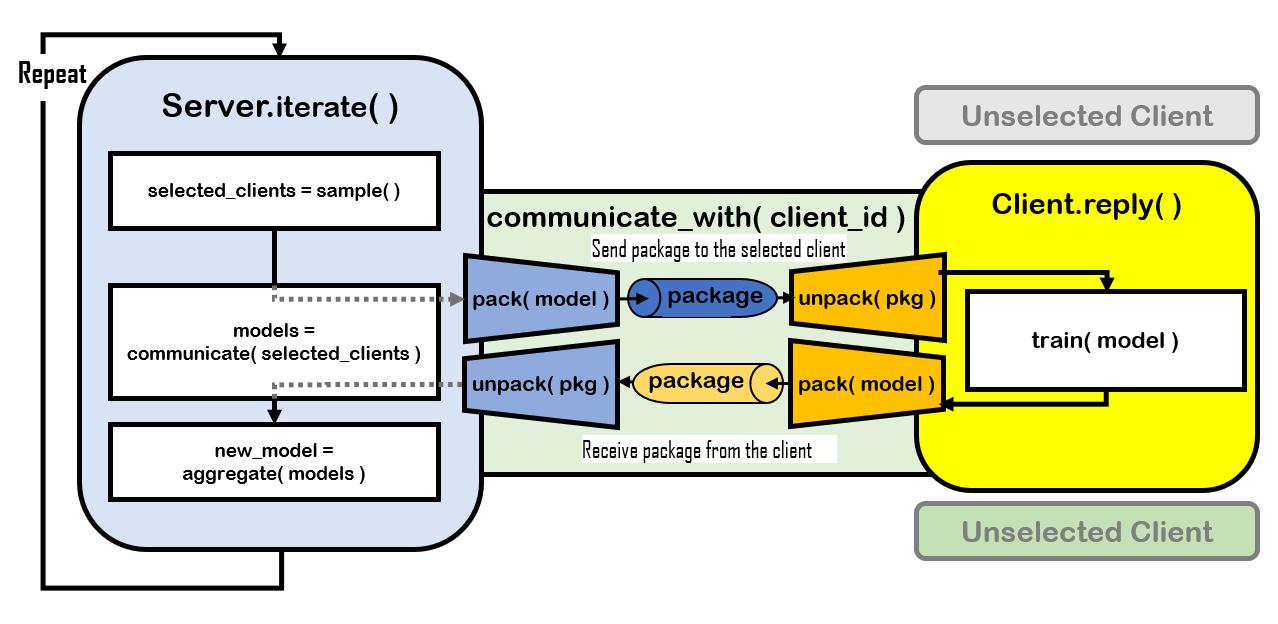
|
||||
|
||||
The cooresponding implementation of the FL process is shown in Figure 2. We use `iterate` function to model the behaviors of the server and `reply` function to model the behaviors of clients when being selected.
|
||||
|
||||
# 2.1.2 Details of Steps
|
||||
|
||||
## Server's Behavior: Server.iterate
|
||||
|
||||
The training process starts with `run` method of the server, which starts iterations (i.e. communication rounds) by using a loop. In each iteration of the loop, the server will call `iterate` to carry out each step. A standard implementation of `iterate` (i.e. `flgo.algorithm.fedbase.iterate`) is as below:
|
||||
|
||||
|
||||
```python
|
||||
def iterate(self):
|
||||
"""
|
||||
The standard iteration of each federated communication round that contains three
|
||||
necessary procedure in FL: client selection, communication and model aggregation.
|
||||
|
||||
Returns:
|
||||
False if the global model is not updated in this iteration
|
||||
"""
|
||||
# sample clients: Uniform sampling as default
|
||||
self.selected_clients = self.sample()
|
||||
# training
|
||||
models = self.communicate(self.selected_clients)['model']
|
||||
# aggregate: pk = ni/sum(ni) as default
|
||||
self.model = self.aggregate(models)
|
||||
return len(models) > 0
|
||||
```
|
||||
|
||||
## ① Server.sample
|
||||
|
||||
During each iteration, the server first sample clients by calling `self.sample()`, which returns a list of clients' IDs. We implement three sampling strategies in our preset sampling method as below. `full` sampling means selecting all the clients. `uniform` sampling means selecting clients uniformly without replacement. `md` sampling means selecting clients with replacement by probabilities w.r.t. the ratio of data sizes. Improvement on sampling strategies can be adapted here by overwriting `sample`.
|
||||
|
||||
|
||||
```python
|
||||
def sample(self):
|
||||
r"""
|
||||
Sample the clients. There are three types of sampling manners:
|
||||
full sample, uniform sample without replacement, and MDSample
|
||||
with replacement. Particularly, if 'available' is in self.sample_option,
|
||||
the server will only sample from currently available clients.
|
||||
|
||||
Returns:
|
||||
a list of the ids of the selected clients
|
||||
|
||||
Example:
|
||||
```python
|
||||
>>> selected_clients=self.sample()
|
||||
>>> selected_clients
|
||||
>>> # The selected_clients is a list of clients' ids
|
||||
```
|
||||
"""
|
||||
all_clients = self.available_clients if 'available' in self.sample_option else [cid for cid in
|
||||
range(self.num_clients)]
|
||||
# full sampling with unlimited communication resources of the server
|
||||
if 'full' in self.sample_option:
|
||||
return all_clients
|
||||
# sample clients
|
||||
elif 'uniform' in self.sample_option:
|
||||
# original sample proposed by fedavg
|
||||
selected_clients = list(
|
||||
np.random.choice(all_clients, min(self.clients_per_round, len(all_clients)), replace=False)) if len(
|
||||
all_clients) > 0 else []
|
||||
elif 'md' in self.sample_option:
|
||||
# the default setting that is introduced by FedProx, where the clients are sampled with the probability in proportion to their local data sizes
|
||||
local_data_vols = [self.clients[cid].datavol for cid in all_clients]
|
||||
total_data_vol = sum(local_data_vols)
|
||||
p = np.array(local_data_vols) / total_data_vol
|
||||
selected_clients = list(np.random.choice(all_clients, self.clients_per_round, replace=True, p=p)) if len(
|
||||
all_clients) > 0 else []
|
||||
return selected_clients
|
||||
```
|
||||
|
||||
## ② Communication- Broadcast: Server.pack & Client.unpack
|
||||
|
||||
The communication process is realized by the method `communicate(client_ids: list[int], mtype: str, asynchronous: bool)`, which contains a full ask&reply process between the server and the clients. The second step only refers to the broadcast-communication, which only describes what the server transmitting to the clients. Therefore, we use two method, `Server.pack(client_id)` and `Client.unpack()` to model the broadcast-communication process
|
||||
|
||||
|
||||
```python
|
||||
class Server:
|
||||
def pack(self, client_id, mtype=0, *args, **kwargs):
|
||||
"""
|
||||
Pack the necessary information for the client's local training.
|
||||
Any operations of compression or encryption should be done here.
|
||||
:param
|
||||
client_id: the id of the client to communicate with
|
||||
:return
|
||||
a dict that only contains the global model as default.
|
||||
"""
|
||||
return {
|
||||
"model" : copy.deepcopy(self.model),
|
||||
}
|
||||
|
||||
class Client:
|
||||
def unpack(self, received_pkg):
|
||||
"""
|
||||
Unpack the package received from the server
|
||||
:param
|
||||
received_pkg: a dict contains the global model as default
|
||||
:return:
|
||||
the unpacked information that can be rewritten
|
||||
"""
|
||||
# unpack the received package
|
||||
return received_pkg['model']
|
||||
```
|
||||
|
||||
The transmitted package should be a `dict` in python. The server will send a copy of the global model, and the client will unpack the package to obtain the global model as default. Any changes on the content of the down-streaming packages should be implemented here.
|
||||
|
||||
## Clients' Behavior: Client.reply
|
||||
|
||||
After clients receiving the global models, the method `Client.reply` will automatically be triggered to model the clients' behaviors. The implementation of `reply` is as follows:
|
||||
|
||||
|
||||
```python
|
||||
def reply(self, svr_pkg):
|
||||
r"""
|
||||
Reply a package to the server. The whole local procedure should be defined here.
|
||||
The standard form consists of three procedure: unpacking the
|
||||
server_package to obtain the global model, training the global model,
|
||||
and finally packing the updated model into client_package.
|
||||
|
||||
Args:
|
||||
svr_pkg (dict): the package received from the server
|
||||
|
||||
Returns:
|
||||
client_pkg (dict): the package to be send to the server
|
||||
"""
|
||||
model = self.unpack(svr_pkg)
|
||||
self.train(model)
|
||||
cpkg = self.pack(model)
|
||||
return cpkg
|
||||
```
|
||||
|
||||
## ③ Local Training: Client.train
|
||||
|
||||
The local training is made by the method `Client.train`, which receives a global model as the input and trains it with local data. Any modification on local training procedures should be implemented here. The default implementation is as follow:
|
||||
|
||||
|
||||
```python
|
||||
def train(self, model):
|
||||
r"""
|
||||
Standard local training procedure. Train the transmitted model with
|
||||
local training dataset.
|
||||
|
||||
Args:
|
||||
model (FModule): the global model
|
||||
"""
|
||||
model.train()
|
||||
optimizer = self.calculator.get_optimizer(model, lr=self.learning_rate, weight_decay=self.weight_decay,
|
||||
momentum=self.momentum)
|
||||
for iter in range(self.num_steps):
|
||||
# get a batch of data
|
||||
batch_data = self.get_batch_data()
|
||||
model.zero_grad()
|
||||
# calculate the loss of the model on batched dataset through task-specified calculator
|
||||
loss = self.calculator.compute_loss(model, batch_data)['loss']
|
||||
loss.backward()
|
||||
if self.clip_grad>0:torch.nn.utils.clip_grad_norm_(parameters=model.parameters(), max_norm=self.clip_grad)
|
||||
optimizer.step()
|
||||
return
|
||||
```
|
||||
|
||||
Particularly, we let the task-spefific calculation be transparent to the optimization algorithms. Therefore, one algorithm (e.g. FedAvg) can be adapted to different types of tasks without any changes. `calculator` is responsible for all the task-specific calculations.
|
||||
|
||||
## ④ Communication - Upload: Client.pack & Server.unpack
|
||||
|
||||
The communication of uploading models from clients is modeled by `Client.pack(*args, **kwargs)` and `Server.unpack(packages_list)`, which is similar to the step ②. Different from ②, the server as the receiver needs to simultaneously handle a list of packages from different clients. We let `Server.unpack` return the values in the uploaded packages as a dict that shares the same keys with each client's pakcage. Modification on the content of upload-communication should be implemented in `Client.pack` that returns a dict as a package each time.
|
||||
|
||||
|
||||
```python
|
||||
class Server:
|
||||
def unpack(self, packages_received_from_clients):
|
||||
"""
|
||||
Unpack the information from the received packages. Return models and losses as default.
|
||||
:param
|
||||
packages_received_from_clients:
|
||||
:return:
|
||||
res: collections.defaultdict that contains several lists of the clients' reply
|
||||
"""
|
||||
if len(packages_received_from_clients)==0: return collections.defaultdict(list)
|
||||
res = {pname:[] for pname in packages_received_from_clients[0]}
|
||||
for cpkg in packages_received_from_clients:
|
||||
for pname, pval in cpkg.items():
|
||||
res[pname].append(pval)
|
||||
return res
|
||||
|
||||
class Client:
|
||||
def pack(self, model, *args, **kwargs):
|
||||
"""
|
||||
Packing the package to be send to the server. The operations of compression
|
||||
of encryption of the package should be done here.
|
||||
:param
|
||||
model: the locally trained model
|
||||
:return
|
||||
package: a dict that contains the necessary information for the server
|
||||
"""
|
||||
return {
|
||||
"model" : model,
|
||||
}
|
||||
```
|
||||
|
||||
## ⑤ Model Aggregation: Server.aggregate()
|
||||
|
||||
The server finally aggregates the received models into a new global model by the method `Server.aggregate(models: list)`. There are four preset aggregation modes in our implementation. And using the normalized ratios of local data sizes (i.e. FedAvg) is set the default aggregatino option.
|
||||
|
||||
|
||||
```python
|
||||
def aggregate(self, models: list, *args, **kwargs):
|
||||
"""
|
||||
Aggregate the locally improved models.
|
||||
:param
|
||||
models: a list of local models
|
||||
:return
|
||||
the averaged result
|
||||
pk = nk/n where n=self.data_vol
|
||||
K = |S_t|
|
||||
N = |S|
|
||||
-------------------------------------------------------------------------------------------------------------------------
|
||||
weighted_scale |uniform (default) |weighted_com (original fedavg) |other
|
||||
==========================================================================================================================
|
||||
N/K * Σpk * model_k |1/K * Σmodel_k |(1-Σpk) * w_old + Σpk * model_k |Σ(pk/Σpk) * model_k
|
||||
"""
|
||||
if len(models) == 0: return self.model
|
||||
local_data_vols = [c.datavol for c in self.clients]
|
||||
total_data_vol = sum(local_data_vols)
|
||||
if self.aggregation_option == 'weighted_scale':
|
||||
p = [1.0 * local_data_vols[cid] /total_data_vol for cid in self.received_clients]
|
||||
K = len(models)
|
||||
N = self.num_clients
|
||||
return fmodule._model_sum([model_k * pk for model_k, pk in zip(models, p)]) * N / K
|
||||
elif self.aggregation_option == 'uniform':
|
||||
return fmodule._model_average(models)
|
||||
elif self.aggregation_option == 'weighted_com':
|
||||
p = [1.0 * local_data_vols[cid] / total_data_vol for cid in self.received_clients]
|
||||
w = fmodule._model_sum([model_k * pk for model_k, pk in zip(models, p)])
|
||||
return (1.0-sum(p))*self.model + w
|
||||
else:
|
||||
p = [1.0 * local_data_vols[cid] / total_data_vol for cid in self.received_clients]
|
||||
sump = sum(p)
|
||||
p = [pk/sump for pk in p]
|
||||
return fmodule._model_sum([model_k * pk for model_k, pk in zip(models, p)])
|
||||
```
|
||||
|
||||
We will show how to modify each steps to realize different algorithms by the following sections.
|
|
@ -0,0 +1,196 @@
|
|||
# 2.2.1 Example: FedProx
|
||||
|
||||
In this section, we discuss how to realize ideas with modification on the local training phase in FL. We take the method FedProx as the example. [FedProx](https://link.zhihu.com/?target=https%3A//arxiv.org/abs/1812.06127) is proposed by Li Tian in 2018 and accepted by MLSys2020. It addresses the data and system heterogeneity problem in FL, which has made two major improvements over FedAvg:
|
||||
|
||||
- **Sample & Aggregation**: sample clients by the probability w.r.t. the ratios of local data sizes (i.e. MD sampling) and uniformly aggregates the received models (i.e. uniform aggregation)
|
||||
- **Local Training**: optimize a proxy $L'$ of original local objective by additionally adding proximal term on it
|
||||
|
||||
$$L'=L+\frac{\mu}{2}\|w_{k,i}^t-w_{global}^t\|_2^2$$
|
||||
|
||||
where $k$ denoting the $k$th client, $t$ denoting the communication round, and $i$ denoting the $i$th local training iterations. $\mu$ is the hyper-parameter of FedProx.
|
||||
|
||||
# 2.2.2 Implementation
|
||||
|
||||
Since we have already implemented MD sampling and uniform aggregation as preset options, we only consider how to customize the local training process here.
|
||||
|
||||
## 2.2.2.1 Add hyper-parameter
|
||||
|
||||
We provide the API `Server.init_algo_para(algo_para: dict)` for adding additional algorightm-specific hyper-parameters. The definition of the method is as follows:
|
||||
|
||||
|
||||
```python
|
||||
def init_algo_para(self, algo_para: dict):
|
||||
"""
|
||||
Initialize the algorithm-dependent hyper-parameters for the server and all the clients.
|
||||
|
||||
Args:
|
||||
algo_paras (dict): the dict that defines the hyper-parameters (i.e. name, value and type) for the algorithm.
|
||||
|
||||
Example:
|
||||
```python
|
||||
>>> # s is an instance of Server and s.clients are instances of Client
|
||||
>>> s.u # will raise error
|
||||
>>> [c.u for c in s.clients] # will raise errors too
|
||||
>>> s.init_algo_para({'u': 0.1})
|
||||
>>> s.u # will be 0.1
|
||||
>>> [c.u for c in s.clients] # will be [0.1, 0.1,..., 0.1]
|
||||
```
|
||||
Note:
|
||||
Once `option['algo_para']` is not `None`, the value of the pre-defined hyperparameters will be replaced by the list of values in `option['algo_para']`,
|
||||
which requires the length of `option['algo_para']` is equal to the length of `algo_paras`
|
||||
"""
|
||||
...
|
||||
```
|
||||
|
||||
The key-value pairs in `algo_para` corresponds to the names of the hyper-parameters and their defalut values. After calling this method, instances of both Server and Client can directly access the hyper-parameter by self.parameter_name. An example is as shown in the definition. This method is usually called in the `initialize` method of the server. Now we add the hyper-parameter $\mu$ for FedProx and set its default value as 0.1.
|
||||
|
||||
|
||||
```python
|
||||
import flgo.algorithm.fedbase as fedbase
|
||||
import flgo.utils.fmodule as fmodule
|
||||
|
||||
class Server(fedbase.BasicServer):
|
||||
def initialize(self, *args, **kwargs):
|
||||
# set hyper-parameters
|
||||
self.init_algo_para({'mu':0.01})
|
||||
# set sampling option and aggregation option
|
||||
self.sample_option = 'md'
|
||||
self.aggregation_option = 'uniform'
|
||||
```
|
||||
|
||||
## 2.2.2.2 Modify local objective
|
||||
|
||||
|
||||
```python
|
||||
import copy
|
||||
import torch
|
||||
|
||||
class Client(fedbase.BasicClient):
|
||||
@fmodule.with_multi_gpus
|
||||
def train(self, model):
|
||||
# record the global parameters
|
||||
src_model = copy.deepcopy(model)
|
||||
# freeze gradients on the copy of global parameters
|
||||
src_model.freeze_grad()
|
||||
# start local training
|
||||
model.train()
|
||||
optimizer = self.calculator.get_optimizer(model, lr=self.learning_rate, weight_decay=self.weight_decay, momentum=self.momentum)
|
||||
for iter in range(self.num_steps):
|
||||
# get a batch of data
|
||||
batch_data = self.get_batch_data()
|
||||
model.zero_grad()
|
||||
# compute the loss of the model on batched dataset through task-specified calculator
|
||||
loss = self.calculator.compute_loss(model, batch_data)['loss']
|
||||
# compute the proximal term
|
||||
loss_proximal = 0
|
||||
for pm, ps in zip(model.parameters(), src_model.parameters()):
|
||||
loss_proximal += torch.sum(torch.pow(pm - ps, 2))
|
||||
loss = loss + 0.5 * self.mu * loss_proximal
|
||||
loss.backward()
|
||||
optimizer.step()
|
||||
return
|
||||
```
|
||||
|
||||
## 2.2.2.3 Create new class fedprox
|
||||
|
||||
Implement FedProx as a new class like
|
||||
|
||||
|
||||
```python
|
||||
class my_fedprox:
|
||||
Server = Server
|
||||
Client = Client
|
||||
```
|
||||
|
||||
# 2.2.3 Experiment
|
||||
|
||||
Now let's take a look on the experimental results on the `fedprox`. We consider the experimental settings in Sec.1.3.1.
|
||||
|
||||
|
||||
```python
|
||||
import flgo
|
||||
import os
|
||||
# generate federated task
|
||||
task = './test_synthetic'
|
||||
config = {'benchmark':{'name':'flgo.benchmark.synthetic_regression', 'para':{'alpha':0.5, 'beta':0.5, 'num_clients':30}}}
|
||||
if not os.path.exists(task): flgo.gen_task(config, task_path = task)
|
||||
|
||||
# running methods
|
||||
import flgo.algorithm.fedavg as fedavg
|
||||
option = {'num_rounds':200, 'num_epochs':5, 'batch_size':10, 'learning_rate':0.1, 'gpu':0}
|
||||
fedavg_runner = flgo.init(task, fedavg, option=option)
|
||||
my_fedprox_runner = flgo.init(task, my_fedprox, option=option)
|
||||
fedavg_runner.run()
|
||||
my_fedprox_runner.run()
|
||||
|
||||
# show results
|
||||
import flgo.experiment.analyzer
|
||||
analysis_plan = {
|
||||
'Selector':{
|
||||
'task': task,
|
||||
'header':['fedavg', 'my_fedprox_mu0.01'],
|
||||
'filter':{'R':200}
|
||||
},
|
||||
'Painter':{
|
||||
'Curve':[
|
||||
{'args':{'x': 'communication_round', 'y':'test_loss'}, 'fig_option':{'title':'test loss on Synthetic'}},
|
||||
{'args':{'x': 'communication_round', 'y':'test_accuracy'}, 'fig_option':{'title':'test accuracy on Synthetic'}},
|
||||
]
|
||||
}
|
||||
}
|
||||
flgo.experiment.analyzer.show(analysis_plan)
|
||||
```
|
||||
|
||||
|
||||
|
||||
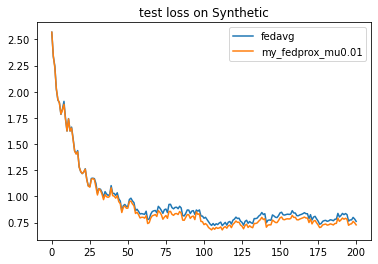
|
||||
|
||||
|
||||
|
||||
|
||||
|
||||
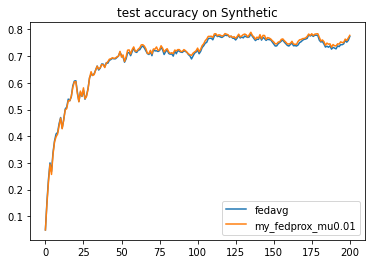
|
||||
|
||||
|
||||
|
||||
### 2.2.3.1 Change values of hyper-parameters
|
||||
|
||||
We change the value of hyper-parameter $\mu$ by specifying the keyword `algo_para` in option
|
||||
|
||||
|
||||
```python
|
||||
option01 = {'algo_para':0.1, 'num_rounds':200, 'num_epochs':5, 'batch_size':10, 'learning_rate':0.1, 'gpu':0}
|
||||
option10 = {'algo_para':10.0, 'num_rounds':200, 'num_epochs':5, 'batch_size':10, 'learning_rate':0.1, 'gpu':0}
|
||||
my_fedprox001_runner = flgo.init(task, my_fedprox, option=option01)
|
||||
my_fedprox001_runner.run()
|
||||
my_fedprox100_runner = flgo.init(task, my_fedprox, option=option10)
|
||||
my_fedprox100_runner.run()
|
||||
analysis_plan = {
|
||||
'Selector':{
|
||||
'task': task,
|
||||
'header':['fedavg', 'my_fedprox'],
|
||||
'filter':{'R':200}
|
||||
},
|
||||
'Painter':{
|
||||
'Curve':[
|
||||
{'args':{'x': 'communication_round', 'y':'test_loss'}, 'fig_option':{'title':'test loss on Synthetic'}},
|
||||
{'args':{'x': 'communication_round', 'y':'test_accuracy'}, 'fig_option':{'title':'test accuracy on Synthetic'}},
|
||||
]
|
||||
}
|
||||
}
|
||||
flgo.experiment.analyzer.show(analysis_plan)
|
||||
```
|
||||
|
||||
|
||||
|
||||
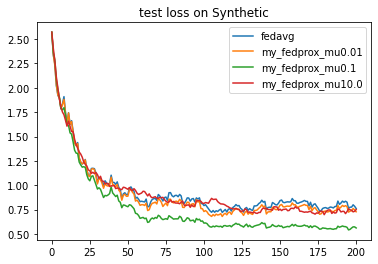
|
||||
|
||||
|
||||
|
||||
|
||||
|
||||
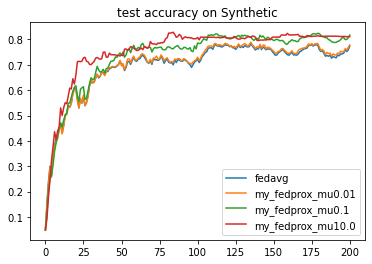
|
||||
|
||||
|
||||
|
||||
The results suggest that increasing $\mu$ significantly improves the performance of FedProx on this task.
|
|
@ -0,0 +1,131 @@
|
|||
# Example: FedMGDA+
|
||||
|
||||
This section describes how to use FLGo to implement the algorithm that makes changes during the aggregation phase. The example used here is FedMGDA+, an algorithm proposed by Hu et al. in 2020 and published in IEEE Transactions on Network Science and Engineering 2022. Inspired by the Multi-Gradient Descent Algorithm (MGDA) in multi-objective optimization, it applies MGDA to the aggregation stage of FL, so as to prevent the aggregated model update from harming the interests of any party (i.e. the inner product of the global update amount and any user’s update amount is non-negative).
|
||||
|
||||
Compared to FedAvg, FedMGDA+ only differs in the aggregation stage. It finds an aggregation weight that deconflict the global model update and local model updates by solving the following problem
|
||||
|
||||
$$\mathbf{\lambda}^*=\min_{\mathbf{\lambda}}||\sum_{i\in \mathbb{S}_t}\lambda_i d_i||_2\\s.t. \|\mathbf{\lambda}-\mathbf{\lambda_0}\|_{\infty}\le \epsilon, \mathbf{1}^\top\mathbf{\lambda}=1$$
|
||||
|
||||
where $\Delta\theta_i = \theta^t-\theta^{t+1}_i, d_i=\frac{\Delta\theta_i}{\|\Delta\theta_i\|}$
|
||||
|
||||
Then, update the global model with the global learning rate
|
||||
|
||||
$$\theta^{t+1}=\theta^t-\eta \sum_{i} \lambda_i d_i$$
|
||||
|
||||
# Implementation
|
||||
|
||||
First notice that the FedMGDA+ algorithm has two hyperparameters: $\eta$ and $\epsilon$, so add the algorithm hyperparameters in the initialization method initialize; For the aggregation part, the aggregate function receives the parameter as models by default, so the steps to implement aggregate are strictly followed by the above three steps. Note that the part of optimizing the weights is left to the self.optim_lambda method implementation, which receives the current set of gradients, the original weights, and the use of the hyperparameter self.eta to find the optimal weights (the implementation of the methods of reading optim_lambda and quadprog can be skipped for now, which are unrelated to the main flow of FLGo). The CVXOPT library (which can be installed via pip install CVXOPT) is used here to solve the minimum norm problem in step 2. If the cvxopt library is not installed, run the following command to install it:
|
||||
|
||||
|
||||
```python
|
||||
!pip install cvxopt
|
||||
```
|
||||
|
||||
The code of FedMGDA+ is as follows:
|
||||
|
||||
|
||||
```python
|
||||
from flgo.utils import fmodule
|
||||
from flgo.algorithm.fedbase import BasicServer
|
||||
from flgo.algorithm.fedavg import Client
|
||||
import flgo
|
||||
import os
|
||||
import numpy as np
|
||||
import copy
|
||||
import cvxopt
|
||||
|
||||
class Server(BasicServer):
|
||||
def initialize(self, *args, **kwargs):
|
||||
# init hyper-parameters
|
||||
self.init_algo_para({'eta':1.0, 'epsilon':0.1})
|
||||
|
||||
##############################################################
|
||||
########## Overwrite the aggregate method#####################
|
||||
def aggregate(self, models: list, *args, **kwargs):
|
||||
# 1. calculate normalized gradients
|
||||
grads = [self.model - w for w in models]
|
||||
for gi in grads: gi.normalize()
|
||||
|
||||
# 2. calculate λ0
|
||||
nks = [len(self.clients[cid].train_data) for cid in self.received_clients]
|
||||
nt = sum(nks)
|
||||
lambda0 = [1.0 * nk / nt for nk in nks]
|
||||
# 3. optimize lambdas to minimize ||λ'g||² s.t. λ∈Δ, ||λ - λ0||∞ <= ε
|
||||
op_lambda = self.optim_lambda(grads, lambda0)
|
||||
op_lambda = [ele[0] for ele in op_lambda]
|
||||
|
||||
# 4. aggregate grads
|
||||
dt = fmodule._model_average(grads, op_lambda)
|
||||
return self.model - dt * self.eta
|
||||
|
||||
def optim_lambda(self, grads, lambda0):
|
||||
# create H_m*m = 2J'J where J=[grad_i]_n*m
|
||||
n = len(grads)
|
||||
Jt = []
|
||||
for gi in grads:
|
||||
Jt.append((copy.deepcopy(fmodule._modeldict_to_tensor1D(gi.state_dict())).cpu()).numpy())
|
||||
Jt = np.array(Jt)
|
||||
# target function
|
||||
P = 2 * np.dot(Jt, Jt.T)
|
||||
|
||||
q = np.array([[0] for i in range(n)])
|
||||
# equality constraint λ∈Δ
|
||||
A = np.ones(n).T
|
||||
b = np.array([1])
|
||||
# boundary
|
||||
lb = np.array([max(0, lambda0[i] - self.epsilon) for i in range(n)])
|
||||
ub = np.array([min(1, lambda0[i] + self.epsilon) for i in range(n)])
|
||||
G = np.zeros((2*n,n))
|
||||
for i in range(n):
|
||||
G[i][i]=-1
|
||||
G[n+i][i]=1
|
||||
h = np.zeros((2*n,1))
|
||||
for i in range(n):
|
||||
h[i] = -lb[i]
|
||||
h[n+i] = ub[i]
|
||||
res=self.quadprog(P, q, G, h, A, b)
|
||||
return res
|
||||
|
||||
def quadprog(self, P, q, G, h, A, b):
|
||||
"""
|
||||
Input: Numpy arrays, the format follows MATLAB quadprog function: https://www.mathworks.com/help/optim/ug/quadprog.html
|
||||
Output: Numpy array of the solution
|
||||
"""
|
||||
P = cvxopt.matrix(P.tolist())
|
||||
q = cvxopt.matrix(q.tolist(), tc='d')
|
||||
G = cvxopt.matrix(G.tolist())
|
||||
h = cvxopt.matrix(h.tolist())
|
||||
A = cvxopt.matrix(A.tolist())
|
||||
b = cvxopt.matrix(b.tolist(), tc='d')
|
||||
sol = cvxopt.solvers.qp(P, q.T, G.T, h.T, A.T, b)
|
||||
return np.array(sol['x'])
|
||||
```
|
||||
|
||||
|
||||
```python
|
||||
# Construct FedMGDA+
|
||||
class fedmgda:
|
||||
Server = Server
|
||||
Client = Client
|
||||
```
|
||||
|
||||
# Experiment
|
||||
|
||||
|
||||
```python
|
||||
import flgo.algorithm.fedavg as fedavg
|
||||
task = './test_cifar10'
|
||||
config = {'benchmark':{'name':'flgo.benchmark.cifar10_classification'},'partitioner':{'name': 'DiversityPartitioner','para':{'num_clients':100, 'diversity':0.2}}}
|
||||
if not os.path.exists(task): flgo.gen_task(config, task_path = task)
|
||||
option = {'learning_rate':0.01, 'num_steps':5, 'num_rounds':200,'gpu':0}
|
||||
|
||||
fedavg_runner = flgo.init(task, fedavg, option=option)
|
||||
fedmgda_runner_eta1epsilon01 = flgo.init(task, fedmgda, option=option)
|
||||
fedmgda_runner_eta05epsilon01 = flgo.init(task, fedmgda, option={'learning_rate':0.01, 'num_steps':5, 'num_rounds':200,'gpu':0, 'algo_para':[0.5, 0.1]})
|
||||
fedmgda_runner_eta01epsilon01 = flgo.init(task, fedmgda, option={'learning_rate':0.01, 'num_steps':5, 'num_rounds':200,'gpu':0, 'algo_para':[0.1, 0.1]})
|
||||
|
||||
fedavg_runner.run()
|
||||
fedmgda_runner_eta1epsilon01.run()
|
||||
fedmgda_runner_eta05epsilon01.run()
|
||||
fedmgda_runner_eta01epsilon01.run()
|
||||
```
|
|
@ -0,0 +1,54 @@
|
|||
# Example: PowerOfChoice
|
||||
|
||||
This section describes how to use FLGo to implement algorithms that make changes during the user sampling phase. The example used here is PowerOfChoice, an algorithm proposed by Cho et al. in 2020 ([link to paper](https://arxiv.org/abs/2010.01243)). Compared with the traditional unbiased sampling strategy, this method uses a biased but faster convergence sampling method, that is, it preferentially samples those users with large losses of local datasets. To achieve this, its sampling steps are summarized as follows:
|
||||
|
||||
1. The server does not put back the sampled $d$ candidate users from all $K$ users according to the size ratio of the dataset ($m<=d<=K$, $m$ is the actual number of sampled users in the current round of the server);
|
||||
2. The server broadcasts the current global model $\theta^t$ to $d$ candidate users, evaluates their local dataset loss, and these users send back the loss value $F_k(\theta^t)$;
|
||||
3. The server sorts according to the loss value sent back by $d$ candidate users, and preferentially selects the first $m$ users with the largest loss to participate in this round of training
|
||||
|
||||
The following describes how to implement this sampling strategy with FLGo.
|
||||
|
||||
|
||||
```python
|
||||
import numpy as np
|
||||
import flgo.algorithm.fedavg as fedavg
|
||||
from flgo.algorithm.fedbase import BasicServer
|
||||
import flgo.system_simulator.base as ss
|
||||
import os
|
||||
import flgo
|
||||
|
||||
class Server(BasicServer):
|
||||
def initialize(self, *args, **kwargs):
|
||||
self.init_algo_para({'d': self.num_clients})
|
||||
|
||||
def sample(self):
|
||||
# create candidate set A
|
||||
num_candidate = min(self.d, len(self.available_clients))
|
||||
p_candidate = np.array([len(self.clients[cid].train_data) for cid in self.available_clients])
|
||||
candidate_set = np.random.choice(self.available_clients, num_candidate, p=p_candidate / p_candidate.sum(), replace=False)
|
||||
candidate_set = sorted(candidate_set)
|
||||
# communicate with the candidates for their local loss
|
||||
losses = []
|
||||
for cid in candidate_set:
|
||||
losses.append(self.clients[cid].test(self.model, dataflag='train')['loss'])
|
||||
# sort candidate set according to their local loss value, and choose the top-M highest ones
|
||||
sort_id = np.array(losses).argsort().tolist()
|
||||
sort_id.reverse()
|
||||
num_selected = min(self.clients_per_round, len(self.available_clients))
|
||||
selected_clients = np.array(self.available_clients)[sort_id][:num_selected]
|
||||
return selected_clients.tolist()
|
||||
|
||||
class powerofchoice:
|
||||
Server=Server
|
||||
Client=fedavg.Client
|
||||
```
|
||||
|
||||
First, the algorithm has a hyperparameter d to control the number of candidates, so implement the hyperparameter in the initialization method initialize and set the default value to the total number of users;
|
||||
|
||||
Then, the number of candidates is determined to be the smaller value of the current active user and d, and the candidate_set of the candidate set is obtained by sampling according to the size ratio of its dataset.
|
||||
|
||||
Then, for convenience, instead of rewriting communication-related content, directly call the candidates' test functions to obtain their local dataset loss (the two effects are equivalent, and rewriting communication-related code is more troublesome). The set of candidates is then sorted based on loss.
|
||||
|
||||
Finally, the top self.clients_per_round users with the most losses are selected and their IDs are returned.
|
||||
|
||||
Note: The decorator of the sample method is ss.with_availability to instantly refresh the user's usability, and this function is to achieve system heterogeneity, which will be explained in subsequent chapters
|
|
@ -0,0 +1,106 @@
|
|||
# Example: q-FFL
|
||||
|
||||
This section describes how to use FLGo to implement algorithms that make changes during the communication phase. An example is used here of a method that has only been modified less in the communication phase, qffl, proposed by Li Tian et al. in 2019 and published in ICLR 2020 ([link to paper](https://arxiv.org/abs/1905.10497)), which aims to improve the fairness of federated learning. The following explains how to implement the algorithm with FLGo.
|
||||
|
||||
|
||||
|
||||
The algorithm is inspired by load balancing in the network and proposes a fairer optimization goal:
|
||||
|
||||
$$\min_w f_q(w)=\sum_{k=1}^m \frac{p_k}{q 1}F_k^{q 1}(w)$$
|
||||
|
||||
where $q$ is an artificially set hyperparameter, $F_k(w)$ is the local loss of user $k$, and $p_k$ is the original objective function weight of user $k$.
|
||||
By observing the above goal, it can be found that as long as $q>0$, each user's loss in that goal $F'_k=\frac{F_k^{q 1}}{q 1}$ will have the property that as $F_k$ increases, $F'_k$ increases rapidly (greater than the growth rate of $F_k$), so that the global objective function $f_q$ also increases rapidly. Therefore, in order to prevent $f_q$ from skyrocketing, optimizing the objective function will be forced to automatically balance the loss value of different users to prevent the occurrence of any larger value, where $q$ determines the growth rate of $F'_k$, and the larger the $q$, the stronger the fairness.
|
||||
|
||||
In order to optimize this fairness objective function, the authors propose the q-FedAVG algorithm, the core steps of which are as follows:
|
||||
|
||||
1. After user $k$ receives the global model, use the global model $w^t$ to evaluate the loss of the local training set, and obtain $F_k(w^t)$;
|
||||
|
||||
2. User $k$ trains the global model, obtains $\bar{w}_k^{t 1}$, and calculates the following variables:
|
||||
|
||||
$$\Delta w_k^t=L(w^t-\bar{w}_k^{t 1})\approx\frac{1}{\eta}(w^t-\bar{w}_k^{t 1})\\\Delta_k^t=F_k^q(w^t) \Delta w_k^t\\h_k^t=qF_k^{q-1}(w^t)\|\Delta w_k^t\|^2 LF_k^q(w^t)$$
|
||||
|
||||
3. Users upload $h_k^t$ and $\Delta_k^t$;
|
||||
|
||||
4. The global model for server aggregation is:
|
||||
|
||||
$$w^{t 1}=w^t-\frac{\sum_{k\in S_t}\Delta_k^t}{\sum_{k\in S_t}h_k^t}$$
|
||||
|
||||
# Implementation
|
||||
|
||||
Compared with the global model of fedavg communication, qffl communicates $h_k^t$ and $\Delta_k^t$, so complete the calculation of these two items in the pack function local of the client and modify the returned dictionary. In contrast, there are more than models in the package received by the server, so the keywords (dk and hk) are used to take out the results in the package, and the aggregation strategy is directly adjusted to the form of qffl in the iterate.
|
||||
|
||||
|
||||
|
||||
```python
|
||||
import flgo
|
||||
import flgo.algorithm.fedbase as fedbase
|
||||
import torch
|
||||
import flgo.utils.fmodule as fmodule
|
||||
import flgo.algorithm.fedavg as fedavg
|
||||
import copy
|
||||
import os
|
||||
|
||||
class Client(fedbase.BasicClient):
|
||||
def unpack(self, package):
|
||||
model = package['model']
|
||||
self.global_model = copy.deepcopy(model)
|
||||
return model
|
||||
|
||||
def pack(self, model):
|
||||
Fk = self.test(self.global_model, 'train')['loss']+1e-8
|
||||
L = 1.0/self.learning_rate
|
||||
delta_wk = L*(self.global_model - model)
|
||||
dk = (Fk**self.q)*delta_wk
|
||||
hk = self.q*(Fk**(self.q-1))*(delta_wk.norm()**2) + L*(Fk**self.q)
|
||||
self.global_model = None
|
||||
return {'dk':dk, 'hk':hk}
|
||||
|
||||
class Server(fedbase.BasicServer):
|
||||
def initialize(self, *args, **kwargs):
|
||||
self.init_algo_para({'q': 1.0})
|
||||
|
||||
def iterate(self):
|
||||
self.selected_clients = self.sample()
|
||||
res = self.communicate(self.selected_clients)
|
||||
self.model = self.model - fmodule._model_sum(res['dk'])/sum(res['hk'])
|
||||
return len(self.received_clients)>0
|
||||
|
||||
class qffl:
|
||||
Server = Server
|
||||
Client = Client
|
||||
|
||||
```
|
||||
|
||||
# Experiment
|
||||
|
||||
|
||||
```python
|
||||
task = './synthetic11_client100'
|
||||
config = {'benchmark':{'name':'flgo.benchmark.synthetic_regression', 'para':{'alpha':1, 'beta':1, 'num_clients':100}}}
|
||||
if not os.path.exists(task): flgo.gen_task(config, task_path = task)
|
||||
option = {'num_rounds':2000, 'num_epochs':1, 'batch_size':10, 'learning_rate':0.1, 'gpu':0, 'proportion':0.1,'lr_scheduler':0}
|
||||
fedavg_runner = flgo.init(task, fedavg, option=option)
|
||||
qffl_runner = flgo.init(task, qffl, option=option)
|
||||
fedavg_runner.run()
|
||||
qffl_runner.run()
|
||||
```
|
||||
|
||||
|
||||
```python
|
||||
analysis_on_q = {
|
||||
'Selector':{
|
||||
'task': task,
|
||||
'header':['fedavg','qffl' ]
|
||||
},
|
||||
'Painter':{
|
||||
'Curve':[
|
||||
{'args':{'x': 'communication_round', 'y':'test_accuracy'}, 'fig_option':{'title':'test accuracy on Synthetic(1,1)'}},
|
||||
{'args':{'x': 'communication_round', 'y':'std_valid_loss'}, 'fig_option':{'title':'std_valid_loss on Synthetic(1,1)'}},
|
||||
{'args':{'x': 'communication_round', 'y':'mean_valid_accuracy'}, 'fig_option':{'title':'mean valid accuracy on Synthetic(1,1)'}},
|
||||
|
||||
|
||||
]
|
||||
}
|
||||
}
|
||||
flgo.experiment.analyzer.show(analysis_on_q)
|
||||
```
|
|
@ -0,0 +1,3 @@
|
|||
In this section, we first discuss the general paradigm of horizontal FL and the corresponding implementation.
|
||||
Then, we take several existing federated algorithms as examples to demonstrate customization processes during different stages of training.
|
||||
We finally list other FL paradigm across scenes (e.g. vertical FL, decentralized FL, and hierarchical FL).
|
|
@ -0,0 +1,74 @@
|
|||
# Example: MNIST
|
||||
|
||||
### 1. Prepare the following dataset configuration file `my_dataset.py` (remark: The name can be arbitrarily set)
|
||||
|
||||
The constructed dataset configuration `.py` file needs to have variables: `train_data`, `test_data` (optional), and function `get_model()`
|
||||
|
||||
|
||||
|
||||
```python
|
||||
import os
|
||||
import torchvision
|
||||
import torch
|
||||
|
||||
transform = torchvision.transforms.Compose(
|
||||
[torchvision.transforms.ToTensor(),
|
||||
torchvision.transforms.Normalize((0.1307,), (0.3081,))]
|
||||
)
|
||||
path = os.path.join(os.path.dirname(os.path.abspath(__file__)))
|
||||
# Define Variable: train_data
|
||||
train_data = torchvision.datasets.MNIST(root=path, train=True, download=True, transform=transform)
|
||||
# Define Variable: test_data
|
||||
test_data = torchvision.datasets.MNIST(root=path, train=False, download=True, transform=transform)
|
||||
|
||||
class mlp(torch.nn.Module):
|
||||
def __init__(self):
|
||||
super(mlp, self).__init__()
|
||||
self.fc1 = torch.nn.Linear(784, 200)
|
||||
self.fc2 = torch.nn.Linear(200, 200)
|
||||
self.fc3 = torch.nn.Linear(200, 10)
|
||||
self.relu = torch.nn.ReLU()
|
||||
|
||||
def forward(self, x):
|
||||
x = x.view(-1, x.shape[1] * x.shape[-2] * x.shape[-1])
|
||||
x = self.relu(self.fc1(x))
|
||||
x = self.relu(self.fc2(x))
|
||||
x = self.fc3(x)
|
||||
return x
|
||||
|
||||
# Define Model: get_model()
|
||||
def get_model():
|
||||
return mlp()
|
||||
```
|
||||
|
||||
### 2. Using `flgo.gen_benchmark` to generate benchmark from configuration
|
||||
|
||||
|
||||
```python
|
||||
import flgo
|
||||
import os
|
||||
bmkname = 'my_mnist_classification' # the name of benchmark
|
||||
bmk_config = './my_dataset.py' # the path of the configuration file
|
||||
# Constructing benchmark by flgo.gen_benchmark
|
||||
if not os.path.exists(bmkname):
|
||||
bmk = flgo.gen_benchmark_from_file(bmkname, bmk_config, target_path='.', data_type='cv', task_type='classification')
|
||||
print(bmk)
|
||||
```
|
||||
|
||||
### 3. Test
|
||||
|
||||
Use the constructed benchmark as other plugins.
|
||||
|
||||
|
||||
```python
|
||||
import flgo.algorithm.fedavg as fedavg
|
||||
bmk = 'my_mnist_classification'
|
||||
task = './my_mnist'
|
||||
task_config = {
|
||||
'benchmark':bmk,
|
||||
}
|
||||
if not os.path.exists(task): flgo.gen_task(task_config, task_path=task)
|
||||
# 运行fedavg算法
|
||||
runner = flgo.init(task, fedavg, {'gpu':[0,],'log_file':True, 'num_steps':5, 'num_rounds':3})
|
||||
runner.run()
|
||||
```
|
|
@ -0,0 +1,240 @@
|
|||
# Overview
|
||||
The supported types of fast-customization-on-task are listed below:
|
||||
|
||||
- classification
|
||||
- detection
|
||||
- segmentation
|
||||
|
||||
The dependency is
|
||||
|
||||
- torchvision
|
||||
|
||||
Now we introduce each type of task by examples.
|
||||
|
||||
# Classification
|
||||
## 1. Format of Configuration File
|
||||
| **Name** | **Type** | **Required** | **Description** | **Remark** |
|
||||
|------------|----------|--------------|-----------------|------------|
|
||||
| train_data | | True | | - |
|
||||
| get_model | | True | | - |
|
||||
| test_data | | False | | |
|
||||
| val_data | | False | | |
|
||||
|
||||
|
||||
**Example:**
|
||||
```python
|
||||
import os
|
||||
import torchvision
|
||||
import flgo.benchmark
|
||||
|
||||
transform = torchvision.transforms.Compose([torchvision.transforms.ToTensor(), torchvision.transforms.Normalize((0.485,0.456,0.406), (0.229,0.224,0.225))])
|
||||
root = os.path.join(flgo.benchmark.path,'RAW_DATA', 'SVHN') # 可以为任意存放原始数据的绝对路径
|
||||
train_data = torchvision.datasets.SVHN(root=root,transform=transform, download=True, split='train')
|
||||
test_data = torchvision.datasets.SVHN(root=root, transform=transform, download=True, split='test')
|
||||
|
||||
class CNN(torch.nn.Module):
|
||||
def __init__(self):
|
||||
super(CNN, self).__init__()
|
||||
self.embedder = torch.nn.Sequential(
|
||||
torch.nn.Conv2d(3, 64, 5),
|
||||
torch.nn.ReLU(),
|
||||
torch.nn.MaxPool2d(2),
|
||||
torch.nn.Conv2d(64, 64, 5),
|
||||
torch.nn.ReLU(),
|
||||
torch.nn.MaxPool2d(2),
|
||||
torch.nn.Flatten(1),
|
||||
torch.nn.Linear(1600, 384),
|
||||
torch.nn.ReLU(),
|
||||
torch.nn.Linear(384, 192),
|
||||
torch.nn.ReLU(),
|
||||
)
|
||||
self.fc = torch.nn.Linear(192, 10)
|
||||
|
||||
def forward(self, x):
|
||||
x = self.embedder(x)
|
||||
return self.fc(x)
|
||||
|
||||
def get_model():
|
||||
return CNN()
|
||||
```
|
||||
## 2. Construction
|
||||
```python
|
||||
import flgo
|
||||
|
||||
# create svhn_classification
|
||||
bmk = flgo.gen_benchmark_from_file(
|
||||
benchmark='svhn_classification',
|
||||
config_file='./config_svhn.py',
|
||||
target_path='.',
|
||||
data_type='cv',
|
||||
task_type='classification',
|
||||
)
|
||||
|
||||
# Generate IID federated task
|
||||
task = './test_svhn' # task name
|
||||
task_config = {
|
||||
'benchmark': bmk,
|
||||
'partitioner':{
|
||||
'name':'IIDPartitioner'
|
||||
}
|
||||
} # task configuration
|
||||
flgo.gen_task(task_config, task) # generate task
|
||||
|
||||
# run fedavg
|
||||
import flgo.algorithm.fedavg as fedavg
|
||||
runner = flgo.init(task, fedavg) # init fedavg runner
|
||||
runner.run() # run
|
||||
```
|
||||
# Detection
|
||||
## 1. Format of Configuration File
|
||||
| **Name** | **Type** | **Required** | **Description** | **Remark** |
|
||||
|------------|----------|--------------|-----------------|------------|
|
||||
| train_data | | True | | - |
|
||||
| get_model | | True | | - |
|
||||
| test_data | | False | | |
|
||||
| val_data | | False | | |
|
||||
|
||||
**Example:**
|
||||
```python
|
||||
import torch
|
||||
import torchvision.models
|
||||
import torchvision.transforms as T
|
||||
import flgo.benchmark
|
||||
from torchvision.models.detection.faster_rcnn import FastRCNNPredictor
|
||||
import torchvision.datasets
|
||||
import os
|
||||
# 0. preprocess VOC
|
||||
CLASSES = (
|
||||
'__background__',
|
||||
"aeroplane",
|
||||
"bicycle",
|
||||
"bird",
|
||||
"boat",
|
||||
"bottle",
|
||||
"bus",
|
||||
"car",
|
||||
"cat",
|
||||
"chair",
|
||||
"cow",
|
||||
"diningtable",
|
||||
"dog",
|
||||
"horse",
|
||||
"motorbike",
|
||||
"person",
|
||||
"pottedplant",
|
||||
"sheep",
|
||||
"sofa",
|
||||
"train",
|
||||
"tvmonitor",
|
||||
)
|
||||
CLASSES_MAP = {name:idx for idx, name in enumerate(CLASSES)}
|
||||
def voc_target_transform(y):
|
||||
objects = y['annotation']['object']
|
||||
boxes = [torch.FloatTensor([int(v) for v in obj['bndbox'].values()]) for obj in objects]
|
||||
labels = [torch.LongTensor(torch.LongTensor([CLASSES_MAP[obj['name'].lower()]])) for obj in objects]
|
||||
return {'boxes': torch.stack(boxes), 'labels':torch.cat(labels)}
|
||||
transform = T.Compose([T.ToTensor(), T.Normalize([0.485, 0.456, 0.406], [0.229, 0.224, 0.225])])
|
||||
root = os.path.join(flgo.benchmark.path, 'RAW_DATA', 'VOC')
|
||||
|
||||
# 1. define train_data and test_data
|
||||
train_data = torchvision.datasets.VOCDetection(root=root, download=True, image_set='trainval', year='2007', transform=transform, target_transform=voc_target_transform)
|
||||
test_data = torchvision.datasets.VOCDetection(root=root, download=True, image_set='test', year='2007', transform=transform, target_transform=voc_target_transform)
|
||||
train_data.num_classes = len(CLASSES)
|
||||
test_data.num_classes = len(CLASSES)
|
||||
|
||||
# 2. define get_model()
|
||||
def get_model():
|
||||
model = torchvision.models.detection.fasterrcnn_resnet50_fpn(weights=torchvision.models.detection.FasterRCNN_ResNet50_FPN_Weights.COCO_V1)
|
||||
in_features = model.roi_heads.box_predictor.cls_score.in_features
|
||||
model.roi_heads.box_predictor = FastRCNNPredictor(in_features, len(CLASSES))
|
||||
return model
|
||||
```
|
||||
## 2. Construction
|
||||
```python
|
||||
import flgo
|
||||
|
||||
# create voc_detection
|
||||
bmk = flgo.gen_benchmark_from_file(
|
||||
benchmark='voc_detection',
|
||||
config_file='./config_voc.py',
|
||||
target_path='.',
|
||||
data_type='cv',
|
||||
task_type='detection'
|
||||
)
|
||||
|
||||
# generate federated task
|
||||
task = './my_IID_voc'
|
||||
task_config = {
|
||||
'benchmark':bmk,
|
||||
}
|
||||
flgo.gen_task(task_config, task_path=task)
|
||||
|
||||
# run fedavg
|
||||
import flgo.algorithm.fedavg as fedavg
|
||||
runner = flgo.init(task, fedavg, {'gpu':[0,],'log_file':True, 'learning_rate':0.0001,'num_epochs':1, 'batch_size':2, 'num_rounds':100, 'proportion':1.0, 'test_batch_size':2, 'train_holdout':0,'eval_interval':1,})
|
||||
runner.run()
|
||||
```
|
||||
|
||||
# Segmentation
|
||||
## 1. Format of Configuration File
|
||||
| **Name** | **Type** | **Required** | **Description** | **Remark** |
|
||||
|------------|----------|--------------|-----------------|------------|
|
||||
| train_data | | True | | - |
|
||||
| get_model | | True | | - |
|
||||
| test_data | | False | | |
|
||||
| val_data | | False | | |
|
||||
|
||||
|
||||
**Example:**
|
||||
```python
|
||||
import os
|
||||
import torchvision
|
||||
import torch
|
||||
import torchvision.transforms as T
|
||||
|
||||
transform = T.Compose([
|
||||
T.ToTensor(),
|
||||
T.Normalize((0.5, 0.5, 0.5), (0.5, 0.5, 0.5))
|
||||
])
|
||||
target_transform = T.Compose([
|
||||
T.PILToTensor(),
|
||||
T.Lambda(lambda x: (x-1).squeeze().type(torch.LongTensor))
|
||||
])
|
||||
path = os.path.join(os.path.dirname(os.path.abspath(__file__)))
|
||||
# define train_data
|
||||
train_data = torchvision.datasets.OxfordIIITPet(root=path, split='trainval', download=True, target_types='segmentation', transform=transform, target_transform=target_transform)
|
||||
# define test_data
|
||||
test_data = torchvision.datasets.OxfordIIITPet(root=path, split='test', download=True, target_types='segmentation', transform=transform, target_transform=target_transform)
|
||||
# set num_classes=2 (i.e. classes in dataset) + 1 (i.e. background)
|
||||
train_data.num_classes = 3
|
||||
test_data.num_classes = 3
|
||||
|
||||
def get_model():
|
||||
model = torchvision.models.segmentation.fcn_resnet50(num_classes=3)
|
||||
return model
|
||||
```
|
||||
## 2. Construction
|
||||
```python
|
||||
import flgo
|
||||
|
||||
# create oxfordiiitpet_segmentation
|
||||
bmk = flgo.gen_benchmark_from_file(
|
||||
benchmark='oxfordiiitpet_segmentation',
|
||||
config_file='./config_oxford.py',
|
||||
target_path='.',
|
||||
data_type='cv',
|
||||
task_type='segmentation'
|
||||
)
|
||||
|
||||
# generate federated task
|
||||
task = './my_IID_oxford'
|
||||
task_config = {
|
||||
'benchmark':bmk,
|
||||
}
|
||||
flgo.gen_task(task_config, task_path=task)
|
||||
|
||||
# run fedavg
|
||||
import flgo.algorithm.fedavg as fedavg
|
||||
runner = flgo.init(task, fedavg, {'gpu':0,'log_file':True, 'learning_rate':0.01, 'num_steps':1, 'batch_size':2, 'num_rounds':1000, 'test_batch_size':8, 'train_holdout':0})
|
||||
runner.run()
|
||||
```
|
|
@ -0,0 +1,20 @@
|
|||
# Overview
|
||||
The supported types of fast-customization-on-task are listed below:
|
||||
|
||||
- classification
|
||||
- translation
|
||||
- language modeling
|
||||
|
||||
The dependency is
|
||||
|
||||
- torchtext
|
||||
- torchdata
|
||||
|
||||
Now we introduce each type of task by examples.
|
||||
|
||||
# Classification
|
||||
|
||||
# Translation
|
||||
|
||||
# Language Modeling
|
||||
|
|
@ -0,0 +1,19 @@
|
|||
# Overview
|
||||
The supported types of fast-customization-on-task are listed below:
|
||||
|
||||
- graph classification
|
||||
- node classification
|
||||
- link prediction
|
||||
-
|
||||
The dependency is
|
||||
|
||||
- PyG
|
||||
|
||||
Now we introduce each type of task by examples.
|
||||
|
||||
# Graph Classification
|
||||
|
||||
# Node Classification
|
||||
|
||||
# Link Prediction
|
||||
|
|
@ -0,0 +1,3 @@
|
|||
```python
|
||||
|
||||
```
|
|
@ -0,0 +1,3 @@
|
|||
```python
|
||||
|
||||
```
|
|
@ -0,0 +1,148 @@
|
|||
# 1 Introduction to Data Heterogeneity
|
||||
|
||||
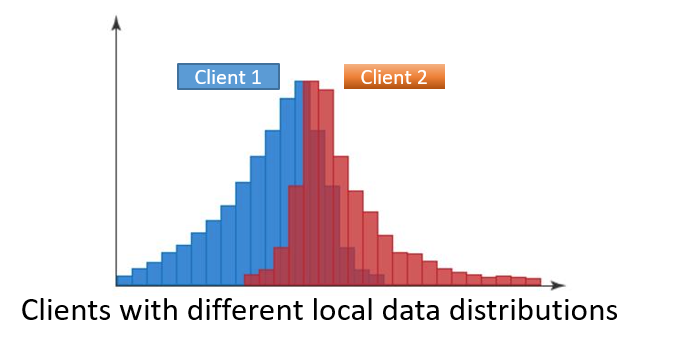
|
||||
|
||||
Data heterogeneity in federated learning refers to different users holding data with different distributions (i.e. non-I.I.D., data non independent identical distribution). The common data heterogeneity in experiments in existing federated learning papers is mainly the following two categories:
|
||||
|
||||
- **Label Skew**: Different users hold different distribution of tags, for example, in the federal handwritten digit classification task, some users only hold a sample of the number 1;
|
||||
- **Feature Skew**: different users hold different distributions of features, such as different users holding different styles of handwritten digital images 1;
|
||||
|
||||
In addition to these two isomerities, there are **Concept Drift** (i.e. different X corresponds to the same Y) and **Concept Shift** (i.e. the same X corresponds to different Y), which are less conducted in experiments by previous works. Different types of heterogeneity generally accompany each other. For example, in the above label heterogeneous example, users with local labels with only the number 1 also hold images with only the number 1 feature.
|
||||
|
||||
# 2 Customization on Data Heterogeneity
|
||||
|
||||
## General Process of FLGo
|
||||
|
||||
In FLGo, the process of generating and running each federation task can be simply thought of as the following 4 steps:
|
||||
|
||||
1. **Load**: Load the original dataset
|
||||
2. **Partition**: *Divide the original dataset (**customizing here**)*
|
||||
3. **Save**: Save the division information
|
||||
4. **Running-time Reload**: Load raw data and partitioning information, and recover the divided dataset.
|
||||
|
||||
For the vast majority of datasets, custom data heterogeneity depends on how the datasets are divided. We let class `Partitioner` be responsible for the partition step, and its instance method `__call__(data: Any)->List[List[int]]` will partition the indices of samples in the original datasets.
|
||||
|
||||
|
||||
```python
|
||||
import flgo.benchmark.partition as fbp
|
||||
from typing import *
|
||||
|
||||
class MyPartitioner(fbp.BasicPartitioner):
|
||||
def __call__(self, data:Any):
|
||||
r"""The partitioned results should be of type List[List[int]],
|
||||
where each list element saves the partitioning information for a client.
|
||||
"""
|
||||
local_datas = []
|
||||
return local_datas
|
||||
```
|
||||
|
||||
## Example 1: Imbalanced IID Partitioner on MNIST
|
||||
|
||||
|
||||
```python
|
||||
import flgo.benchmark.mnist_classification
|
||||
import flgo.benchmark.partition as fbp
|
||||
import numpy as np
|
||||
# 1. Define the partitioner
|
||||
class MyIIDPartitioner(fbp.BasicPartitioner):
|
||||
def __init__(self, samples_per_client=[15000, 15000, 15000, 15000]):
|
||||
self.samples_per_client = samples_per_client
|
||||
self.num_clients = len(samples_per_client)
|
||||
|
||||
def __call__(self, data):
|
||||
# 1.1 shuffle the indices of samples
|
||||
d_idxs = np.random.permutation(len(data))
|
||||
# 1.2 Divide all the indices into num_clients shards
|
||||
local_datas = np.split(d_idxs, np.cumsum(self.samples_per_client))[:-1]
|
||||
local_datas = [di.tolist() for di in local_datas]
|
||||
return local_datas
|
||||
|
||||
# 2. Specify the Partitioner in task configuration
|
||||
task_config = {
|
||||
'benchmark': flgo.benchmark.mnist_classification,
|
||||
'partitioner':{
|
||||
'name':MyIIDPartitioner,
|
||||
'para':{
|
||||
'samples_per_client':[5000, 14000, 19000, 22000]
|
||||
}
|
||||
}
|
||||
}
|
||||
task = 'my_test_partitioner'
|
||||
|
||||
# 3. Test it now
|
||||
flgo.gen_task(task_config, task)
|
||||
import flgo.algorithm.fedavg as fedavg
|
||||
runner = flgo.init(task, fedavg)
|
||||
runner.run()
|
||||
```
|
||||
|
||||
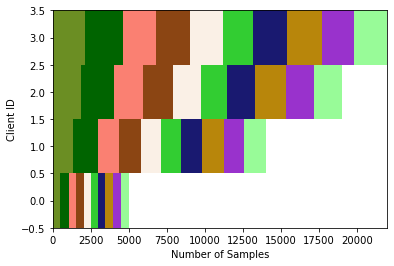
|
||||
|
||||
## Example 2: Feature Skew Partitioner
|
||||
|
||||
FLGo has integrated data partitioners commonly used in existing papers, such as IID data partitioning, diversity data partitioning, Dirichlet data partitioning, etc. These partitioners can divide specific data by specifying properties that partition dependencies in the task configuration parameters `task_config`. For example, in the MNIST handwritten digit classification task, the pixel density of different images is different. To differ the levels of pixel densities of images held by different users, you can bind all samples to a label representing the pixel density size and then have the partitioner rely on this property to divide. For all partitioners, the parameter in the constructor `index_func: X->list[Any]` specifies the basis for the partition. For example:
|
||||
|
||||
|
||||
```python
|
||||
"""
|
||||
class DiversityPartitioner(BasicPartitioner):
|
||||
def __init__(self, num_clients=100, diversity=1.0, index_func=lambda X:[xi[-1] for xi in X]):
|
||||
self.num_clients = num_clients
|
||||
self.diversity = diversity
|
||||
self.index_func = index_func # 指定按照什么属性的多样性进行划分
|
||||
...
|
||||
|
||||
class IDPartitioner(BasicPartitioner):
|
||||
def __init__(self, num_clients=-1, priority='random', index_func=lambda X:X.id):
|
||||
self.num_clients = int(num_clients)
|
||||
self.priorty = priority
|
||||
self.index_func = index_func # 指定按照什么属性为ID
|
||||
...
|
||||
|
||||
class DirichletPartitioner(BasicPartitioner):
|
||||
def __init__(self, num_clients=100, alpha=1.0, error_bar=1e-6, imbalance=0, index_func=lambda X:[xi[-1] for xi in X]):
|
||||
self.num_clients = num_clients
|
||||
self.alpha = alpha
|
||||
self.imbalance = imbalance
|
||||
self.index_func = index_func # 指定按照什么属性服从狄利克雷分布
|
||||
self.error_bar = error_bar
|
||||
"""
|
||||
```
|
||||
|
||||
`index_func` receives the same input as the `__call__` method of the corresponding partitioner, and the output is a list of the same length as data, where each element is of some attribute value (e.g. such as pixel density) of the corresponding sample. The following examples takes the pixel density of MNIST as an example to illustrate how to construct the desired data heterogeneity by specifying `index_func` function
|
||||
|
||||
|
||||
```python
|
||||
import flgo.benchmark.mnist_classification
|
||||
import flgo.benchmark.partition as fbp
|
||||
import torch
|
||||
# 1. Define index_func
|
||||
def index_func(data):
|
||||
xfeature = torch.tensor([di[0].mean() for di in data]).argsort()
|
||||
group = [-1 for _ in range(len(data))]
|
||||
gid = 0
|
||||
# Attach each sample with a label of pixel density
|
||||
num_levels = 10
|
||||
for i, did in enumerate(xfeature):
|
||||
if i >= (gid + 1) * len(data) / num_levels:
|
||||
gid += 1
|
||||
group[did] = gid
|
||||
return group
|
||||
|
||||
# 2. Spefify the Partitioner and pass the index_func
|
||||
task_config = {
|
||||
'benchmark': flgo.benchmark.mnist_classification,
|
||||
'partitioner':{
|
||||
'name':fbp.IDPartitioner, # IDPartitioner根据每个样本所属的ID直接构造用户,每个用户对应一个ID
|
||||
'para':{
|
||||
'index_func': index_func
|
||||
}
|
||||
}
|
||||
}
|
||||
task = 'my_test_partitioner2'
|
||||
# 3. test it now
|
||||
flgo.gen_task(task_config, task)
|
||||
import flgo.algorithm.fedavg as fedavg
|
||||
runner = flgo.init(task, fedavg)
|
||||
runner.run()
|
||||
```
|
|
@ -0,0 +1 @@
|
|||
This section dicsusses the customization for different types of ML tasks.
|
|
@ -0,0 +1,123 @@
|
|||
# 1 Intermittent Client Availability
|
||||
|
||||
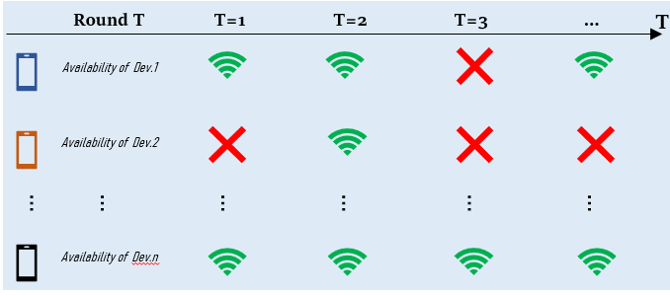
|
||||
|
||||
Classical FL ideally assumes the full client availability where all the clients are always available during the FL training process, which is inpractical in the real-world situation. To enable more sutdies on FL wiht intermittent client availability, we provide APIs for customizing different types of intermittent client availability.
|
||||
|
||||
# 2 API for Availability
|
||||
|
||||
<img src="https://raw.githubusercontent.com/WwZzz/myfigs/master/flgo_avail_shift.png" width="40%">
|
||||
|
||||
The availability of clients is described by the rule of shift of the availability state, which contains two variables for each client in `Simulator`:
|
||||
|
||||
- **prob_available** (float): range(0,1), the probability of being available from a unavailable state
|
||||
- **prob_unavailable** (float): range(0,1), the probability of being unavailable from a unavailable state
|
||||
|
||||
To set the next moment's availability, one should overwrite the method `update_client_availability(self)` of a new created `Simulator`.
|
||||
|
||||
|
||||
```python
|
||||
import flgo.simulator.base
|
||||
class MySimulator(flgo.simulator.base.BasicSimulator):
|
||||
def update_client_availabililty(self):
|
||||
"""
|
||||
Define the variable prob_unavailable and prob_available for all the clients by APIs
|
||||
self.set_variable(self.all_clients, 'prob_available', prob_values: List[float]) and
|
||||
self.set_variable(self.all_clients, 'unprob_available', prob_values: List[float]).
|
||||
If the next state of a client is deterministic, directly set the prob value to be 1 or 0.
|
||||
To fix the availability state of clients until the next aggregation round comes, set attribute
|
||||
self.round_fixed_availability=True, whose value is False as default.
|
||||
"""
|
||||
return
|
||||
```
|
||||
|
||||
Now we show how to customize client availability distribution by a simple example.
|
||||
|
||||
# 3 Example
|
||||
|
||||
|
||||
```python
|
||||
import flgo.algorithm.fedavg as fedavg
|
||||
import flgo.experiment.analyzer
|
||||
import flgo.experiment.logger as fel
|
||||
import flgo.simulator.base
|
||||
import flgo.benchmark.cifar10_classification as cifar
|
||||
import flgo.benchmark.partition as fbp
|
||||
import os
|
||||
import flgo.simulator.base
|
||||
import random
|
||||
# 1. Define two simulators for comparison
|
||||
# 1.1 The probability of stage shift are fixed as 0.1,where clients' states are hard to change
|
||||
class MySimulator(flgo.simulator.base.BasicSimulator):
|
||||
def update_client_availability(self):
|
||||
if self.gv.clock.current_time==0:
|
||||
self.set_variable(self.all_clients, 'prob_available', [1 for _ in self.clients])
|
||||
self.set_variable(self.all_clients, 'prob_unavailable', [int(random.random() >= 0.5) for _ in self.clients])
|
||||
return
|
||||
pa = [0.1 for _ in self.clients]
|
||||
pua = [0.1 for _ in self.clients]
|
||||
self.set_variable(self.all_clients, 'prob_available', pa)
|
||||
self.set_variable(self.all_clients, 'prob_unavailable', pua)
|
||||
|
||||
# 1.2 The probability of clients are fixed as 0.9, where clients' states are easy to change
|
||||
class MySimulator2(flgo.simulator.base.BasicSimulator):
|
||||
def update_client_availability(self):
|
||||
if self.gv.clock.current_time==0:
|
||||
self.set_variable(self.all_clients, 'prob_available', [1 for _ in self.clients])
|
||||
self.set_variable(self.all_clients, 'prob_unavailable', [int(random.random() >= 0.5) for _ in self.clients])
|
||||
return
|
||||
pa = [0.9 for _ in self.clients]
|
||||
pua = [0.9 for _ in self.clients]
|
||||
self.set_variable(self.all_clients, 'prob_available', pa)
|
||||
self.set_variable(self.all_clients, 'prob_unavailable', pua)
|
||||
|
||||
# 2. Generate federated task and test
|
||||
task = './IID_cifar10'
|
||||
gen_config = {
|
||||
'benchmark': cifar,
|
||||
'partitioner': fbp.IIDPartitioner
|
||||
}
|
||||
if not os.path.exists(task): flgo.gen_task(gen_config, task_path=task)
|
||||
|
||||
# 3. Customize Logger to record the availability of clients at each moment
|
||||
class MyLogger(fel.BasicLogger):
|
||||
def log_once(self, *args, **kwargs):
|
||||
if self.gv.clock.current_time==0: return
|
||||
self.output['available_clients'].append(self.coordinator.available_clients)
|
||||
print(self.output['available_clients'][-1])
|
||||
|
||||
if __name__ == '__main__':
|
||||
# 4. Respectively Run FedAvg with the two simulators
|
||||
runner1 = flgo.init(task, fedavg, {'gpu':[0,],'log_file':True, 'num_steps':1, 'num_rounds':100}, Logger=MyLogger, Simulator=MySimulator)
|
||||
runner1.run()
|
||||
runner2 = flgo.init(task, fedavg, {'gpu':[0,],'log_file':True, 'num_steps':1, 'num_rounds':100}, Logger=MyLogger, Simulator=MySimulator2)
|
||||
runner2.run()
|
||||
|
||||
# 5. visualize the availability distribution
|
||||
selector = flgo.experiment.analyzer.Selector({'task':task, 'header':['fedavg',], })
|
||||
def visualize_availability(rec_data, title = ''):
|
||||
avl_clients = rec_data['available_clients']
|
||||
all_points_x = []
|
||||
all_points_y = []
|
||||
for round in range(len(avl_clients)):
|
||||
all_points_x.extend([round + 1 for _ in avl_clients[round]])
|
||||
all_points_y.extend([cid for cid in avl_clients[round]])
|
||||
import matplotlib.pyplot as plt
|
||||
plt.scatter(all_points_x, all_points_y, s=10)
|
||||
plt.title(title)
|
||||
plt.xlabel('communication round')
|
||||
plt.ylabel('client ID')
|
||||
plt.show()
|
||||
rec0 = selector.records[task][0]
|
||||
visualize_availability(rec0.data, rec0.name[rec0.name.find('_SIM')+4:rec0.name.find('_SIM')+16])
|
||||
rec1 = selector.records[task][1]
|
||||
visualize_availability(rec1.data, rec1.name[rec1.name.find('_SIM')+4:rec1.name.find('_SIM')+16])
|
||||
```
|
||||
|
||||
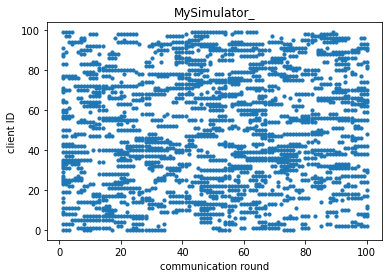
|
||||
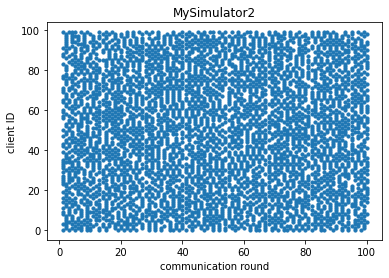
|
||||
|
||||
|
||||
```python
|
||||
|
||||
```
|
|
@ -0,0 +1,74 @@
|
|||
# Unreliable Client Connectivity
|
||||
Unreliable client connectivity means the server may lose connections accidiently to the selected clients.
|
||||
Therefore, the server cannot expect to successfully received the locally updated model from the dropped clinets.
|
||||
To model the unreliable client connectivity, FLGo allocates each client a probability of dropping out after it was selected.
|
||||
The probability can be timely changed by the `Simulator`. Now we provide examples to show how to set the dropping probability of clients.
|
||||
|
||||
To update clients' connectivity, set the `prob_drop` by method `set_variable(client_ids: List[int], var_name:str='prob_drop'', )`
|
||||
# Example
|
||||
|
||||
```python
|
||||
import numpy as np
|
||||
import flgo.simulator.base
|
||||
import flgo
|
||||
# 1. let the probability of dropping out of each client obey UNIFORM(0,0.5) at each round
|
||||
class CONSimulator(flgo.simulator.base.BasicSimulator):
|
||||
def update_client_connectivity(self, client_ids):
|
||||
self.set_variable(client_ids, 'prob_drop', np.random.uniform(0.,0.5, len(client_ids)).tolist())
|
||||
|
||||
# 2. generate task
|
||||
task = './DIR_cifar10'
|
||||
gen_config = {
|
||||
'benchmark': cifar,
|
||||
'partitioner': fbp.DirichletPartitioner
|
||||
}
|
||||
if not os.path.exists(task): flgo.gen_task(gen_config, task_path=task)
|
||||
|
||||
# 3. Log the time cost
|
||||
import flgo.experiment.logger as fel
|
||||
class MyLogger(fel.BasicLogger):
|
||||
def log_once(self, *args, **kwargs):
|
||||
self.info('Current_time:{}'.format(self.clock.current_time))
|
||||
super(MyLogger, self).log_once()
|
||||
self.output['time'].append(self.clock.current_time)
|
||||
|
||||
if __name__ == '__main__':
|
||||
# 4. set the time waiting for dropped clients to be 10 units by `set_tolerance_for_latency`
|
||||
# remark: the undropped clients will immediately return the locally trained model ideally
|
||||
# Specify Logger and Simulator respectively by their keywords
|
||||
import flgo.algorithm.fedavg as fedavg
|
||||
runner_fedavg = flgo.init(task, fedavg, {'gpu':[0,],'log_file':True, 'num_epochs':1, 'num_rounds':20}, Logger=MyLogger)
|
||||
runner_fedavg.set_tolerance_for_latency(10)
|
||||
runner_fedavg.run()
|
||||
|
||||
runner_fedavg_with_drop = flgo.init(task, fedavg, {'gpu':[0,],'log_file':True, 'num_epochs':1, 'num_rounds':20}, Logger=MyLogger, Simulator=CONSimulator)
|
||||
runner_fedavg_with_drop.set_tolerance_for_latency(10)
|
||||
runner_fedavg_with_drop.run()
|
||||
|
||||
# 5. Visualize Acc. v.s. Rounds\Time
|
||||
import flgo.experiment.analyzer
|
||||
analysis_plan = {
|
||||
'Selector': {'task': task, 'header':['fedavg',] ,'legend_with':['SIM']},
|
||||
'Painter': {
|
||||
'Curve': [
|
||||
{
|
||||
'args': {'x': 'communication_round', 'y': 'test_accuracy'},
|
||||
'obj_option': {'color': ['r', 'g', 'b', 'y', 'skyblue']},
|
||||
'fig_option': {'xlabel': 'communication round', 'ylabel': 'test_accuracy',
|
||||
'title': 'fedavg on {}'.format(task)}
|
||||
},
|
||||
{
|
||||
'args': {'x': 'time', 'y': 'test_accuracy'},
|
||||
'obj_option': {'color': ['r', 'g', 'b', 'y', 'skyblue']},
|
||||
'fig_option': {'xlabel': 'time', 'ylabel': 'test_accuracy',
|
||||
'title': 'fedavg on {}'.format(task)}
|
||||
},
|
||||
]
|
||||
},
|
||||
}
|
||||
flgo.experiment.analyzer.show(analysis_plan)
|
||||
|
||||
```
|
||||
|
||||
The results indicate that useless waiting of the server will cause time wasting.
|
||||
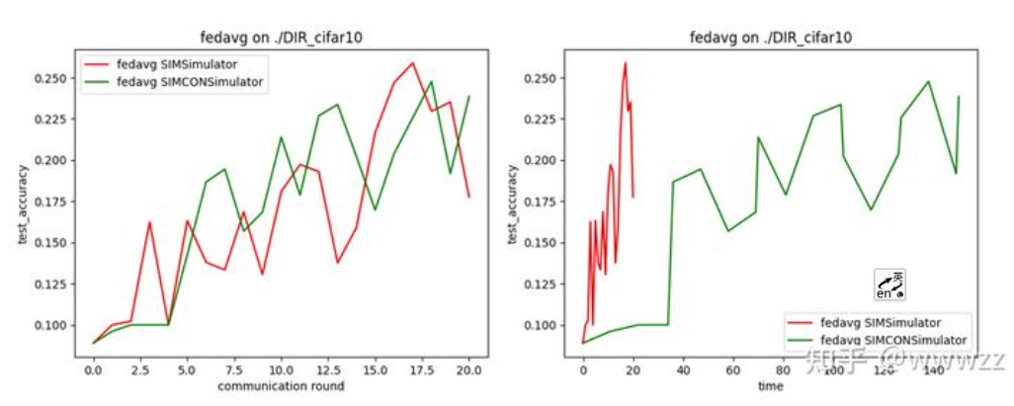
|
|
@ -0,0 +1,87 @@
|
|||
# Varying Client Responsiveness
|
||||
In FL, the communication costs and local training costs can vary across clients.
|
||||
From a view of server, it will only sense the total time costs of each client starting from it sends the global model to it.
|
||||
Therefore, we define the client responsiveness as the time interval between the server sending the global model to it and the server receiving the local results. One important impact of varying client responsiveness is that a synchronous server shuold wait for the slowest client at each communication round, resulting in large time costs.
|
||||
|
||||
In FLGo, we model the client responsiveness of all the clients by the variable `latency` in our `Simulator`.
|
||||
To update the responsiveness of the selected clients, one should change the variable by
|
||||
```python
|
||||
self.set_variable(client_ids:List[Any], var_name:str='latency', latency_values:list[int])
|
||||
```
|
||||
|
||||
Now we show the usage of setting heterogeneity in client responsiveness by the following example. To make a comparison against FedAvg, we adapt a time efficient mehtod, TierFL, to show the impact of heterogeneity.
|
||||
|
||||
# Example
|
||||
```python
|
||||
import os
|
||||
import numpy as np
|
||||
import flgo.simulator.base
|
||||
# 1. let the clients' responsing time obey UNIFORM(5,1000)
|
||||
class RSSimulator(flgo.simulator.base.BasicSimulator):
|
||||
def initialize(self):
|
||||
# init static initial reponse time
|
||||
self.client_time_response = {cid: np.random.randint(5, 1000) for cid in self.clients}
|
||||
self.set_variable(list(self.clients.keys()), 'latency', list(self.client_time_response.values()))
|
||||
|
||||
def update_client_responsiveness(self, client_ids):
|
||||
latency = [self.client_time_response[cid] for cid in client_ids]
|
||||
self.set_variable(client_ids, 'latency', latency)
|
||||
# 2. generate federated task
|
||||
import flgo.benchmark.mnist_classification as mnist
|
||||
import flgo.benchmark.partition as fbp
|
||||
task = './IID_mnist'
|
||||
gen_config = {
|
||||
'benchmark': mnist,
|
||||
'partitioner': fbp.IIDPartitioner
|
||||
}
|
||||
if not os.path.exists(task): flgo.gen_task(gen_config, task_path=task)
|
||||
|
||||
# 3. Customize Logger to record time cost
|
||||
import flgo.experiment.logger as fel
|
||||
class MyLogger(fel.BasicLogger):
|
||||
def log_once(self, *args, **kwargs):
|
||||
self.info('Current_time:{}'.format(self.clock.current_time))
|
||||
super(MyLogger, self).log_once()
|
||||
self.output['time'].append(self.clock.current_time)
|
||||
|
||||
if __name__ == '__main__':
|
||||
# 4. run fedavg and TiFL for comparison
|
||||
import flgo.algorithm.fedavg as fedavg
|
||||
import flgo.algorithm.TiFL as TiFL
|
||||
runner_fedavg = flgo.init(task, fedavg, {'gpu':[0,],'log_file':True, 'num_epochs':1, 'num_rounds':20}, Logger=MyLogger, Simulator=RSSimulator)
|
||||
runner_fedavg.run()
|
||||
runner_tifl = flgo.init(task, TiFL, {'gpu':[0,],'log_file':True, 'num_epochs':1, 'num_rounds':20}, Logger=MyLogger, Simulator=RSSimulator)
|
||||
runner_tifl.run()
|
||||
# 5. visualize the results
|
||||
import flgo.experiment.analyzer
|
||||
analysis_plan = {
|
||||
'Selector': {'task': task, 'header':['fedavg', 'TiFL'] },
|
||||
'Painter': {
|
||||
'Curve': [
|
||||
{
|
||||
'args': {'x': 'communication_round', 'y': 'test_accuracy'},
|
||||
'obj_option': {'color': ['r', 'g', 'b', 'y', 'skyblue']},
|
||||
'fig_option': {'xlabel': 'communication round', 'ylabel': 'test_accuracy',
|
||||
'title': 'fedavg\TiFL on {}'.format(task)}
|
||||
},
|
||||
{
|
||||
'args': {'x': 'time', 'y': 'test_accuracy'},
|
||||
'obj_option': {'color': ['r', 'g', 'b', 'y', 'skyblue']},
|
||||
'fig_option': {'xlabel': 'time', 'ylabel': 'test_accuracy',
|
||||
'title': 'fedavg\TiFL on {}'.format(task)}
|
||||
},
|
||||
]
|
||||
},
|
||||
'Table': {
|
||||
'max_value': [
|
||||
{'x':'test_accuracy'},
|
||||
{'x': 'time'},
|
||||
]
|
||||
},
|
||||
}
|
||||
flgo.experiment.analyzer.show(analysis_plan)
|
||||
```
|
||||
|
||||
The results suggest that TiFL save nearly 40% time costs and achieve similar performance under the same communication rounds
|
||||
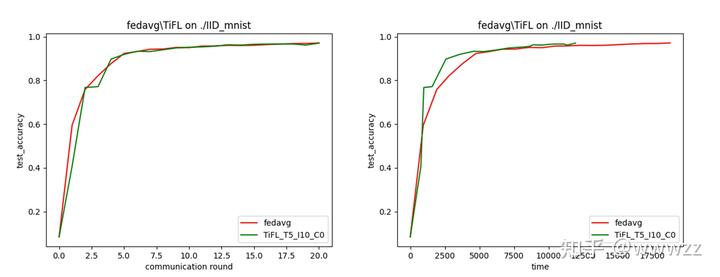
|
||||
|
|
@ -0,0 +1,78 @@
|
|||
# Incomplete Local Training
|
||||
This type of system heterogeneity means clients may not be able to finish all the local training epochs, which can cause objective inconsistency and degrade the model performance.
|
||||
To model this type of heterogeneity, we use variable `working_amount` to control the number of realistic training iterations of clients.
|
||||
Values of this variable can be arbitrarily set by API `Simulator.set_variable(client_ids: List[Any], 'working_amount', values)`
|
||||
|
||||
# Example
|
||||
We show the usage of this part by the following example. We use Synthetic dataset and compare baselines FedAvg, FedProx, and FedNova.
|
||||
```python
|
||||
import flgo.algorithm.fedavg as fedavg
|
||||
import flgo.algorithm.fedprox as fedprox
|
||||
import flgo.algorithm.fednova as fednova
|
||||
import flgo.experiment.analyzer
|
||||
import flgo.experiment.logger as fel
|
||||
import flgo.simulator.base
|
||||
import flgo.benchmark.synthetic_regression as synthetic
|
||||
import numpy as np
|
||||
import os
|
||||
import flgo.simulator.base
|
||||
# 1. Construct the Simulator
|
||||
class CMPSimulator(flgo.simulator.base.BasicSimulator):
|
||||
def update_client_completeness(self, client_ids):
|
||||
if not hasattr(self, '_my_working_amount'):
|
||||
rs = np.random.normal(1.0, 1.0, len(self.clients))
|
||||
rs = rs.clip(0.01, 2)
|
||||
self._my_working_amount = {cid:max(int(r*self.clients[cid].num_steps),1) for cid,r in zip(self.clients, rs)}
|
||||
print(self._my_working_amount)
|
||||
working_amount = [self._my_working_amount[cid] for cid in client_ids]
|
||||
self.set_variable(client_ids, 'working_amount', working_amount)
|
||||
# 2. Create federated task Synthetic(1,1)
|
||||
task = './syncthetic11'
|
||||
gen_config = {'benchmark':{'name':synthetic, 'para':{'alpha':1., 'beta':1., 'num_clients':30}}}
|
||||
if not os.path.exists(task): flgo.gen_task(gen_config, task_path=task)
|
||||
|
||||
if __name__ == '__main__':
|
||||
# 3. sequentically run different runners to eliminate the impact of randomness
|
||||
option = {'gpu':[0,],'proportion':1.0, 'log_file':True, 'num_epochs':5, 'learning_rate':0.02, 'batch_size':20, 'num_rounds':200, 'sample':'full', 'aggregate':'uniform'}
|
||||
runner_fedavg_ideal = flgo.init(task, fedavg, option, Logger=fel.BasicLogger)
|
||||
runner_fedavg_ideal.run()
|
||||
runner_fedavg_hete = flgo.init(task, fedavg, option, Simulator=CMPSimulator, Logger=fel.BasicLogger)
|
||||
runner_fedavg_hete.run()
|
||||
runner_fedprox_hete = flgo.init(task, fedprox, option, Simulator=CMPSimulator, Logger=fel.BasicLogger)
|
||||
runner_fedprox_hete.run()
|
||||
runner_fednova_hete = flgo.init(task, fednova, option, Simulator=CMPSimulator, Logger=fel.BasicLogger)
|
||||
runner_fednova_hete.run()
|
||||
# visualize the experimental result
|
||||
# 4. Visualize results
|
||||
analysis_plan = {
|
||||
'Selector': {'task': task, 'header':['fedavg', 'fedprox', 'fednova'], 'legend_with':['SIM'] },
|
||||
'Painter': {
|
||||
'Curve': [
|
||||
{
|
||||
'args': {'x': 'communication_round', 'y': 'test_loss'},
|
||||
'obj_option': {'color': ['r', 'g', 'b', 'y', 'skyblue']},
|
||||
'fig_option': {'xlabel': 'communication round', 'ylabel': 'test_loss',
|
||||
'title': 'fedavg on {}'.format(task), 'ylim':[0,2]}
|
||||
},
|
||||
{
|
||||
'args': {'x': 'communication_round', 'y': 'test_accuracy'},
|
||||
'obj_option': {'color': ['r', 'g', 'b', 'y', 'skyblue']},
|
||||
'fig_option': {'xlabel': 'communication round', 'ylabel': 'test_accuracy',
|
||||
'title': 'fedavg on {}'.format(task), 'ylim':[0.6,0.9]}
|
||||
},
|
||||
]
|
||||
},
|
||||
'Table': {
|
||||
'min_value': [
|
||||
{'x': 'train_loss'},
|
||||
{'x':'test_loss'}
|
||||
],
|
||||
'max_value': [
|
||||
{'x':'test_accuracy'}
|
||||
]
|
||||
},
|
||||
}
|
||||
flgo.experiment.analyzer.show(analysis_plan)
|
||||
```
|
||||
The results below show that FedNova achieve similar performance under severe data heterogeneity and outperforms other methods, which are consistent with results in FedNova's paper.
|
||||
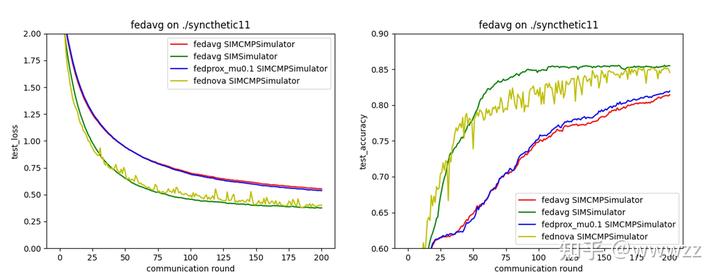
|
|
@ -0,0 +1,172 @@
|
|||
# Staleness
|
||||
|
||||
Staleness refers to when the server aggregates multiple models where there are models that were not trained on the latest model. For example, the server receives the model returned from the sampled users in the first round at the 10th aggregation round. At this point, the server can choose to discard it outright or find ways to exploit the stale information.
|
||||
In FLGo, the ability to receive stale models means that the server does not wait forever for the current sampled user to return all models before aggregating the models. Therefore, the server aggregation model needs to set a condition, that is, when the conditions are met, the server turns on model aggregation, and the next aggregation round (sampling, waiting, aggregation) is opened after aggregation. To achieve this, FLGo allows defining the behavior of the server at each moment, rather than the behavior of each turn, for more complex policy design.
|
||||
Here, I try to set up three aggregation conditions as an example and give the corresponding implementation:
|
||||
- **Cond=0:** always waiting for the selected client before aggregation
|
||||
- **Cond=1:** aggregating once the waiting time exceeds a specific value
|
||||
- **Cond=2:** aggregating once the number of received models is no smaller than K
|
||||
|
||||
The three ways will produce different degree of staleness when aggregating models, which is confirmed to harm FL performance. For example,
|
||||
the first way (i.e. cond=0) won't produce any staleness, but will largely increase the time cost at each communication round due to the waiting for the slowest clients.
|
||||
The second way costs a fixed time to wait for clients, and usually suffers non-trivial staleness. The third way will severely introduce preference towards clients that with fast responsiveness.
|
||||
Now we show how to implement the three ways in our FLGo:
|
||||
```python
|
||||
import copy
|
||||
import numpy as np
|
||||
import flgo.utils.fmodule as fmodule
|
||||
from flgo.algorithm.fedbase import BasicServer
|
||||
from flgo.algorithm.fedbase import BasicClient
|
||||
|
||||
class Server(BasicServer):
|
||||
def initialize(self, *args, **kwargs):
|
||||
self.init_algo_para({'cond': 0, 'time_budget':100, 'K':10})
|
||||
self.round_finished = True
|
||||
self.buffer = {
|
||||
'model': [],
|
||||
'round': [],
|
||||
't': [],
|
||||
'client_id':[],
|
||||
}
|
||||
self.sampling_timestamp = 0
|
||||
self.sample_option = 'uniform_available'
|
||||
|
||||
def pack(self, client_id, mtype=0, *args, **kwargs):
|
||||
return {
|
||||
'model': copy.deepcopy(self.model),
|
||||
'round': self.current_round, # model version
|
||||
}
|
||||
|
||||
def iterate(self):
|
||||
# sampling clients to start a new round \ only listening for new coming models
|
||||
if self.round_finished:
|
||||
self.selected_clients = self.sample()
|
||||
self.sampling_timestamp = self.gv.clock.current_time
|
||||
self.round_finished = False
|
||||
res = self.communicate(self.selected_clients, asynchronous=True)
|
||||
else:
|
||||
res = self.communicate([], asynchronous=True)
|
||||
if res!={}:
|
||||
self.buffer['model'].extend(res['model'])
|
||||
self.buffer['round'].extend(res['round'])
|
||||
self.buffer['t'].extend([self.gv.clock.current_time for _ in res['model']])
|
||||
self.buffer['client_id'].extend(res['__cid'])
|
||||
|
||||
if self.aggregation_condition():
|
||||
# update the global model
|
||||
stale_clients = []
|
||||
stale_rounds = []
|
||||
for cid, round in zip(self.buffer['client_id'], self.buffer['round']):
|
||||
if round<self.current_round:
|
||||
stale_clients.append(cid)
|
||||
stale_rounds.append(round)
|
||||
if len(stale_rounds)>0:
|
||||
self.gv.logger.info('Receiving stale models from clients: {}'.format(stale_clients))
|
||||
self.gv.logger.info('The staleness are {}'.format([r-self.current_round for r in stale_rounds]))
|
||||
self.gv.logger.info('Averaging Staleness: {}'.format(np.mean([r-self.current_round for r in stale_rounds])))
|
||||
self.model = fmodule._model_average(self.buffer['model'])
|
||||
self.round_finished = True
|
||||
# clear buffer
|
||||
for k in self.buffer.keys(): self.buffer[k] = []
|
||||
return self.round_finished
|
||||
|
||||
def aggregation_condition(self):
|
||||
if self.cond==0:
|
||||
for cid in self.selected_clients:
|
||||
if cid not in self.buffer['client_id']:
|
||||
# aggregate only when receiving all the packages from selected clients
|
||||
return False
|
||||
return True
|
||||
elif self.cond==1:
|
||||
# aggregate if the time budget for waiting is exhausted
|
||||
if self.gv.clock.current_time-self.sampling_timestamp>=self.time_budget or all([(cid in self.buffer['client_id']) for cid in self.selected_clients]):
|
||||
if len(self.buffer['model'])>0:
|
||||
return True
|
||||
return False
|
||||
elif self.cond==2:
|
||||
# aggregate when the number of models in the buffer is larger than K
|
||||
return len(self.buffer['client_id'])>=min(len(self.selected_clients), self.K)
|
||||
|
||||
class Client(BasicClient):
|
||||
def unpack(self, received_pkg):
|
||||
self.round = received_pkg['round']
|
||||
return received_pkg['model']
|
||||
|
||||
def pack(self, model, *args, **kwargs):
|
||||
return {
|
||||
'model': model,
|
||||
'round': self.round
|
||||
}
|
||||
|
||||
|
||||
if __name__ =='__main__':
|
||||
import flgo
|
||||
import flgo.benchmark.mnist_classification as mnist
|
||||
import os
|
||||
task = './mnist_100clients'
|
||||
if not os.path.exists(task):
|
||||
flgo.gen_task({'benchmark': mnist, 'partitioner': {'name': 'IIDPartitioner', 'para': {'num_clients': 100}}}, task)
|
||||
class algo:
|
||||
Server = Server
|
||||
Client = Client
|
||||
runner0 = flgo.init(task, algo, option={'num_rounds':10, 'algo_para':[0, 200, 0], "gpu": 0, 'proportion': 0.2, 'num_steps': 5, 'responsiveness': 'UNI-5-1000'})
|
||||
runner0.run()
|
||||
runner1 = flgo.init(task, algo, option={'num_rounds':10, 'algo_para':[1, 200, 0], "gpu": 0, 'proportion': 0.2, 'num_steps': 5, 'responsiveness': 'UNI-5-1000'})
|
||||
runner1.run()
|
||||
runner2 = flgo.init(task, algo, option={'num_rounds':10, 'algo_para':[2, 0, 10], "gpu": 0, 'proportion': 0.2, 'num_steps': 5, 'responsiveness': 'UNI-5-1000'})
|
||||
runner2.run()
|
||||
```
|
||||
|
||||
# Experiment
|
||||
We first create heterogeneity of responsiveness for different clients. Here we specify the responsing time of clients to obey distribution $UNIFORM(5,1000)$ in option by setting the keyword 'responsiveness' as 'UNI-5-1000'.
|
||||
We conduct the simple experiment on i.i.d.-partitioned MNIST with 20 clients.
|
||||
Now let's see the information of different ways at the 6th rounds :
|
||||
**Cond 0**
|
||||
2023-08-29 09:49:45,257 fedbase.py run [line:246] INFO --------------Round 6--------------
|
||||
2023-08-29 09:49:45,257 simple_logger.py log_once [line:14] INFO Current_time:5545
|
||||
2023-08-29 09:49:46,919 simple_logger.py log_once [line:28] INFO test_accuracy 0.8531
|
||||
2023-08-29 09:49:46,919 simple_logger.py log_once [line:28] INFO test_loss 0.6368
|
||||
2023-08-29 09:49:46,920 simple_logger.py log_once [line:28] INFO val_accuracy 0.8400
|
||||
2023-08-29 09:49:46,920 simple_logger.py log_once [line:28] INFO mean_val_accuracy 0.8400
|
||||
2023-08-29 09:49:46,920 simple_logger.py log_once [line:28] INFO std_val_accuracy 0.0508
|
||||
2023-08-29 09:49:46,920 simple_logger.py log_once [line:28] INFO val_loss 0.6688
|
||||
2023-08-29 09:49:46,920 simple_logger.py log_once [line:28] INFO mean_val_loss 0.6688
|
||||
2023-08-29 09:49:46,920 simple_logger.py log_once [line:28] INFO std_val_loss 0.0898
|
||||
2023-08-29 09:49:46,920 fedbase.py run [line:251] INFO Eval Time Cost: 1.6629s
|
||||
2023-08-29 09:49:48,299 con_fedavg.py iterate [line:50] INFO Receiving stale models from clients: []
|
||||
2023-08-29 09:49:48,299 con_fedavg.py iterate [line:51] INFO The staleness are []
|
||||
2023-08-29 09:49:48,299 con_fedavg.py iterate [line:52] INFO Averaging Staleness: nan
|
||||
**Cond 1**
|
||||
2023-08-29 09:50:17,109 fedbase.py run [line:246] INFO --------------Round 6--------------
|
||||
2023-08-29 09:50:17,109 simple_logger.py log_once [line:14] INFO Current_time:1206
|
||||
2023-08-29 09:50:18,785 simple_logger.py log_once [line:28] INFO test_accuracy 0.6874
|
||||
2023-08-29 09:50:18,785 simple_logger.py log_once [line:28] INFO test_loss 1.4880
|
||||
2023-08-29 09:50:18,785 simple_logger.py log_once [line:28] INFO val_accuracy 0.6745
|
||||
2023-08-29 09:50:18,785 simple_logger.py log_once [line:28] INFO mean_val_accuracy 0.6745
|
||||
2023-08-29 09:50:18,785 simple_logger.py log_once [line:28] INFO std_val_accuracy 0.0569
|
||||
2023-08-29 09:50:18,785 simple_logger.py log_once [line:28] INFO val_loss 1.5130
|
||||
2023-08-29 09:50:18,785 simple_logger.py log_once [line:28] INFO mean_val_loss 1.5130
|
||||
2023-08-29 09:50:18,785 simple_logger.py log_once [line:28] INFO std_val_loss 0.0650
|
||||
2023-08-29 09:50:18,785 fedbase.py run [line:251] INFO Eval Time Cost: 1.6764s
|
||||
2023-08-29 09:50:20,072 con_fedavg.py iterate [line:50] INFO Receiving stale models from clients: [12, 1, 16, 93, 15, 60, 25, 54, 68, 46, 23, 10, 30, 87, 64, 66, 0, 47, 73, 51, 62, 26]
|
||||
2023-08-29 09:50:20,072 con_fedavg.py iterate [line:51] INFO The staleness are [-1, -1, -2, -2, -2, -2, -4, -1, -2, -1, -1, -2, -3, -1, -1, -4, -4, -1, -4, -3, -1, -2]
|
||||
2023-08-29 09:50:20,072 con_fedavg.py iterate [line:52] INFO Averaging Staleness: -2.0454545454545454
|
||||
**Cond 2**
|
||||
2023-08-29 09:50:48,869 fedbase.py run [line:246] INFO --------------Round 6--------------
|
||||
2023-08-29 09:50:48,869 simple_logger.py log_once [line:14] INFO Current_time:1113
|
||||
2023-08-29 09:50:50,556 simple_logger.py log_once [line:28] INFO test_accuracy 0.7133
|
||||
2023-08-29 09:50:50,557 simple_logger.py log_once [line:28] INFO test_loss 1.5453
|
||||
2023-08-29 09:50:50,557 simple_logger.py log_once [line:28] INFO val_accuracy 0.6957
|
||||
2023-08-29 09:50:50,557 simple_logger.py log_once [line:28] INFO mean_val_accuracy 0.6957
|
||||
2023-08-29 09:50:50,557 simple_logger.py log_once [line:28] INFO std_val_accuracy 0.0558
|
||||
2023-08-29 09:50:50,557 simple_logger.py log_once [line:28] INFO val_loss 1.5653
|
||||
2023-08-29 09:50:50,557 simple_logger.py log_once [line:28] INFO mean_val_loss 1.5653
|
||||
2023-08-29 09:50:50,557 simple_logger.py log_once [line:28] INFO std_val_loss 0.0565
|
||||
2023-08-29 09:50:50,557 fedbase.py run [line:251] INFO Eval Time Cost: 1.6880s
|
||||
2023-08-29 09:50:51,886 con_fedavg.py iterate [line:50] INFO Receiving stale models from clients: [83, 12, 76, 84, 71, 46, 16]
|
||||
2023-08-29 09:50:51,886 con_fedavg.py iterate [line:51] INFO The staleness are [-4, -2, -4, -2, -3, -3, -4]
|
||||
2023-08-29 09:50:51,886 con_fedavg.py iterate [line:52] INFO Averaging Staleness: -3.142857142857143
|
||||
**Cond 0** costs the longest time to communication 6 rounds, but enjoys the highest aggregation efficiency (i.e. testing accuracy 85% v.s. others).
|
||||
Both **Cond 1** and **Cond 2** produce non-trivial staleness, but significantly reduce the time cost for communication (i.e. nearly 1/5 compared to Cond 0).
|
||||
|
||||
The results suggest that it's emergency to develop more aggregation efficient and less time-cost method in practical FL.
|
|
@ -0,0 +1 @@
|
|||
This section introduces how to customize each type of system heterogeneity in FLGo. Especially, different types of heterogeneity can be directly combined together in a Simulator to form a complex environment.
|
|
@ -0,0 +1,10 @@
|
|||
In this tutorial, we provide comprehensive examples to show how to use FLGo to help your researches.
|
||||
|
||||
Following our instructions, you can do things like
|
||||
|
||||
* easily reproduce and compare the results of different state-of-the-art methods
|
||||
* fast verify your ideas by converting them into runnable codes
|
||||
* conduct experiments under various data heterogeneiry and system heterogeneity
|
||||
* manage your experimental records and visualize them with few codes
|
||||
|
||||
All of our examples can be run on jupyter notebook. The source of our notebooks are [here](https://github.com/WwZzz/easyFL/tree/FLGo/tutorial/en).
|
|
@ -0,0 +1,5 @@
|
|||
# Contact us
|
||||
|
||||
[**Spatial Sensing and Computing Lab, Xiamen University**](http://asc.xmu.edu.cn/)
|
||||
|
||||
zwang@stu.xmu.edu.cn
|
|
@ -0,0 +1,62 @@
|
|||
## Install FLGo
|
||||
|
||||
Install FLGo through pip.
|
||||
|
||||
```
|
||||
pip install flgo
|
||||
```
|
||||
|
||||
If the package is not found, please use the command below to update pip
|
||||
|
||||
```
|
||||
pip install --upgrade pip
|
||||
```
|
||||
|
||||
## Create Your First Federated Task
|
||||
|
||||
Here we take the classical federated benchmark, Federated MNIST [1], as the example, where the MNIST dataset is splitted into 100 parts identically and independently.
|
||||
|
||||
```python
|
||||
import flgo
|
||||
import os
|
||||
|
||||
# the target path of the task
|
||||
task_path = './my_first_task'
|
||||
|
||||
# create task configuration
|
||||
task_config = {'benchmark':{'name': 'flgo.benchmark.mnist_classification'}, 'partitioner':{'name':'IIDPartitioner', 'para':{'num_clients':100}}}
|
||||
|
||||
# generate the task if the task doesn't exist
|
||||
if not os.path.exists(task_path):
|
||||
flgo.gen_task(task_config, task_path)
|
||||
```
|
||||
|
||||
After running the codes above, a federated dataset is successfully created in the `task_path`. The visualization of the task is stored in
|
||||
`task_path/res.png` as below
|
||||

|
||||
|
||||
|
||||
|
||||
## Run FedAvg to Train Your Model
|
||||
Now we are going to run the classical federated optimization algorithm, FedAvg [1], on the task created by us to train a model.
|
||||
```python
|
||||
import flgo.algorithm.fedavg as fedavg
|
||||
# create fedavg runner on the task
|
||||
runner = flgo.init(task, fedavg, {'gpu':[0,],'log_file':True, 'num_steps':5})
|
||||
runner.run()
|
||||
```
|
||||
|
||||
## Show Training Result
|
||||
The training result is saved as a record under the dictionary of the task `task_path/record`. We use the built-in analyzer to read and show it.
|
||||
```python
|
||||
import flgo.experiment.analyzer
|
||||
# create the analysis plan
|
||||
analysis_plan = {
|
||||
'Selector':{'task': task_path, 'header':['fedavg',], },
|
||||
'Painter':{'Curve':[{'args':{'x':'communication_round', 'y':'val_loss'}}]},
|
||||
'Table':{'min_value':[{'x':'val_loss'}]},
|
||||
}
|
||||
|
||||
flgo.experiment.analyzer.show(analysis_plan)
|
||||
```
|
||||

|
Binary file not shown.
After Width: | Height: | Size: 13 KiB |
Binary file not shown.
After Width: | Height: | Size: 66 KiB |
Binary file not shown.
After Width: | Height: | Size: 9.0 KiB |
Binary file not shown.
After Width: | Height: | Size: 7.9 KiB |
Some files were not shown because too many files have changed in this diff Show More
Loading…
Reference in New Issue